Comparison of machine learning algorithms for estimating global lake clarity with Landsat TOA data
IEEE Transactions on Geoscience and Remote Sensing(2024)
摘要
The Secchi disk depth (SDD) is a measure of water clarity appropriate to assess a lake’s trophic level. Optical satellite remote sensing has been widely used to detect water SDD at regional, continental, or global scales, with the Google Earth Engine (GEE) providing an excellent platform for fast and large-scale monitoring of SDD for global lakes. Hence, this study evaluates whether retrieving SDD for global lakes is possible by applying machine learning algorithms using Landsat top-of-atmosphere reflectance (TOA) embedded in GEE. Eleven algorithms were calibrated (5498 samples), and their performances were validated (2749 samples) with a global dataset of Landsat TOA reflectance and in situ SDD match-ups. The results indicated that the smallest modeling error was derived from Gradient Boosting Decision Tree (GBDT) (
R
2
= 0.98,
RMSE
= 0.27 m,
MAPE
= 10.52%,
MAE
= 0.07 m), followed by XGBoost (XGB) and Swarm Optimization-Random Forest (PSO-RF). Furthermore, it was demonstrated that combining GBDT, XGB, PSO-RF, and Landsat TOA reflectance provides a robust way to monitor SDD across global lakes. Eventually, global SDD maps for 2020 were produced using Landsat OLI TOA imagery, with SDD changing range from 0.01 to 13.1 m (mean = 3.3 m). The machine learning models in this study can be applied to archived Landsat imagery to assess SDD trends of global lakes and capture annual or seasonal variations.
更多查看译文
关键词
Secchi disk depth,top-of-atmosphere,machine learning algorithm
AI 理解论文
溯源树
样例
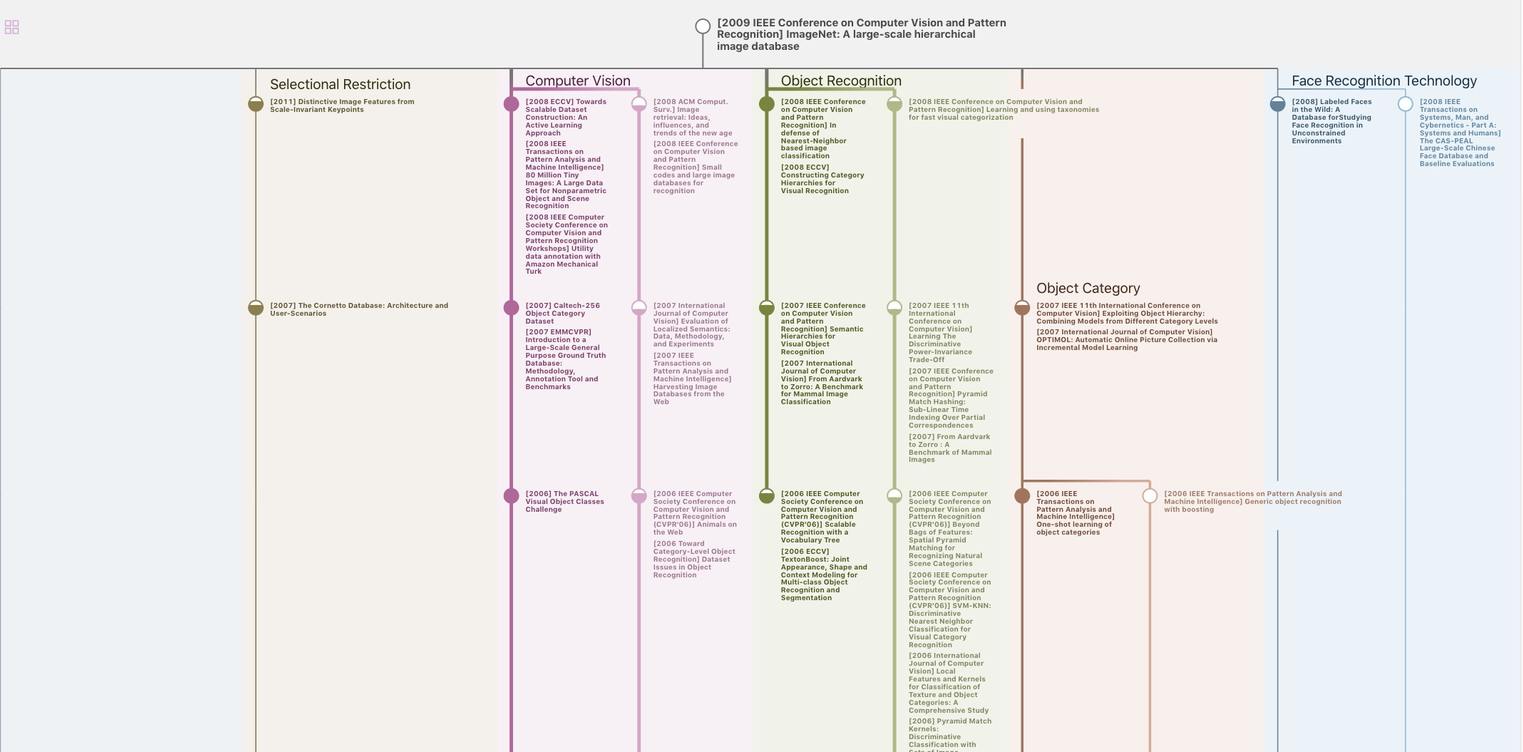
生成溯源树,研究论文发展脉络
Chat Paper
正在生成论文摘要