WSM-MIL: a weakly supervised segmentation method with multiple instance learning for C elegans image
PHYSICA SCRIPTA(2024)
摘要
Recently, image analysis techniques have been introduced to automate nematode information assessment. In image analysis-based nematode information assessment, the initial step involves detecting and segmenting C. elegans from microscopic images and network-based methods have been investigated. However, training a network for C. elegans image segmentation is typically associated with the labor-intensive process of pixel-level mask labeling. To address this challenge, we introduced a weakly supervised segmentation method using multiple instance learning (WSM-MIL). The proposed multi-instance weakly supervised segmentation method comprises three key components: a backbone network, a detection branch, and a segmentation branch. In contrast to fully supervised pixel-level annotation, we opted for weakly supervised bounding box-level annotation. This approach reduces the labour cost of annotation to some extent. The approach offers several advantages, such as simplicity, an end-to-end architecture, and good scalability. We conducted experiments comparing the proposed network with benchmark methods, and the results showed that the network exhibits competitive performance in the image segmentation task of C. elegans. The results of this study provide an effective method in the field of biological image analysis, as well as new ideas for solving complex segmentation tasks. The method is not only applicable to the study of C. elegans but also has wide applicability in biological image segmentation problems in other fields.
更多查看译文
关键词
caenorhabditis elegans,image segmentation,bounding-box level annotations,multi-instance learning,weakly supervised learning,biological image analysis
AI 理解论文
溯源树
样例
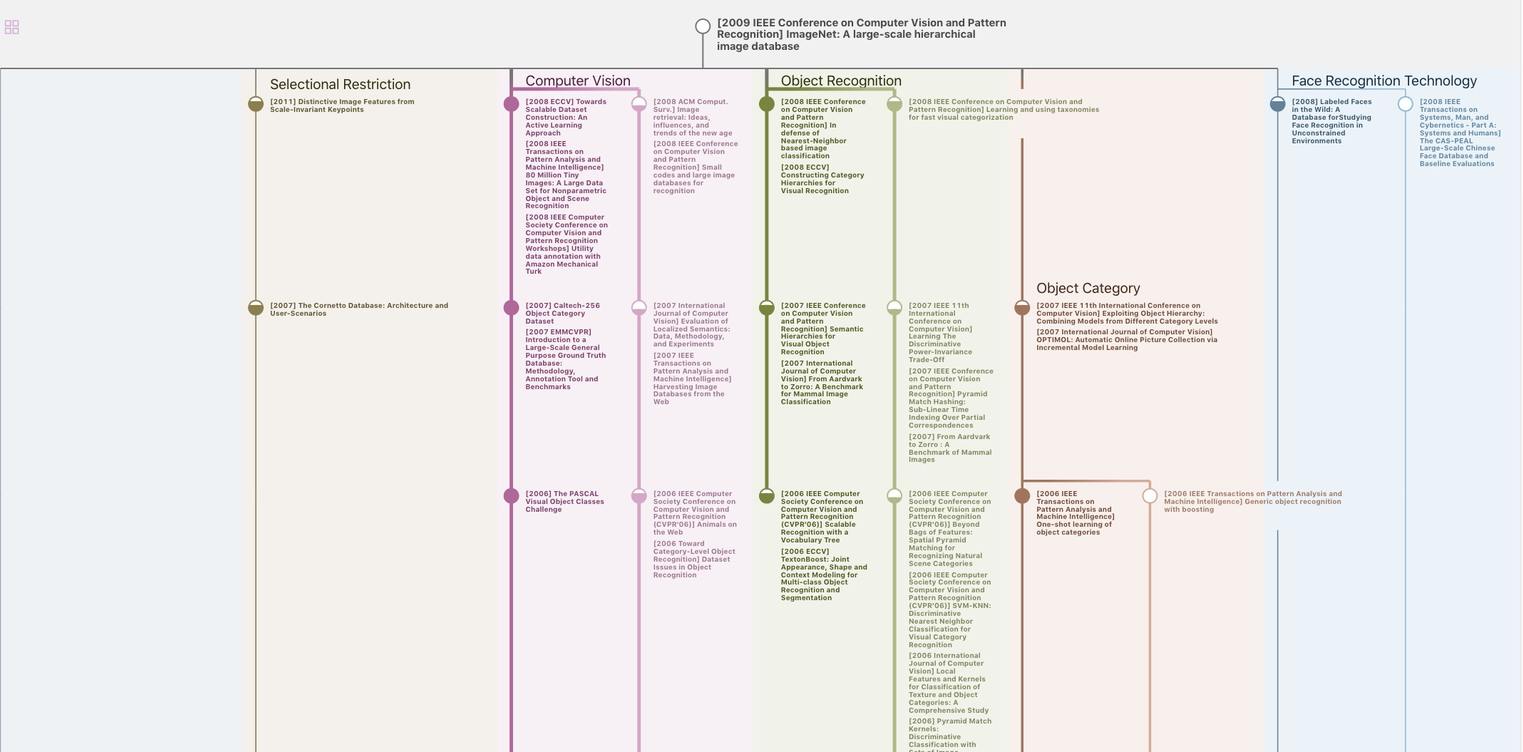
生成溯源树,研究论文发展脉络
Chat Paper
正在生成论文摘要