A Deep Learning Approach For Epistemic Uncertainty Quantification Of Turbulent Flow Simulations
arxiv(2024)
摘要
Simulations of complex turbulent flow are part and parcel of the engineering design process. Eddy viscosity based turbulence models represent the workhorse for these simulations. The underlying simplifications in eddy viscosity models make them computationally inexpensive but also introduce structural uncertainties in their predictions. Currently the Eigenspace Perturbation Method is the only approach to predict these uncertainties. Due to its purely physics based nature this method often leads to unrealistically large uncertainty bounds that lead to exceedingly conservative designs. We use a Deep Learning based approach to address this issue. We control the perturbations using trained deep learning models that predict how much to perturb the modeled Reynolds stresses. This is executed using a Convolutional Neural Network that learns the difference between eddy viscosity based model predictions and high fidelity data as a mapping of flow features. We show that this approach leads to improvements over the Eigenspace Perturbation Method.
更多查看译文
AI 理解论文
溯源树
样例
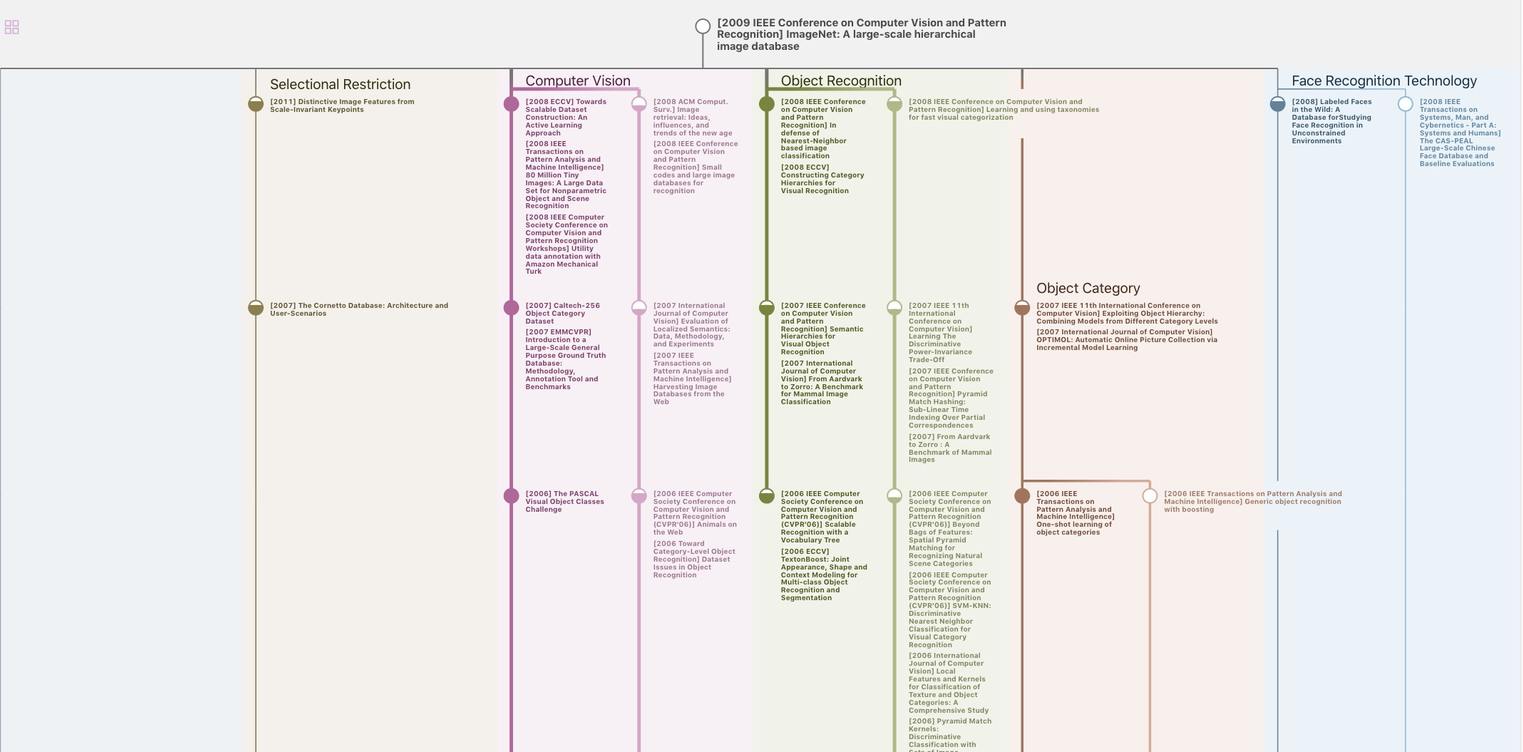
生成溯源树,研究论文发展脉络
Chat Paper
正在生成论文摘要