Coronary artery segmentation based on ACMA-Net and unscented Kalman filter algorithm
Computers in Biology and Medicine(2024)
摘要
Accurate segmentation of coronary arteries is a prerequisite for high-precision reconstruction of three-dimensional (3D) models of coronary arteries. With personalized 3D coronary anatomy, clinicians can more readily visually determine the location, type, and severity of a stenotic lesion. However, due to the complex structure and small size of coronary arteries, and the interference of image acquisition conditions, 3D models of segmented and reconstructed coronary arteries are noisy and prone to disconnection; accurate segmentation of coronary arteries is still a challenge.To overcome the above challenges, this study proposes a two-stage coronary segmentation algorithm. In the first stage, we added an atrous convolution feature fusion module (ACFFM) and a multi-axis attention module (MAM) to 3D U-Net, referred to as ACMA-Net, to enhance the feature expression ability of the network and effect the preliminary segmentation of coronary arteries. In the second stage, the preliminary segmentation results were skeletonized and endpoint detection was performed. The regions of coronary artery disconnection were determined by finding the minimum distance between the main trunk branch and the endpoint of each disconnected branch. The disconnected skeleton was repaired by reconnection using an unscented Kalman filter (UKF) algorithm.On the private CORO dataset and the public ASOCA dataset, our method obtains an average Dice coefficient of 0.818 and 0.731, respectively, so it outperforms most of the state-of-the-art algorithms compared, proving its excellent effectiveness and robustness.
更多查看译文
关键词
Coronary artery segmentation,Attention mechanism,Atrous convolution,Deep learning,Unscented Kalman filter
AI 理解论文
溯源树
样例
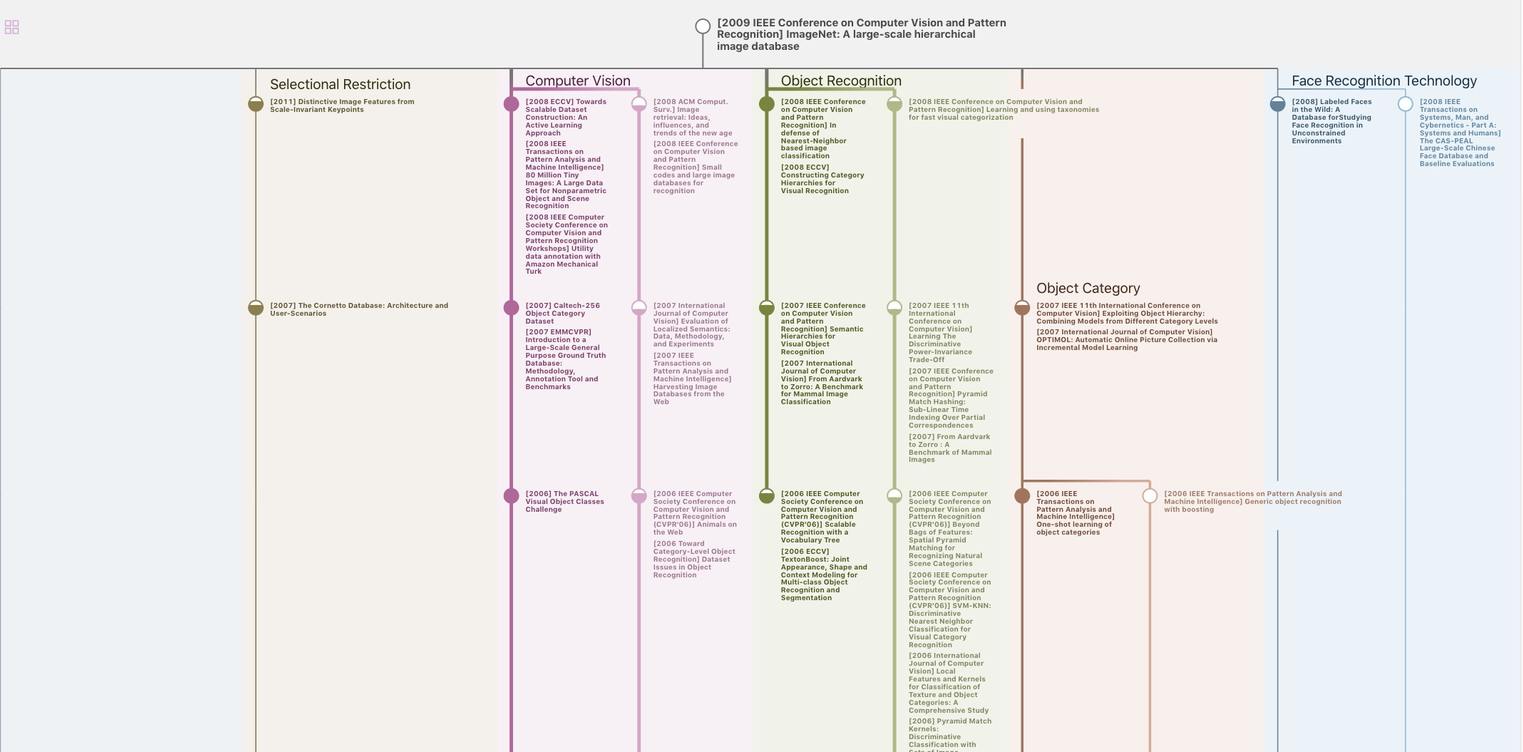
生成溯源树,研究论文发展脉络
Chat Paper
正在生成论文摘要