An adaptive enrichment design using Bayesian model averaging for selection and threshold-identification of tailoring variables
arxiv(2024)
摘要
Precision medicine stands as a transformative approach in healthcare, offering tailored treatments that can enhance patient outcomes and reduce healthcare costs. As understanding of complex disease improves, clinical trials are being designed to detect subgroups of patients with enhanced treatment effects. Biomarker-driven adaptive enrichment designs, which enroll a general population initially and later restrict accrual to treatment-sensitive patients, are gaining popularity. Current practice often assumes either pre-trial knowledge of biomarkers defining treatment-sensitive subpopulations or a simple, linear relationship between continuous markers and treatment effectiveness. Motivated by a trial studying rheumatoid arthritis treatment, we propose a Bayesian adaptive enrichment design which identifies important tailoring variables out of a larger set of candidate biomarkers. Our proposed design is equipped with a flexible modelling framework where the effects of continuous biomarkers are introduced using free knot B-splines. The parameters of interest are then estimated by marginalizing over the space of all possible variable combinations using Bayesian model averaging. At interim analyses, we assess whether a biomarker-defined subgroup has enhanced or reduced treatment effects, allowing for early termination due to efficacy or futility and restricting future enrollment to treatment-sensitive patients. We consider pre-categorized and continuous biomarkers, the latter of which may have complex, nonlinear relationships to the outcome and treatment effect. Using simulations, we derive the operating characteristics of our design and compare its performance to two existing approaches.
更多查看译文
AI 理解论文
溯源树
样例
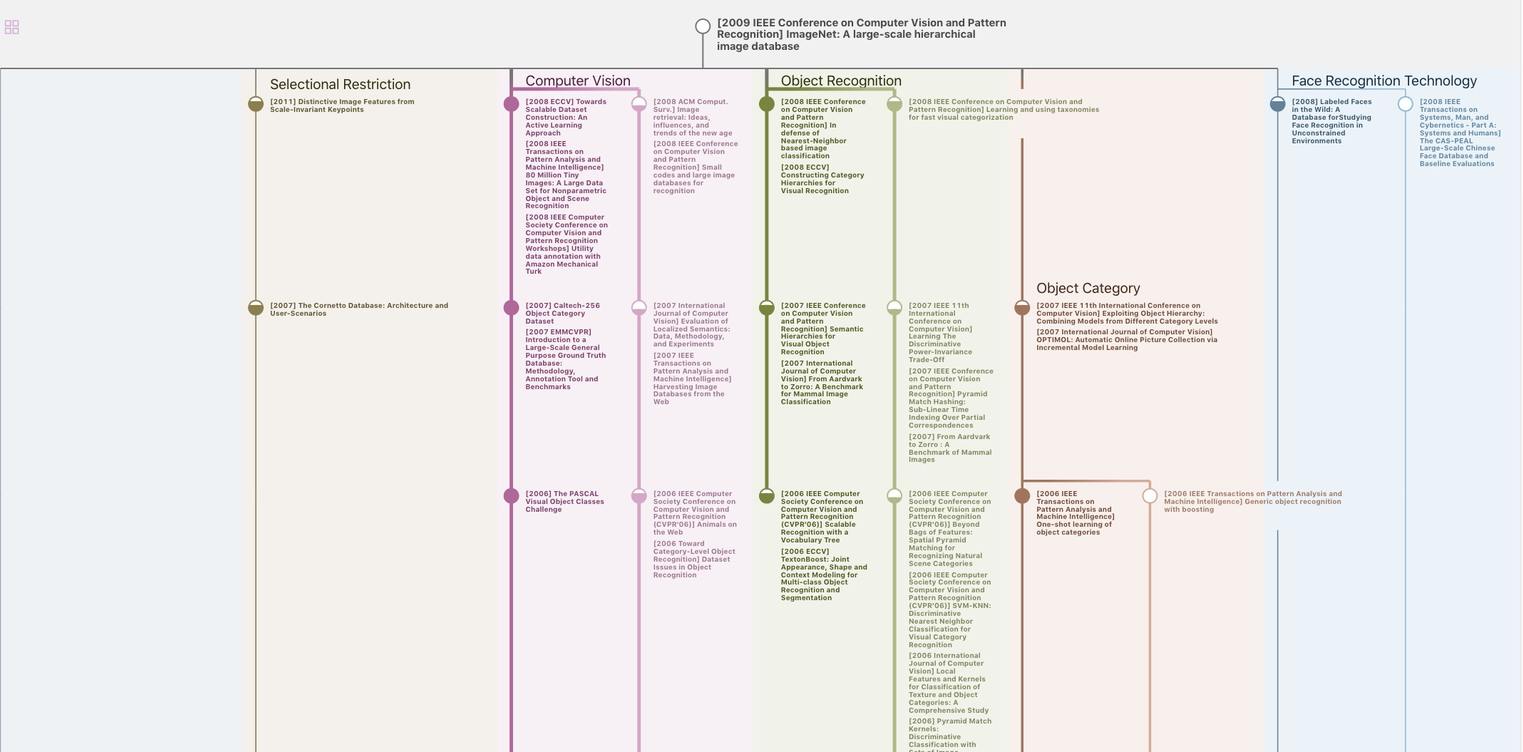
生成溯源树,研究论文发展脉络
Chat Paper
正在生成论文摘要