Improving Distributed Acoustic Sensing Data Quality with Self-Supervised Learning
IEEE Geoscience and Remote Sensing Letters(2024)
摘要
Nowadays, one of the predominant deep learning approaches to improve the quality of DAS VSP seismic data is executed through supervised learning, which requires paired training set including data simulation with relevant parameters and solutions of elastic wave equations. However, differences between simulated data and field data in terms of signal regulations and noise distributions often leads to poor results. An alternative approach is self-supervised learning, such as the representative framework--Blind Spot Network (BSN), but unfortunately, the effective information in blind spots cannot be fully utilized. To solve this problem, this paper considers BSN as a basis and establishes a novel self-supervised network--blind spot visualization (BSV) to suppress random noise and improve the quality of DAS VSP data. In BSV, one branch is dedicated to first produce more denoised data with blind spots and then recover the valid information covered by the blind spots, assuming that the signal is partially data-dependent and the DAS noise is conditionally data-independent. The other branch is designed to generate a target for training without blind spots, so that the dual-branch network can accomplish a self-supervised task in the way of supervised learning. More than that, unlike BSN, we utilize a tailor-made blind spot mapper (BSM) to recover effective information in the blind spots. Results of field data testing prove BSV’s advantages in suppressing random noise and improving the quality of DAS VSP data, although test on synthetic data is nearly identical to supervised learning.
更多查看译文
关键词
Seismic data denoising,Self-supervised deep learning,Blind Spot Network / BSN,Blind Spot Visualization / BSV,DAS VSP data
AI 理解论文
溯源树
样例
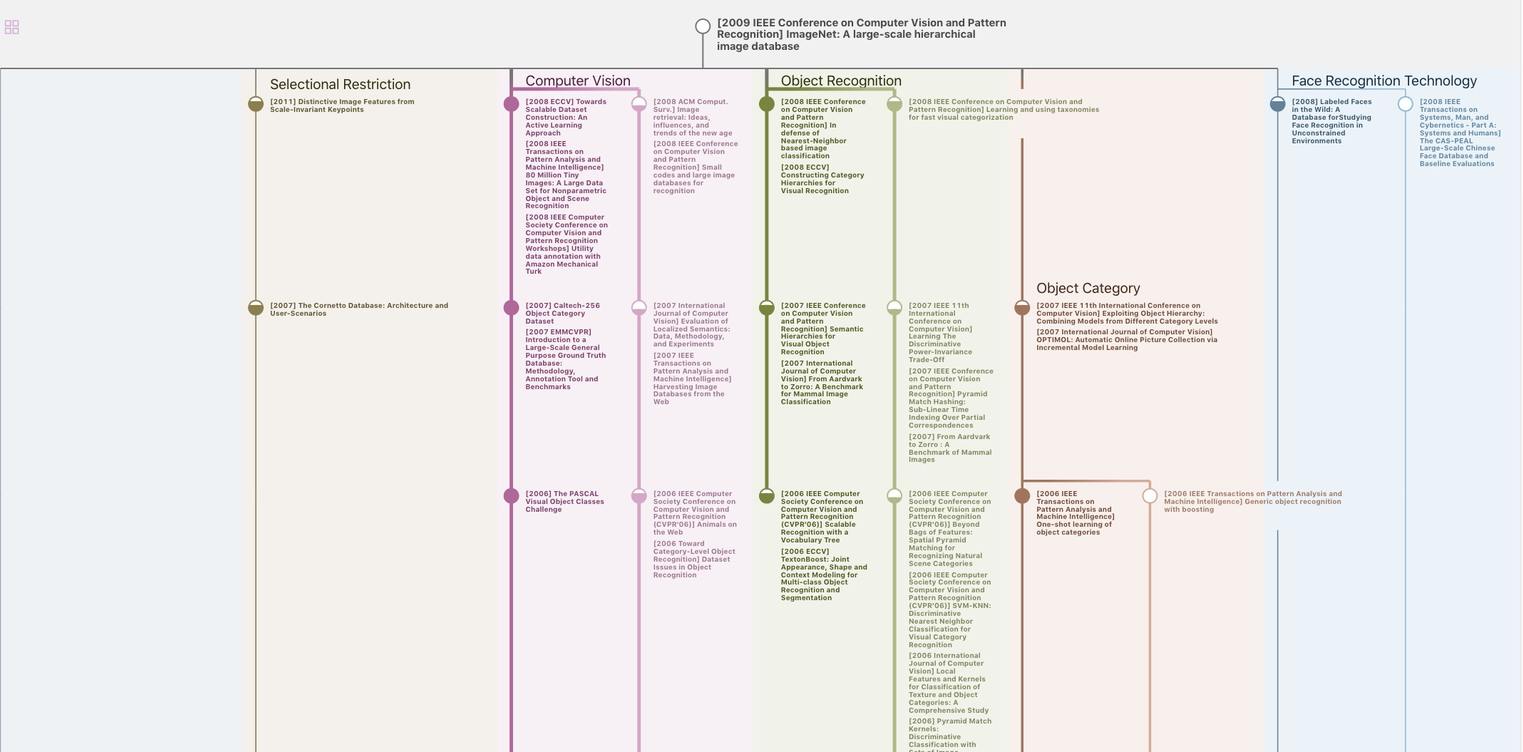
生成溯源树,研究论文发展脉络
Chat Paper
正在生成论文摘要