Multiscale Global Context Network for Semantic Segmentation of High-Resolution Remote Sensing Images.
IEEE Trans. Geosci. Remote. Sens.(2024)
摘要
Semantic segmentation of high-resolution remote sensing images (HRSIs) is a challenging task because objects in HRSIs usually have great scale variance and appearance variance. Although deep convolutional neural networks (DCNNs) have been widely applied in the semantic segmentation of HRSIs, they have inherent limitations in capturing global context. Attention mechanisms and transformer can effectively model long-range dependencies, but they often result in high computational costs when being applied to process HRSIs. In this article, an encoder-decoder network (MSGCNet) is proposed to fully and efficiently model multiscale context and long-range dependencies of HRSIs. Specifically, the multiscale interaction (MSI) module employs an efficient cross-attention to facilitate interaction among multiscale features of the encoder, which bridges the semantic gap between high- and low-level features and introduces more scale information to the network. In order to efficiently model long-range dependencies in both spatial and channel dimensions, the transformer-based decoder block (TBDB) implements window-based efficient multihead self-attention (W-EMSA) and enables interactions cross windows. Furthermore, to further integrate the global context generated by TBDB, the scale-aware fusion (SAF) module is proposed to deeply supervise the decoder, which iteratively fuses hierarchical features through spatial attention. As demonstrated by both quantitative and qualitative experimental results on two publicly available datasets, the proposed MSGCNet exhibits superior performance compared to currently popular methods. The code will be available at
http://github.com/JingxiangZhou/MSGCNet
.
更多查看译文
关键词
Attention mechanism,remote sensing images,semantic segmentation,transformer
AI 理解论文
溯源树
样例
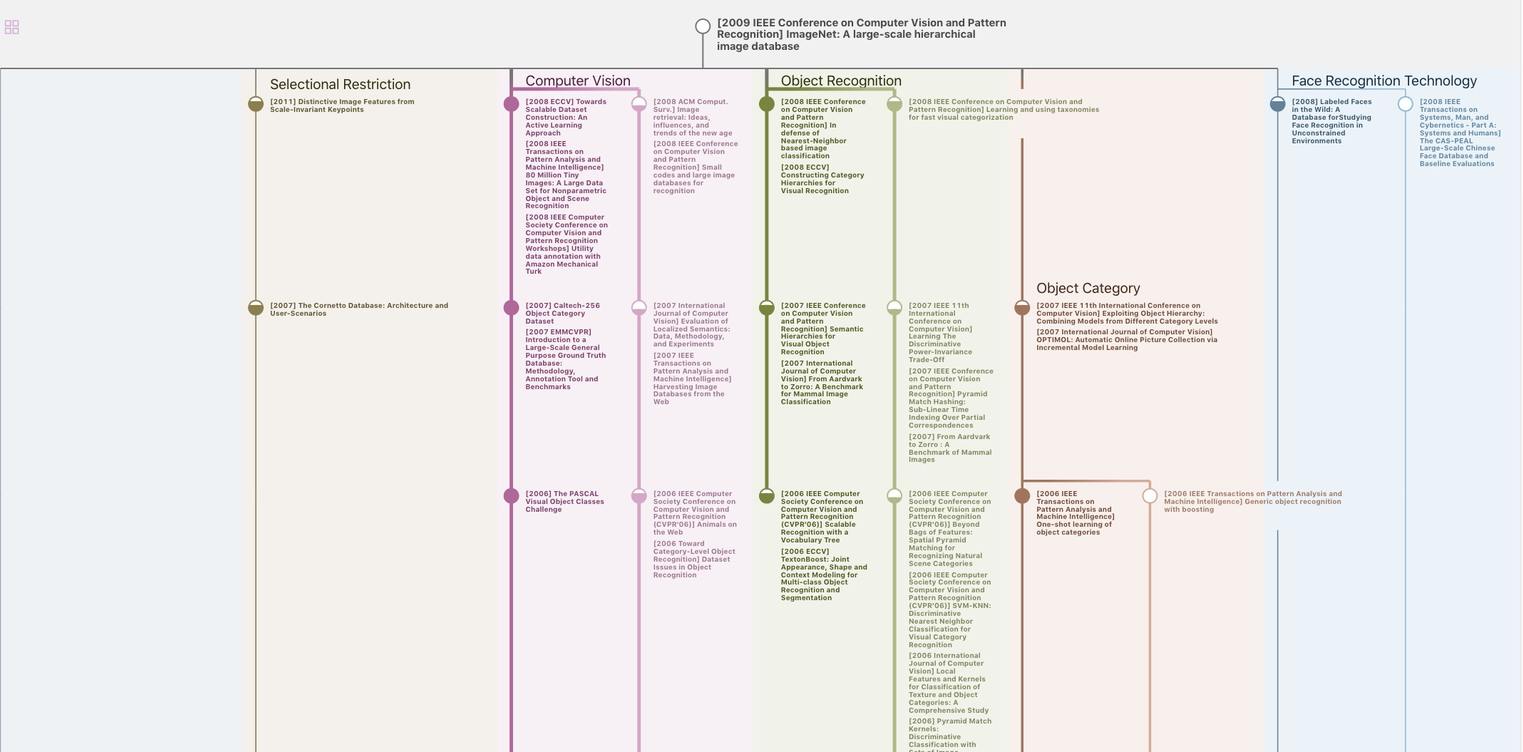
生成溯源树,研究论文发展脉络
Chat Paper
正在生成论文摘要