Using Density and Fuzzy Clustering for Data Cleaning and Segmental Description of Livestock Data
Journal of Agricultural, Biological and Environmental Statistics(2024)
摘要
The cluster algorithms density-based clustering with noise and fuzzy c-means were used to edit and group a large, noisy data set from a livestock herd recording scheme consisting of slaughter records on 73,743 bulls. Density-based clustering with noise was used for data selection with ϵ =0.06 and minPts = 8. The remaining data ( n = 65,446 ) was exposed to a fuzzy c-means analysis to partition data based on three variables: Age at slaughter, carcass weight, and average daily carcass gain. Appropriate number of clusters was chosen by the maximum value of the modified partition coefficient ( k = 3 clusters). Cluster validation for both hard cluster assignment and cluster membership was performed with linear models and a permutation test. The three clusters had centroid values for slaughter age and carcass weight interpreted as: Part of production systems characterized by high bull turnover (Cluster 1), production systems aiming for heavy slaughter weights (Cluster 2), and a less intensive system with higher roughage proportions (Cluster 3). The results show that the approach can be successfully combined to segment meaningful groups from large, noisy industry data, exemplified by the description of slaughter performance records. Supplementary materials accompanying this paper appear online.
更多查看译文
关键词
Beef production,DBSCAN,Fuzzy c-means,Norwegian red
AI 理解论文
溯源树
样例
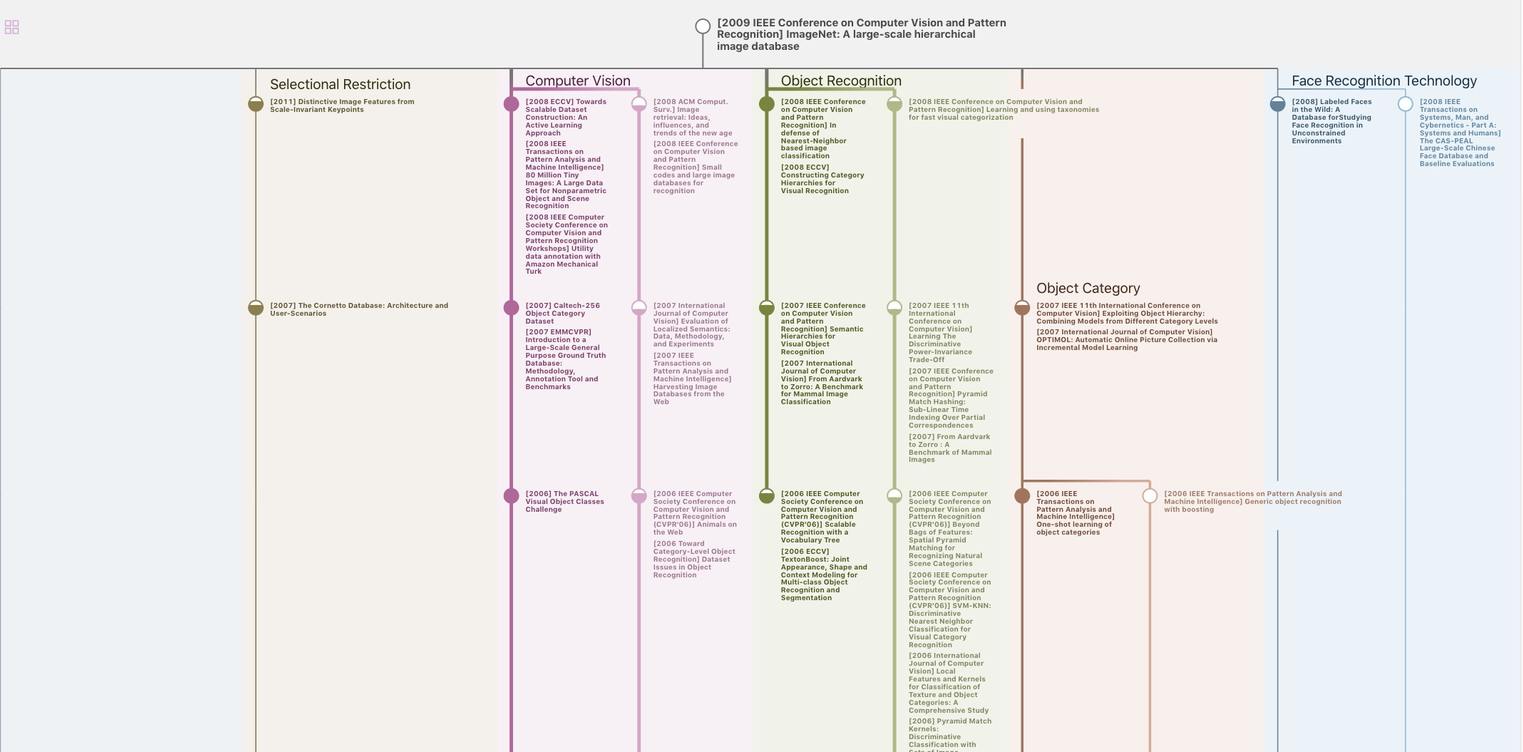
生成溯源树,研究论文发展脉络
Chat Paper
正在生成论文摘要