Fragrant: frequency-auxiliary guided relational attention network for low-light action recognition
The Visual Computer(2024)
摘要
Video action recognition aims to classify actions within sequences of video frames, which has important applications in computer vision fields. Existing methods have shown proficiency in well-lit environments but experience a drop in efficiency under low-light conditions. This decline is due to the challenge of extracting relevant information from dark, noisy images. Furthermore, simply introducing enhancement networks as preprocessing will lead to an increase in both parameters and computational burden for the video. To address this dilemma, this paper presents a novel frequency-based method, FRequency-Auxiliary Guided Relational Attention NeTwork (FRAGRANT), designed specifically for low-light action recognition. Its distinctive features can be summarized as: (1) a novel Frequency-Auxiliary Module that focuses on informative object regions, characterizing action and motion while effectively suppressing noise; (2) a sophisticated Relational Attention Module that enhances motion representation by modeling the local s between position neighbors, thereby more efficiently resolving issues, such as fuzzy boundaries. Comprehensive testing demonstrates that FRAGRANT outperforms existing methods, achieving state-of-the-art results on various standard low-light action recognition benchmarks.
更多查看译文
关键词
Low-light action recognition,Frequency domain analysis,Relational attention mechanism,Motion representation,Action boundary
AI 理解论文
溯源树
样例
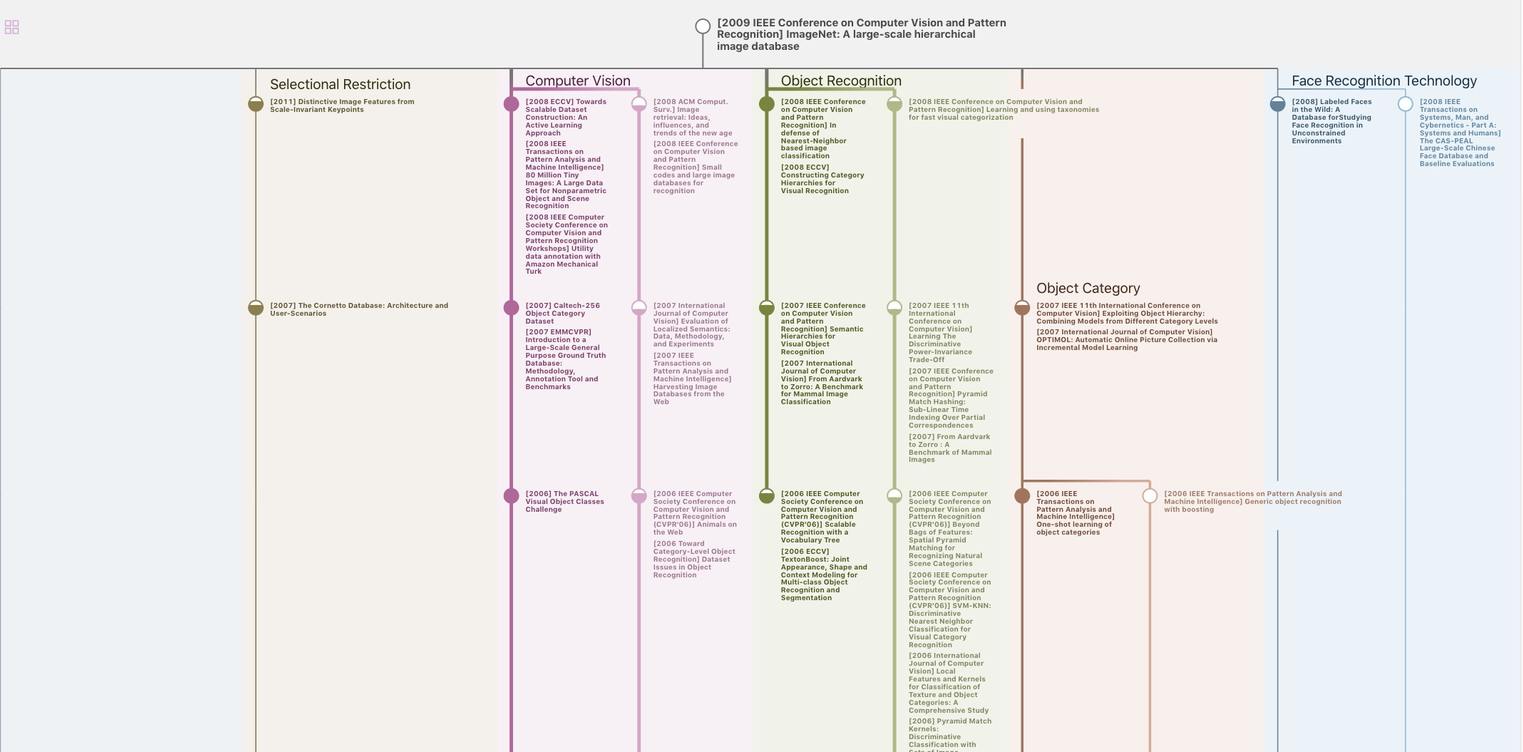
生成溯源树,研究论文发展脉络
Chat Paper
正在生成论文摘要