One-Dimensional Dictionary Learning with Variational Sparse Representation for Single-Channel Seismic Denoising
IEEE Transactions on Geoscience and Remote Sensing(2024)
摘要
Seismic data acquired from the field inevitably suffer from noise pollution, which covers the useful signals and affects the reliability of subsequent seismic data processing and interpretation. Many two-dimensional (2D) multi-channel seismic denoising methods depend on the assumption that the receiver array is spatial coherent in field microseismic data acquisition, which limits their performance when dealing with field data. However, the single-channel methods are more flexible when faced with real microseismic data because they do not require any assumptions regarding spatial coherency. Therefore, we propose a one-dimensional (1D) dictionary learning (DL) framework based on variational sparse representation to suppress background noise in seismic data. Compared with the 2D multi-channel denoising method, the proposed method takes into account the waveform characteristics and requires no spatial coherency of single-channel seismic data, thus achieving better denoising performance. Additionally, the 1D dictionary learning method requires fewer training samples than the 2D method to reach a promising result, thereby causing less consumption time. Numerical results show that compared with the bandpass (BP) filtering, structure-oriented filtering (SOF), and K-SVD methods, the proposed DL framework can significantly improve the signal-to-noise ratio (SNR) of seismic data and protect effective signals better without causing extra computation. Furthermore, we discuss how to further suppress the residual horizontal noise and erratic noise based on the proposed method.
更多查看译文
关键词
one-dimensional seismic data,K-SVD,dictionary learning,variational sparse representation,seismic data denoising
AI 理解论文
溯源树
样例
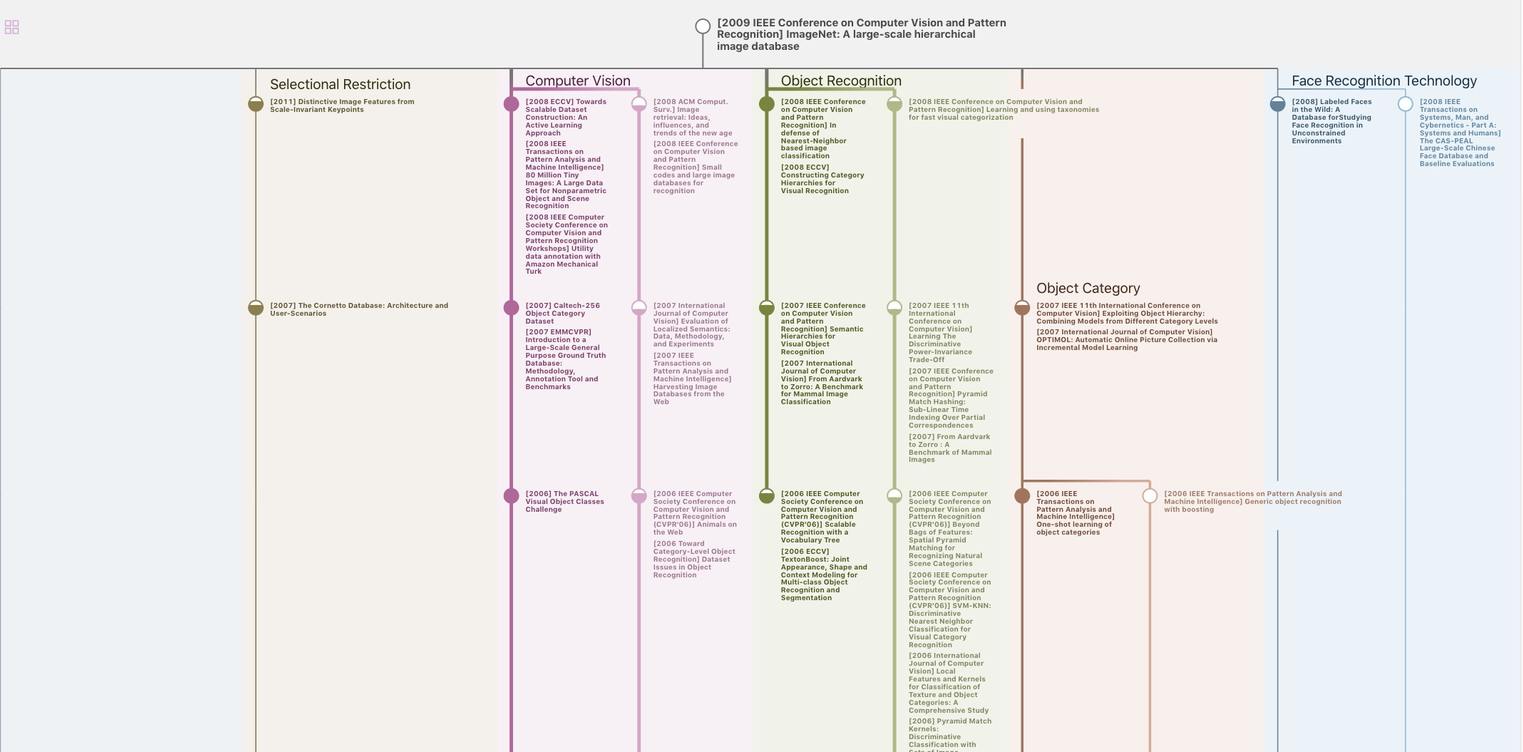
生成溯源树,研究论文发展脉络
Chat Paper
正在生成论文摘要