Judgmentally Adjusted Q-values Based on Q-ensemble for Offline Reinforcement Learning
Neural Computing and Applications(2024)
摘要
Recent advancements in offline reinforcement learning (offline RL) have leveraged the Q-ensemble approach to derive optimal policies from static datasets collected in the past. By increasing the batch size, a portion of Q-ensemble instances penalizing out-of-distribution (OOD) data can be replaced, significantly reducing the Q-ensemble size while maintaining comparable performance and expediting the algorithm’s training. To further enhance the Q-ensembles’ ability to penalize OOD data, a technique involving large batch punishment and a binary classification network was employed. This method differentiates in-distribution (ID) data from OOD data. For ID data, positive adjustments to Q values were made (reward-based adjustment), whereas negative adjustments (penalty-based adjustment) were applied for OOD data, which replaced some OOD data punishment within large Q-ensembles, reducing their size without compromising performance. For different tasks on the D4RL benchmark datasets, we selectively use one of its methods. Experimental results demonstrated that employing reward-based adjustment improved algorithm performance. Simultaneously, utilizing penalty-based adjustment reduced Q-ensemble size without compromising performance. In comparison to LB-SAC, this approach reduced average convergence time by 38
更多查看译文
关键词
Offline RL,D4RL,Binary classification network,Q-ensemble
AI 理解论文
溯源树
样例
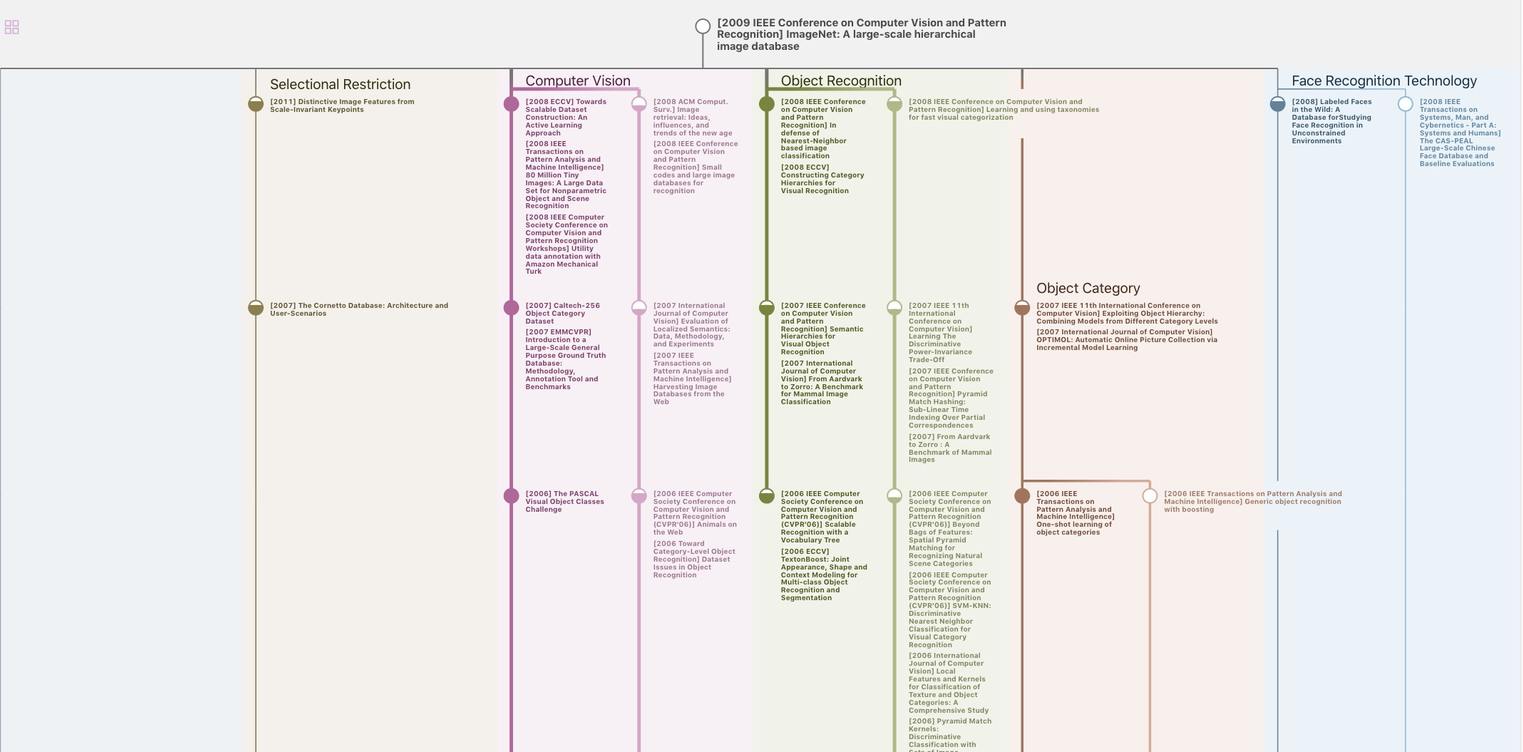
生成溯源树,研究论文发展脉络
Chat Paper
正在生成论文摘要