Prediction of Impact Sensitivity and Electrostatic Spark Sensitivity for Energetic Compounds by Machine Learning and Density Functional Theory
Journal of materials science(2024)
Abstract
In the present work, four machine learning algorithms back propagation neural network, multilayer perceptron, random forest and support vector regression were employed to predict both the impact sensitivity and electrostatic spark sensitivity of energetic compounds. Based on the density functional theory calculations mainly, there are nine and ten different kinds of descriptors which contain the important parameters related to the molecular composition and structure, the electronic structure, the structural stability and strength, and the energy performance have been selected out to construct the ML models for predicting the impact sensitivity and electrostatic spark sensitivity, respectively. It could be found that selected descriptors are well correlated with the experimental results which have high Pearson’s correlation coefficients generally. For the impact sensitivity, the oxygen balance, charge of nitro group, heat of release and self-multiplying coefficient are key descriptors which should be with larger weightiness in the construction of the ML model, while those are the detonation velocity and detonation pressure in the ML of electrostatic spark sensitivity. Based on the analysis and comparing of various parameters for the assessment of ML models, for both the impact sensitivity and electrostatic spark sensitivity, the back propagation neural network model was found to be possess high accuracy and better than other three used models. This work may provide new methods for predicting the sensitivity of energetic compounds and promote the application of machine learning in the field of energetic compounds.
MoreTranslated text
AI Read Science
Must-Reading Tree
Example
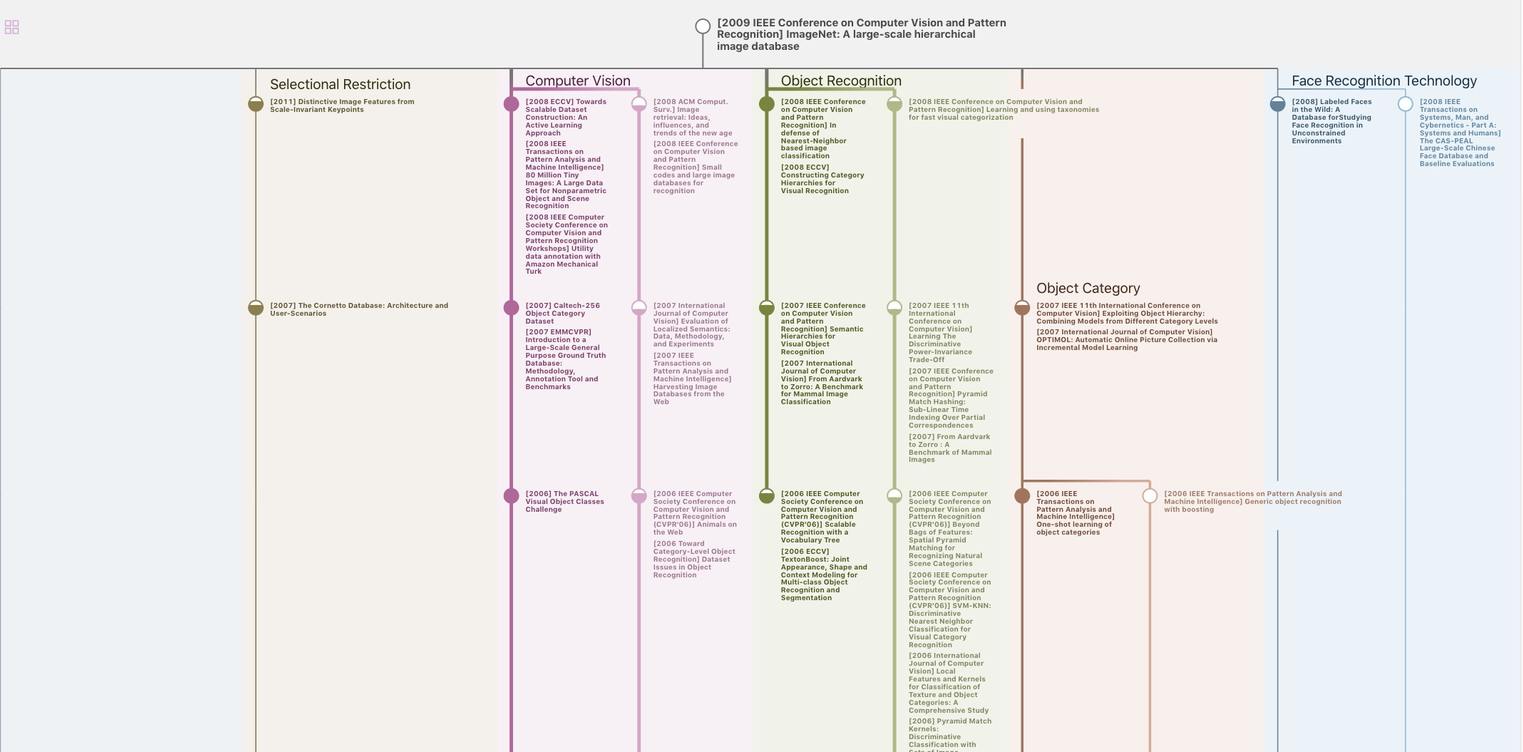
Generate MRT to find the research sequence of this paper
Chat Paper
Summary is being generated by the instructions you defined