Bias Mitigation in Primary Healthcare Artificial Intelligence Models: Scoping Review (Preprint)
crossref(2024)
摘要
BACKGROUND Artificial intelligence (AI) predictive models in primary healthcare can potentially lead to benefits for population health. Algorithms can identify more rapidly and accurately who should receive care and health services, but they could also perpetuate or exacerbate existing biases toward diverse groups. We noticed a gap in actual knowledge about which strategies are deployed to assess and mitigate bias toward diverse groups, based on their personal or protected attributes, in primary healthcare algorithms. OBJECTIVE To describe attempts, strategies, and methods used to mitigate bias in primary healthcare artificial intelligence models. To identify which diverse groups or protected attributes have been considered. To evaluate the results on bias attenuation and AI models performance of these attempts, strategies, and methods. METHODS We conducted a scoping review informed by the Joanna Briggs Institute (JBI) review recommendations. An experienced librarian developed a search strategy in four databases (Medline (OVID), CINAHL (EBSCO), PsycInfo (OVID), and Web of Science) to identify sources published between 2017-01-01 and 2022-11-15. We imported data in Covidence and pairs of reviewers independently screened titles and abstracts, applied the selection criteria, and performed full-text screening. Any discrepancies regarding the inclusion of studies were resolved through consensus. Based on reporting standards for AI in health care, we performed data extraction - study objectives, models’ main features, diverse groups concerned, mitigation strategies deployed, and results. Using the Mixed-Methods Appraisal Tool (MMAT), we appraised the quality of studies. RESULTS After removing 585 duplicates, we screened 1018 titles and abstracts. From remaining 189 after exclusion, we excluded 172 full texts and included 17 studies. The most investigated personal or protected attributes were Race (or Ethnicity) in (12/17), and Sex (mostly identified as Gender in studies), using binary “male vs female” in (10/17) of included studies. We grouped studies according to bias mitigation attempts into the following categories: 1) existing AI models or datasets, 2) sourcing data such as Electronic Health Records, 3) developing tools with “human-in-the-loop” and 4) identifying ethical principles for informed decision-making. Mathematical and algorithmic preprocessing methods, such as changing data labeling and reweighing, along with a natural language processing method using data extraction from unstructured notes, showed the greatest potential. Other methods to enhance model fairness include group recalibration and the application of the equalized odds metric, which either exacerbated predictions errors between groups or resulted in overall models miscalibrations. CONCLUSIONS Results suggests that biases toward diverse groups can be more easily mitigated when data are open-sourced, multiple stakeholders are involved, and during the algorithm’ preprocessing stage. Further empirical studies, considering more diverse groups, such as nonbinary gender identities or Indigenous peoples in Canada, are needed to confirm and to expand this knowledge. CLINICALTRIAL OSF Registries qbph8; https://osf.io/qbph8 INTERNATIONAL REGISTERED REPORT RR2-10.2196/46684
更多查看译文
AI 理解论文
溯源树
样例
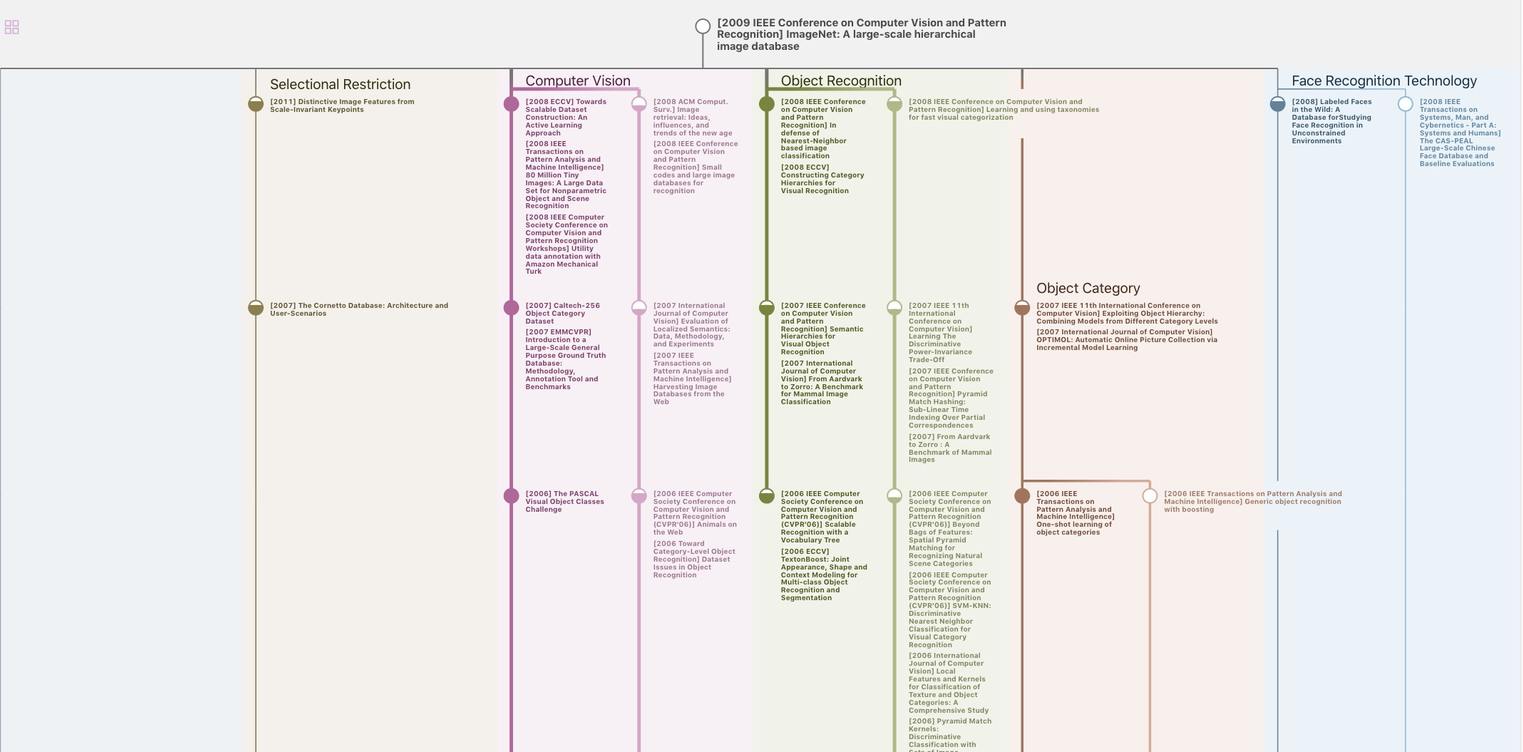
生成溯源树,研究论文发展脉络
Chat Paper
正在生成论文摘要