SIAlex: Species Identification and Monitoring Based on Bird Sound Features
Ecological informatics(2024)
摘要
The combination of deep learning and bird sound recognition is widely employed in bird species conservation monitoring. A complex network structure is not conducive for deploying bird sound recognition devices, resulting in problems such as long inference time and low efficiency. Using AlexNet as the backbone model, we explore the potential of shallow and straightforward models without complex connection techniques or attention mechanisms, named SIAlex, to recognise and classify 20 bird sound datasets, which are simultaneously validated on a 10 class UrbanSound8k dataset. Using the structural re-parameterization method, the number of model layers is reduced, computational efficiency is improved, and the inference time is significantly reduced, achieving a decoupling of training and inference time in the structure. To increase the nonlinearity of the model, a cascaded approach is utilised to increase the number of activation functions, thereby significantly improving the generalisation performance of the model. Simultaneously, in the classifier section, convolutional layer replaces the original fully connected layer, thereby reducing the inference time and increasing the feature extraction ability of the model, improving accuracy, and effectively recognising bird speech. The experimental data show that the SIAlex network on the Birdsdata dataset improves the accuracy to 93.66%, and the inference time for a piece of data is only 2.466 ms. The accuracy of the UrbanSound8k dataset reaches 96.04%, and the inference time for a piece of data is 3.031 ms. A large number of experimental comparisons have shown that the method proposed in this paper achieves good results in reducing the inference time of the model, bringing breakthroughs in the application of shallow, simple models.
更多查看译文
关键词
Lightweight,Cascading activation function,Bird sound recognition,Structural re -parameterization,Nonlinear performance
AI 理解论文
溯源树
样例
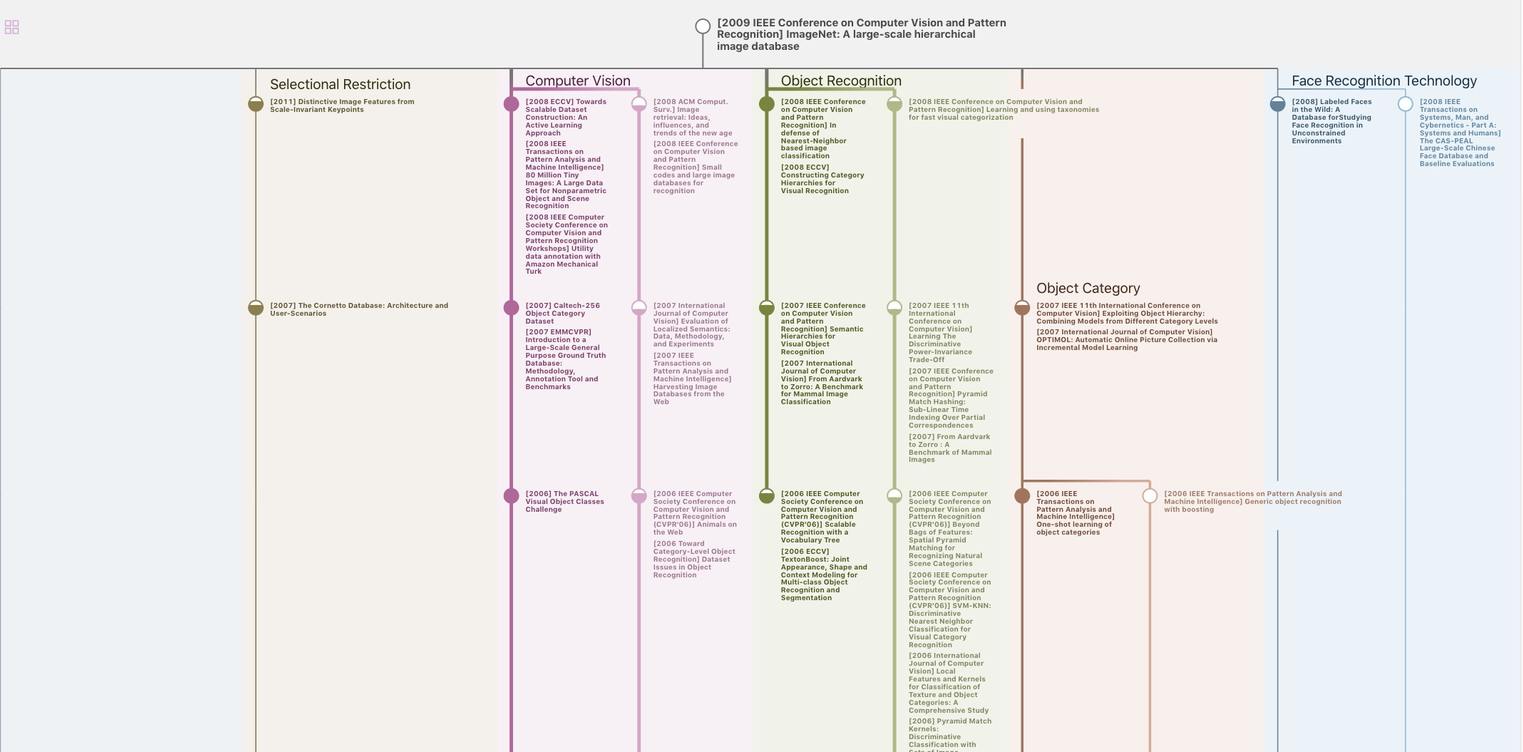
生成溯源树,研究论文发展脉络
Chat Paper
正在生成论文摘要