Mitigating Stochasticity in Visual Servoing of Soft Robots Using Data-Driven Generative Models
International Conference on Soft Robotics(2024)
摘要
Soft robots, due to their adaptability and safety, can make a significant impact in specialized fields such as surgical robotics owing to their compliance. However, such highly flexible robots are especially susceptible to modeling and control challenges due to nonlinearities, large deformations, and infinite degrees of freedom. Combined with material variations, manufacturing inconsistencies, and environmental influences, these robots exhibit stochastic behavior. To address this, the paper introduces a learning-based approach supported by a Neural Network-based Generative Model designed to address the inherent stochastic nature of such robots in visual servoing tasks. By generating large quantities of synthetic data that represent the stochastic effects, another learning model used for control can be trained to mitigate them. The method's efficacy is demonstrated in visual servoing tasks by significantly improving the accuracy of the robot. Implementing the proposed model in a feedback control loop improves the performance when compared to the open loop approach in more challenging trajectories, marking a substantial stride in overcoming the challenges associated with stochastic behavior in soft robotics.
更多查看译文
关键词
Soft Robots,Visual Servoing,Learning Models,Feedback Control,Large Deformation,Open Loop,Inherent Noise,Neural Network,Model Performance,Actuator,Active Learning,Adam Optimizer,Lookup Table,Kriging,Inverse Model,Incremental Learning,Robot Control,ReLU Activation Function,Camera View,Template Matching,Open-loop Control,Task Space,Inverse Mapping,Reference Template,Gaussian Process Regression Model,Forward Mapping,Motion Estimation,Kinematic,Validation Set,Robot Performance
AI 理解论文
溯源树
样例
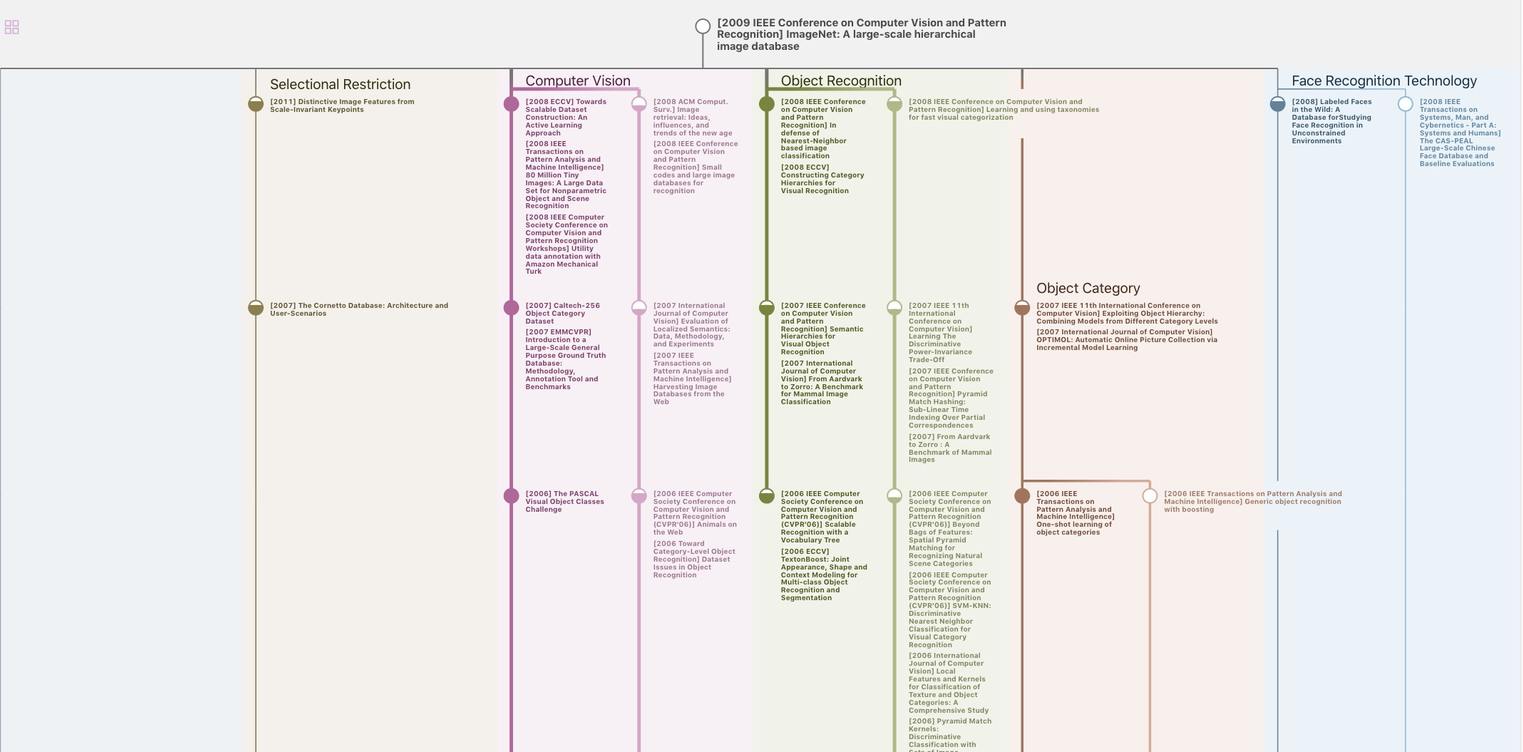
生成溯源树,研究论文发展脉络
Chat Paper
正在生成论文摘要