Magnetic Resonance Sounding Data Denoising based on Successive Dn-ResUnet Models with Noise Pre-Detection Using Support Vector Machine
IEEE Transactions on Geoscience and Remote Sensing(2024)
摘要
Magnetic resonance sounding (MRS) measurements used for detecting the subsurface aquifers commonly suffer from the notoriously low signal-to-noise ratio (SNR). The conventional approaches usually deal with the specific noise components step by step to improve the SNR, but the denoising effect is limited. MRS denoising methods based on neural networks show great potential in recovering the effective signals at low SNRs, but need large amount of high-quality labeled training datasets and have limitations in the application of measured data. And the denoising model trained for specific noise without noise type identification leads to excessive noise cancellation and low effective signal fidelity. To address these issues, we propose an intelligent denoising process by combining the noise detection method using support vector machine (SVM) and the intelligent denoising models for suppressing the specific noise. We first consider the discriminative features from the noisy signal in time and frequency domains for specific types of noise to be identified, so as to construct 3D feature vectors. Second, the noise detection model using SVM with radial basis function (RBF) kernel function is trained on the training samples. And the cross-validation technique is adapted to assess the performance of the noise detectors in the training process. Finally, the combination of the well-trained SVM noise detectors and the Dn-ResUnet models for specific noise is applied to process the noisy MRS data. The results of the simulation and field experiments show that our proposed method provides a one-stop flow for automatic noise identification and intelligent noise removal, which achieves a better denoising performance compared with the existing denoising methods.
更多查看译文
关键词
Magnetic resonance sounding (MRS),signal denoising,noise detection,Support Vector Machine
AI 理解论文
溯源树
样例
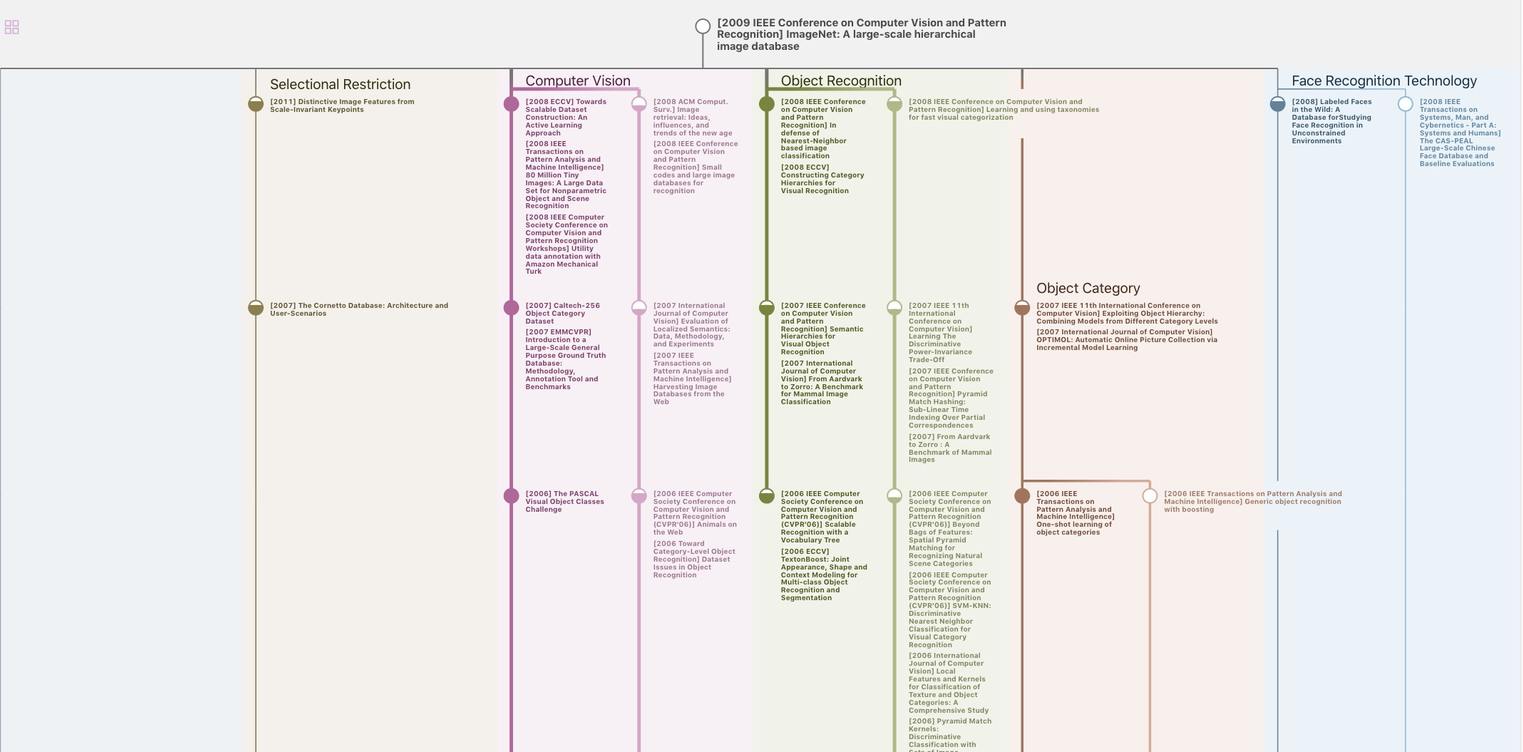
生成溯源树,研究论文发展脉络
Chat Paper
正在生成论文摘要