Optimization Using Pathwise Algorithmic Derivatives of Electromagnetic Shower Simulations
arxiv(2024)
摘要
Among the well-known methods to approximate derivatives of expectancies
computed by Monte-Carlo simulations, averages of pathwise derivatives are often
the easiest one to apply. Computing them via algorithmic differentiation
typically does not require major manual analysis and rewriting of the code,
even for very complex programs like simulations of particle-detector
interactions in high-energy physics. However, the pathwise derivative estimator
can be biased if there are discontinuities in the program, which may diminish
its value for applications.
This work integrates algorithmic differentiation into the electromagnetic
shower simulation code HepEmShow based on G4HepEm, allowing us to study how
well pathwise derivatives approximate derivatives of energy depositions in a
sampling calorimeter with respect to parameters of the beam and geometry. We
found that when multiple scattering is disabled in the simulation, means of
pathwise derivatives converge quickly to their expected values, and these are
close to the actual derivatives of the energy deposition. Additionally, we
demonstrate the applicability of this novel gradient estimator for stochastic
gradient-based optimization in a model example.
更多查看译文
AI 理解论文
溯源树
样例
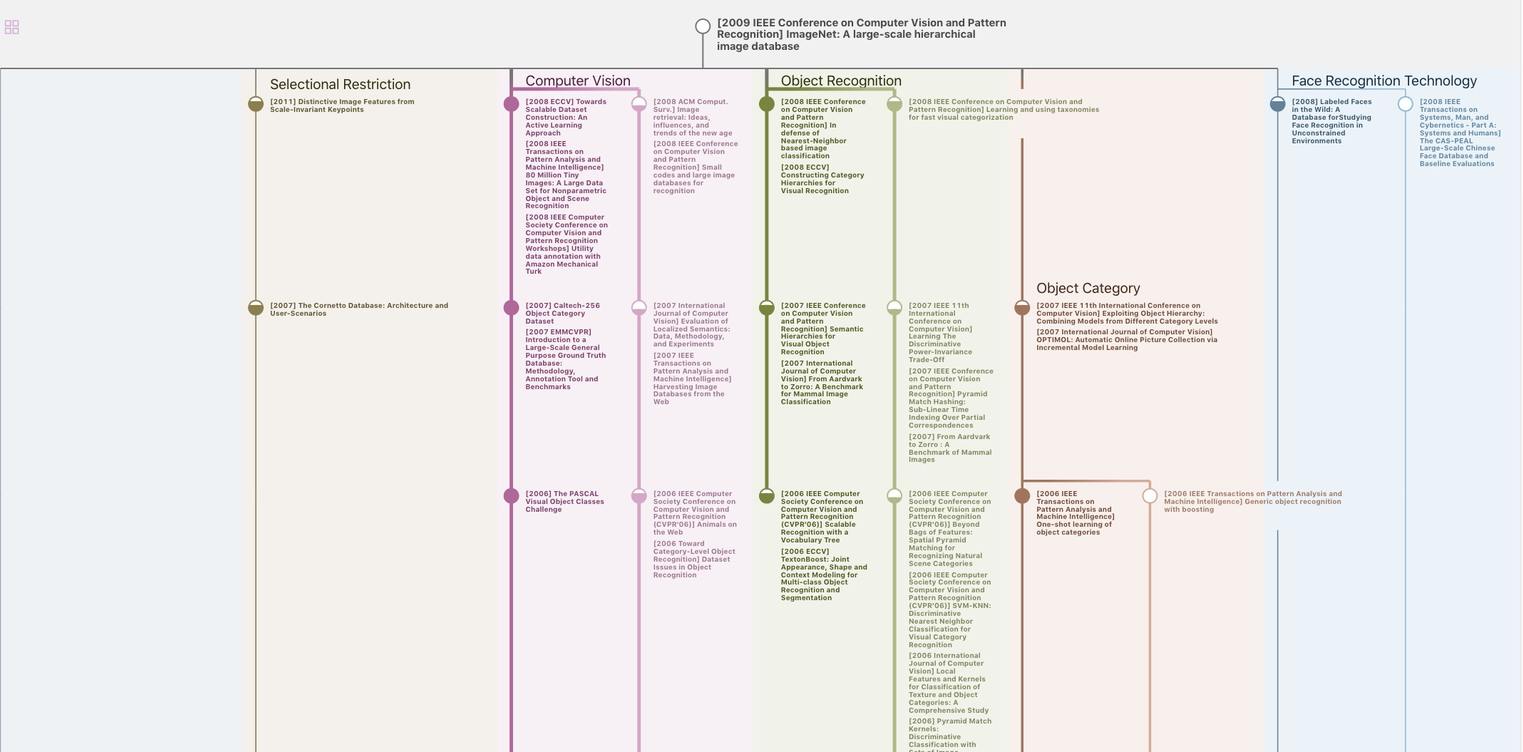
生成溯源树,研究论文发展脉络
Chat Paper
正在生成论文摘要