A framework for detecting zero-day exploits in network flows
Computer Networks(2024)
摘要
Zero-day attack detection solutions aim to proactively identify unknown threats targeting valuable assets within a given system. While many Intrusion Detection System (IDS) solutions leverage learning techniques to build novel attack detection systems, they often focus on enhancing accuracy for specific attack types, overlooking the potential for multiple attack scenarios. Therefore, we introduce a novel framework for detecting zero-day attacks that evade current detection systems. Our framework enhances attack identification and qualification through a hybrid learning approach, where supervised learning ensures detection of known attacks and unsupervised learning. It encompasses intrusion detection phases from data collection to new attack class detection by identifying anomalies in real-time network flow data. Unsupervised learning, which involves grouping similar data points into clusters, establishes minimum distances within these clusters. This process triggers cluster division when certain thresholds are reached. Finally, an online supervised learning process validates our approach’s effectiveness in identifying anomalies associated with zero-day attack flows.This approach significantly reduces the False Detection Rate (FDR) without solely focusing on optimizing machine learning (ML) and deep learning (DL) algorithms hyper-parameters. We evaluated our framework on two datasets: one from a real industrial context at IBM and the NSL-KDD dataset. The results demonstrate our framework’s ability to detect anomalies in previously zero-day attack targets. On average, we identified 71 anomalous flows per target, achieving an overall average online learning accuracy of 98.4% for the IBM dataset and 96.6% for the NSL-KDD dataset, thereby validating the detection of these new attack scenarios.
更多查看译文
关键词
Zero-day,Intrusion detection system,Machine learning,Deep learning,Cyber attack
AI 理解论文
溯源树
样例
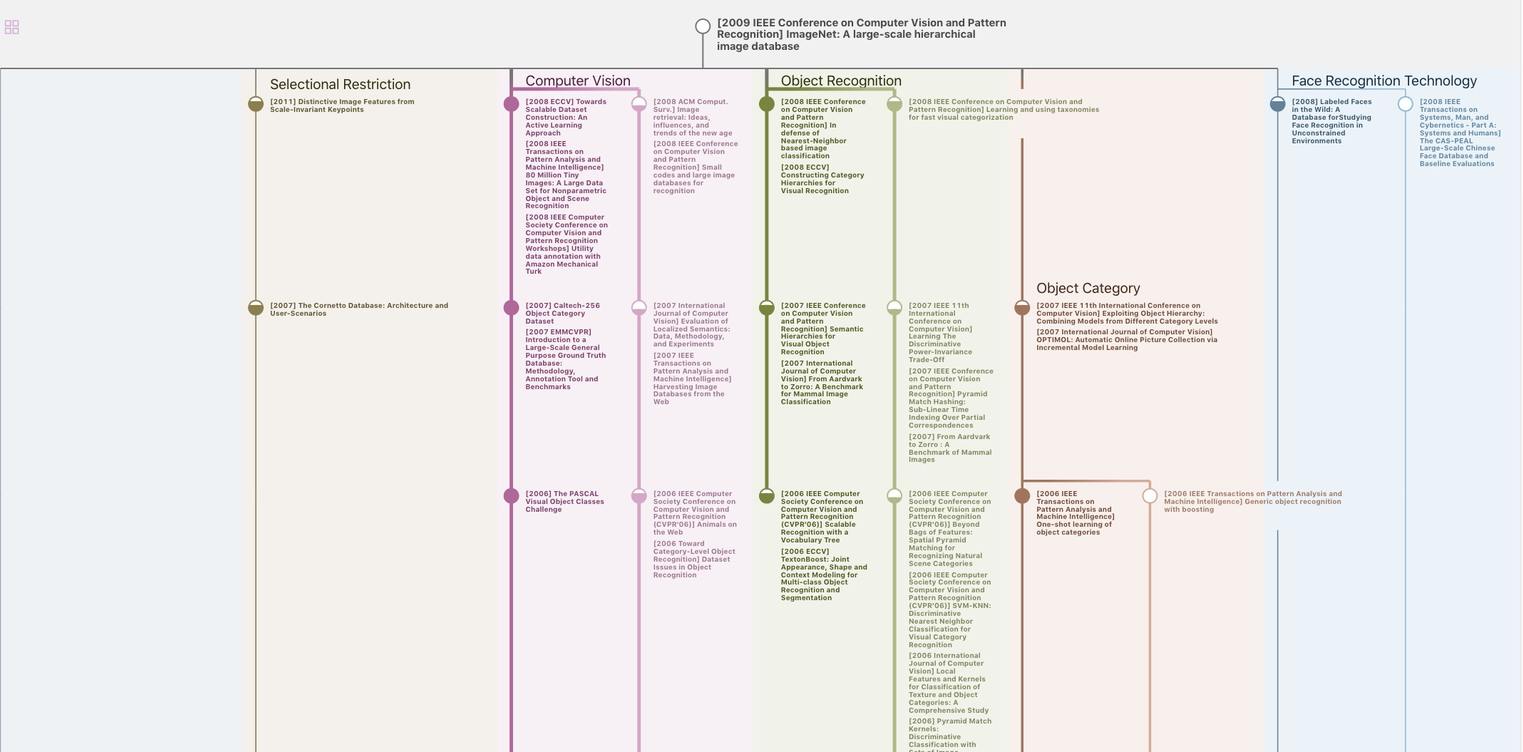
生成溯源树,研究论文发展脉络
Chat Paper
正在生成论文摘要