Fusion of Graph and Natural Language Processing in Predictive Analytics for Adverse Drug Reactions
2024 AUSTRALIAN COMPUTER SCIENCE WEEK, ACSW 2024(2024)
摘要
Adverse Drug Reactions (ADRs) pose a critical challenge to patient safety and healthcare economics worldwide. This study presents a novel graph-assisted machine learning algorithm applied to a comprehensive dataset provided by the Commonwealth Bank Health Society (CBHS), spanning 1976 to 2018, to predict ADRs. Utilizing the narrative-like structure of patients’ medical histories through Natural Language Processing (NLP), the research uniquely encodes International Classification of Diseases (ICD) codes into word embeddings. In a departure from traditional methods, it also employs networks analytics to transform disease histories into a knowledge graph structure that captures temporal and contextual relationships between ICD codes. This study's results demonstrated that integrating Node2Vec with Word2Vec improved model performance across various metrics, with significant enhancements in recall for K-nearest neighbors (KNN) and area under the receiver operating characteristic curve (AUROC) for all models. The findings underscore the potential of NLP in medical contexts and highlight the advantages of graph-based approaches in capturing complex, non-linear interdependencies inherent in patient data. This research marks a significant stride toward harnessing the power of NLP and graph theory in the predictive modeling of ADRs, aiming to improve patient outcomes by preempting potential adverse drug interactions.
更多查看译文
关键词
Knowledge Graph,Natural Language Processing,Adverse Drug Reactions,Machine Learning
AI 理解论文
溯源树
样例
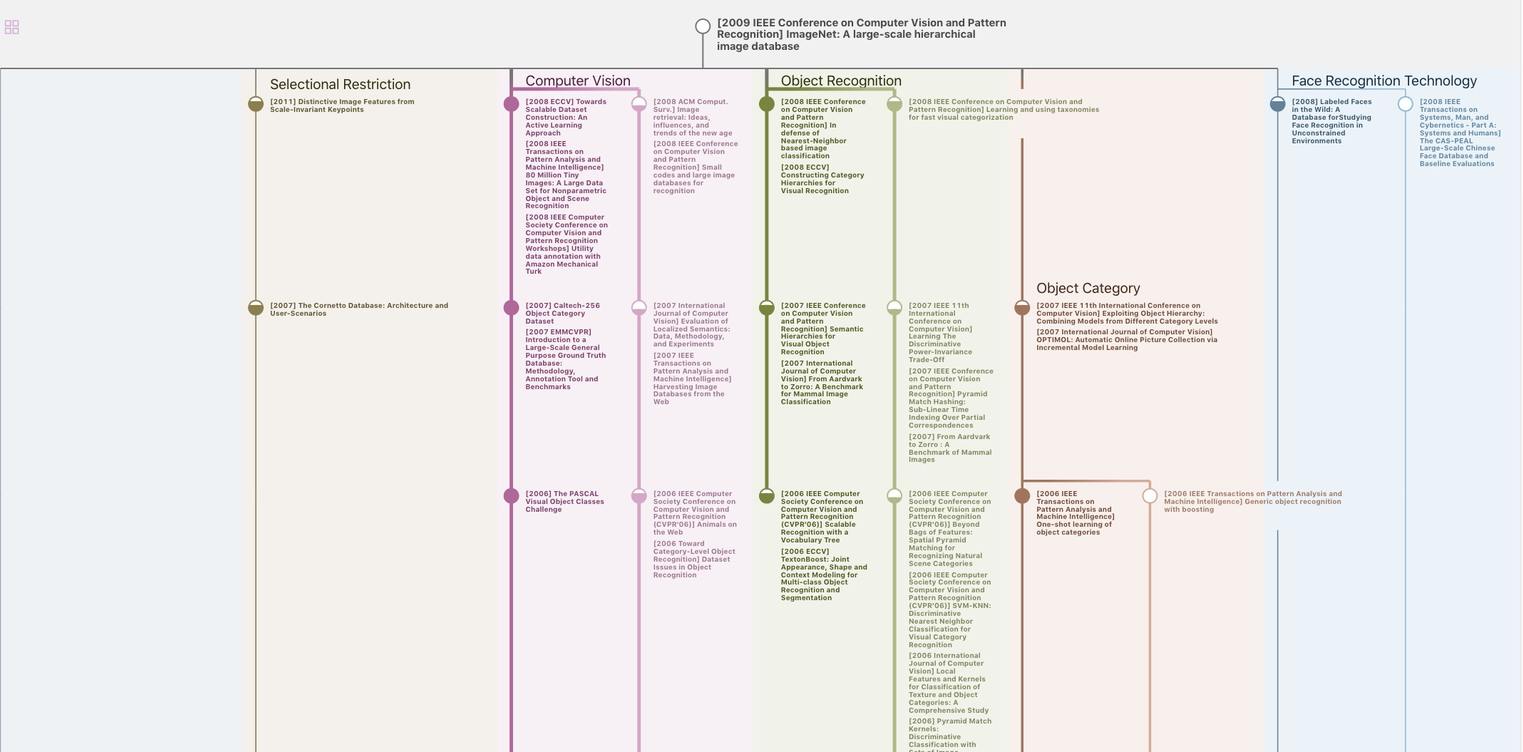
生成溯源树,研究论文发展脉络
Chat Paper
正在生成论文摘要