LGFDR: local and global feature denoising reconstruction for unsupervised anomaly detection
The Visual Computer(2024)
摘要
Unsupervised anomaly detection is a challenging task in many visual inspection scenarios and has attracted significant attention. Anomalies are typically related to local low-level features or require global semantic information to be detected. However, most of the existing methods fail to strike a balance between local and global features and thus lack versatility and practicality. To address this issue, we propose local and global feature denoising reconstruction (LGFDR). The proposed method can implicitly learn the latent distribution of local and global features for normal images via a dual-tower reconstruction network. Next, a selective reconstruction head (SRH) is designed to adaptively fuse the information from local and global reconstructions. Moreover, adding noise to the features proves a simple and general operation that can further enhance the generalization of reconstruction networks. On the MVTec AD benchmark, LGFDR achieves 98.8 https://github.com/Karma1628/work-1 .
更多查看译文
关键词
Unsupervised learning,Anomaly detection,Anomaly localization,Local and global anomalies,Feature denoising reconstruction
AI 理解论文
溯源树
样例
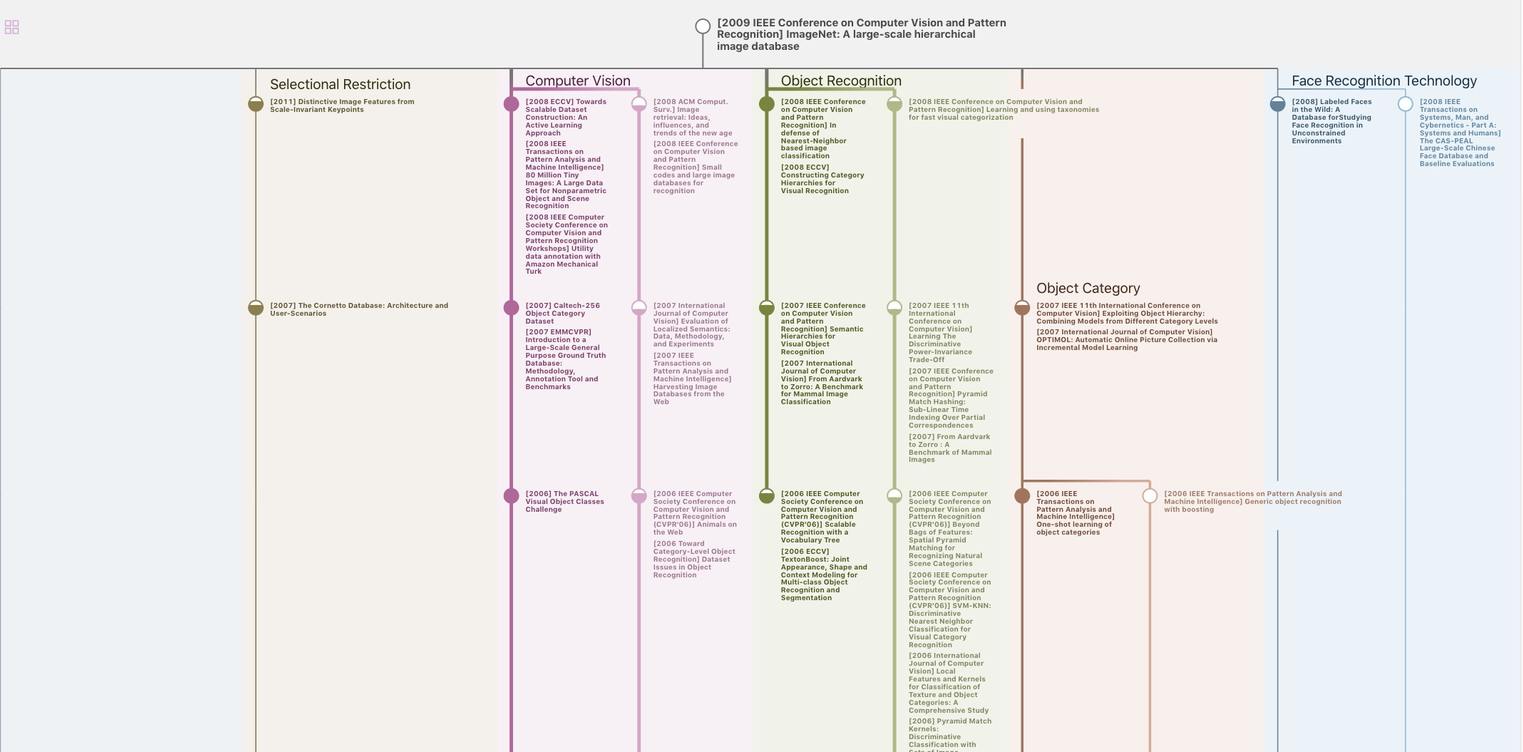
生成溯源树,研究论文发展脉络
Chat Paper
正在生成论文摘要