SCARNet: using convolution neural network to predict time series with time-varying variance
Multimedia Tools and Applications(2024)
摘要
Time series forecasting tasks are important in practical scenarios as they can be applied in various fields such as economics, meteorology, and transportation. However, there are still challenges when applying methods based on Convolutional Neural Networks (CNNs) and Recurrent Neural Networks (RNNs) to time series forecasting. These challenges include limitations in gradient propagation, handling long-range dependencies, and ensuring stability in the prediction results. In this paper, we propose a deep learning forecasting model called SCARNet (Stacked Convolution Sequence Autoregressive Encoding Network) to address these challenges. The SCARNet adopts an encoder-decoder structure and utilizes one-dimensional convolution to achieve autoregressive-like computations. This model can extract deeper-level information from time series, including trend components, periodic components, and white noise. Specifically, SCARNet employs a pyramid-stacked convolutional structure as the encoder for feature extraction and utilizes fully connected layers as the decoder for prediction. We evaluate the proposed model on a private and two mainstream public datasets. Experimental results demonstrate that the SCARNet model outperforms existing models in single-step prediction (with RMSE values of 0.0632, 0.6901, and 0.5416 for the three datasets, respectively) and achieves performance close to the state-of-the-art in medium-term and short-term multi-step prediction (Friedman Test at a significance level of 5
更多查看译文
关键词
Univariate time series,Pyramidal convolution,Deep forecasting,Stacked convolution sequence autoregressive encoding network (SCARNet)
AI 理解论文
溯源树
样例
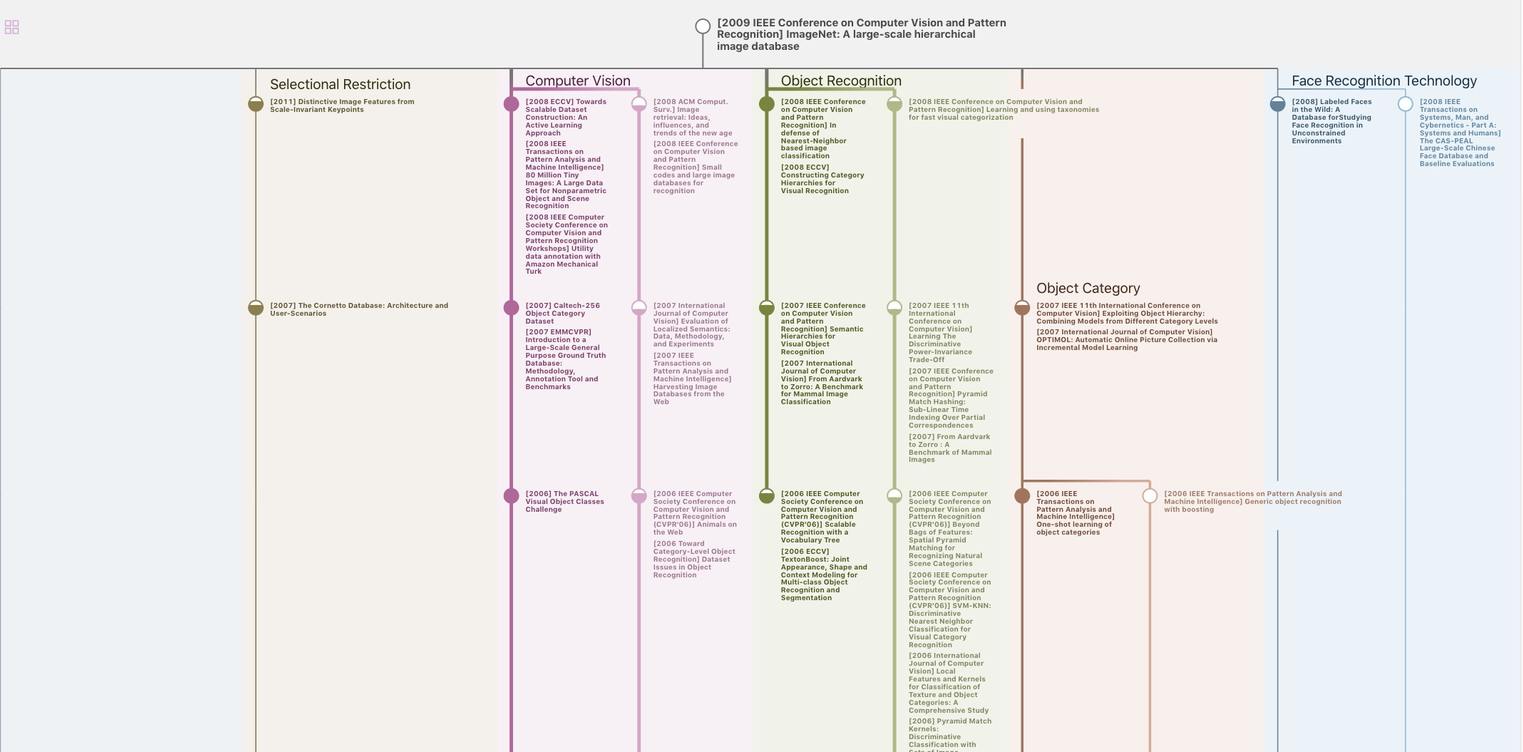
生成溯源树,研究论文发展脉络
Chat Paper
正在生成论文摘要