Visible and Infrared Lab Spectroscopy for Soil Texture Classification: Analysis of Entire Spectra V/s Reduced Spectra
Remote sensing applications(2024)
摘要
Soil, directly or indirectly, impacts seven of the Sustainable Development Goals set by the United Nations. Soil texture is a fundamental physical property that controls other properties ranging from soil stability, erodibility, compaction to water holding capacity, nutrient availability and fertility. While Visible Near-Infrared and Shortwave-Infrared (VNIR/SWIR) laboratory spectroscopy has been already used for soil texture classification, Mid Infrared (MIR) region and unison of the VNIR/SWIR and MIR region warrant quantification. Thus, the objectives of this work were: 1) to investigate the critical spectral regions for soil texture classification, 2) to compare the use of entire spectral bands v/s subset of spectral bands obtained via the feature selection method, and 3) to quantify the degree of misclassification in the neighbouring classes for a given textural class. This study utilized an ICRAF–ISRIC global soil spectral library consisting of 3643 VNIR/SWIR, MIR soil spectra and the USDA soil textural classes.Two classifiers were used for soil texture classification using the three databases (VNIR/SWIR, MIR, VNIR+MIR) with entire spectral bands and three databases with only the Partial Information Correlation (PIC) selected bands (VNIR/SWIR_PIC, MIR_PIC, VNIR+MIR_PIC). The mean confusion matrix, overall accuracy and kappa were calculated to evaluate classifiers' performances over 100 iterations. Two additional measures were proposed for partitioning inaccuracies between neighbouring and far classes: Correct-Neighbouring-Far classes distribution matrix and added neighbourhood accuracy. This work highlighted that: 1) the combined VNIR/SWIR+MIR region provided the best texture classification, followed by MIR and then VNIR/SWIR region, 2) the use of PIC selected bands provided lower classification performances when compared to using all bands but a massive reduction in the number of bands allowing to reduce model complexity, 3) the misclassifications were predominantly in the neighbouring classes rather than far classes, for a given texture class. Finally, the textural classes with poor classification performance had low areal and sample representation. These insights may launch a number of soil texture classification studies worldwide based on global soil spectral databases, further helping map soils and the associated ecosystem services it provides.
更多查看译文
关键词
Soil texture classification,ICRAF–ISRIC Global Soil Spectroscopy Library,USDA texture triangle,Feature Selection,Added Neighbourhood Accuracy
AI 理解论文
溯源树
样例
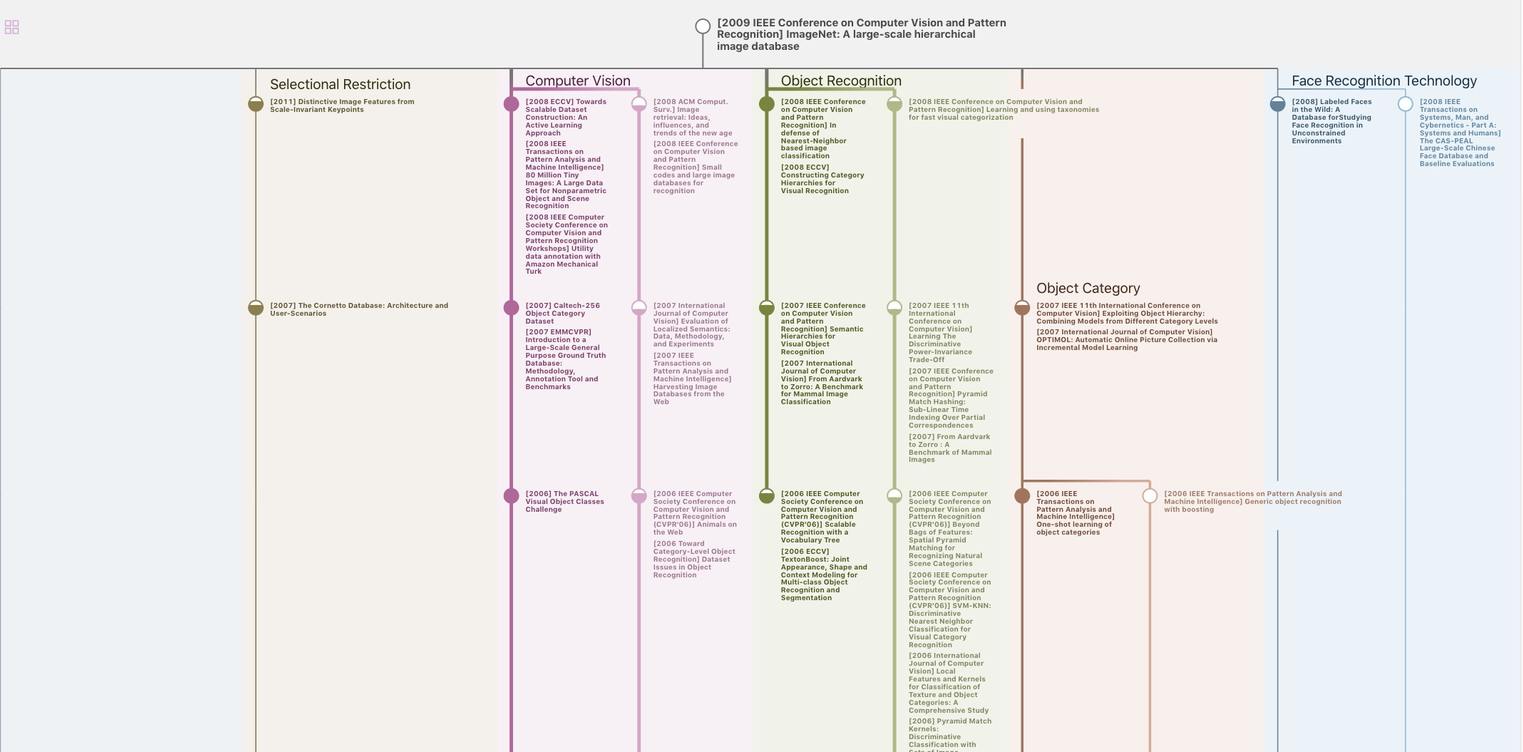
生成溯源树,研究论文发展脉络
Chat Paper
正在生成论文摘要