Improved Bound for Robust Causal Bandits with Linear Models
International Symposium on Information Theory(2024)
Abstract
This paper investigates the robustness of causal bandits (CBs) in the face oftemporal model fluctuations. This setting deviates from the existingliterature's widely-adopted assumption of constant causal models. The focus ison causal systems with linear structural equation models (SEMs). The SEMs andthe time-varying pre- and post-interventional statistical models are allunknown and subject to variations over time. The goal is to design a sequenceof interventions that incur the smallest cumulative regret compared to anoracle aware of the entire causal model and its fluctuations. A robust CBalgorithm is proposed, and its cumulative regret is analyzed by establishingboth upper and lower bounds on the regret. It is shown that in a graph withmaximum in-degree d, length of the largest causal path L, and an aggregatemodel deviation C, the regret is upper bounded by𝒪̃(d^L-1/2(√(T) + C)) and lower bounded byΩ(d^L/2-2max{√(T) , d^2C}). The proposed algorithmachieves nearly optimal 𝒪̃(√(T)) regret when C iso(√(T)), maintaining sub-linear regret for a broad range of C.
MoreTranslated text
Key words
Linear Model,Lower Bound,Upper Bound,Structural Equation Modeling,Causal Model,Causal Path,Causal System,Absolute Difference,Weight Matrix,Ordinary Least Squares,Column Vector,Treatment Model,Graphical Model,Graph Structure,Model Misspecification,Directed Acyclic Graph,Ordinary Least Squares Estimates,Outlier Samples,Linear Graph,Causal Structure,Upper Confidence Bound,Multi-armed Bandit,Bandit Problem,Gram Matrix,Causal Graph,Reproducing Kernel Hilbert Space,Nominal Model,Nodes In The Graph,Euclidean Norm
PDF
View via Publisher
AI Read Science
AI Summary
AI Summary is the key point extracted automatically understanding the full text of the paper, including the background, methods, results, conclusions, icons and other key content, so that you can get the outline of the paper at a glance.
Example
Background
Key content
Introduction
Methods
Results
Related work
Fund
Key content
- Pretraining has recently greatly promoted the development of natural language processing (NLP)
- We show that M6 outperforms the baselines in multimodal downstream tasks, and the large M6 with 10 parameters can reach a better performance
- We propose a method called M6 that is able to process information of multiple modalities and perform both single-modal and cross-modal understanding and generation
- The model is scaled to large model with 10 billion parameters with sophisticated deployment, and the 10 -parameter M6-large is the largest pretrained model in Chinese
- Experimental results show that our proposed M6 outperforms the baseline in a number of downstream tasks concerning both single modality and multiple modalities We will continue the pretraining of extremely large models by increasing data to explore the limit of its performance
Try using models to generate summary,it takes about 60s
Must-Reading Tree
Example
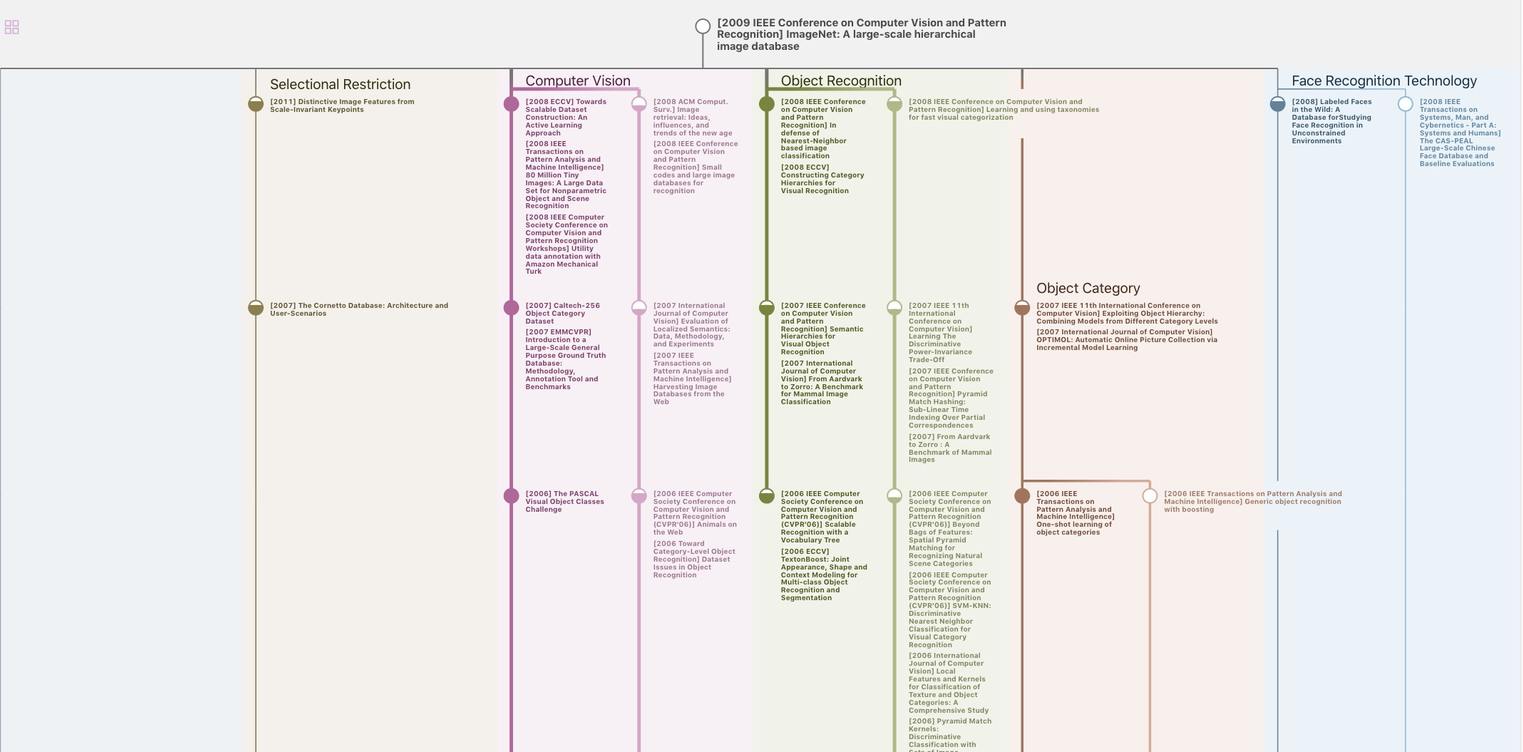
Generate MRT to find the research sequence of this paper
Related Papers
Data Disclaimer
The page data are from open Internet sources, cooperative publishers and automatic analysis results through AI technology. We do not make any commitments and guarantees for the validity, accuracy, correctness, reliability, completeness and timeliness of the page data. If you have any questions, please contact us by email: report@aminer.cn
Chat Paper
GPU is busy, summary generation fails
Rerequest