Global meat consumption driver analysis with machine learning methods
Food Security(2024)
摘要
The growing global meat consumption has serious consequences on human health, the environment and ultimately impacts global food security. Therefore, identifying the drivers of meat consumption and predicting its evolution is necessary. We compared four machine learning methods in modelling meat consumption, leading to the selection of a random forest-based model to detect main drivers for global meat consumption. Our results show that per capita meat consumption is mainly driven by socioeconomic factors, such as national GDP and urbanization. However, the strength of these drivers declined between 1990 and 2018. Pork, beef, and poultry consumption are mainly driven by socioeconomic factors, whereas mutton consumption appears driven by other factors such as the per capita agricultural land. In this work, the model-agnostic interpretability method is introduced to measure the marginal effect of each driver on meat consumption. We found that there may be insufficient evidence to support the inverted U-shaped relationship between per capita GDP and meat consumption, which is reported in previous studies. Our analysis may provide avenues for predicting meat consumption at the national scale.
更多查看译文
关键词
Meat consumption,Driver analysis,Interpretable machine learning,National-level prediction
AI 理解论文
溯源树
样例
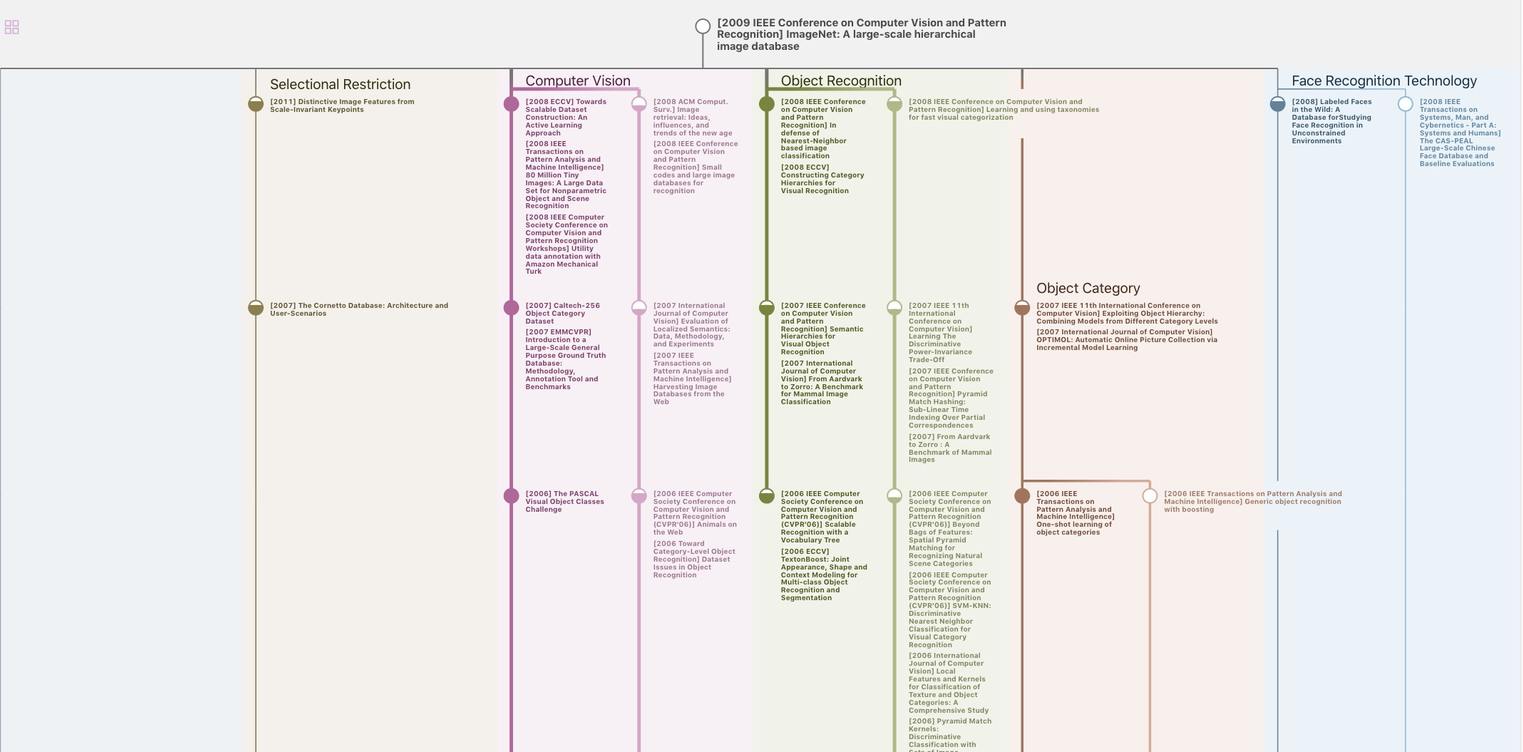
生成溯源树,研究论文发展脉络
Chat Paper
正在生成论文摘要