A support vector machine model of landslide susceptibility mapping based on hyperparameter optimization using the Bayesian algorithm: a case study of the highways in the southern Qinghai–Tibet Plateau
Natural Hazards(2024)
摘要
Recent advancements have seen a pervasive application of machine learning methodologies in assessing the susceptibility of geological hazards. A pivotal element influencing the accuracy of model predictions resides in the prudent selection of model parameters within machine learning frameworks. The objective of this study is to develop a robust landslide susceptibility assessment model by refining the support vector machine (SVM) model through the employment of the Bayesian algorithm for hyperparameter optimization. The southern part of the Qinghai-Tibet Plateau, focusing on major highways, is selected as the study area. Nine influencing factors, namely the elevation, slope, aspect, profile curvature, lithology, topographic wetness index, normalized difference vegetation index, distance to faults, and distance to rivers, are selected as the conditioning variables instrumental in evaluating the likelihood of collapse occurrences. Secondly, data from field surveys involving 351 landslides and randomly generated non-landslide data are utilized in a balanced 1:1 ratio to construct the training and testing datasets. Next, the cross-validation loss rate of the SVM model is selected as the objective function, and the Bayesian algorithm is used to optimize the BoxConstraint and KernelScale parameters of the SVM model, resulting in a Bayesian optimization-based SVM model. The results show that, within a five-fold cross-validation framework, the model yields 99.15
更多查看译文
关键词
Landslide,SVM,Bayesian optimization,Susceptibility mapping
AI 理解论文
溯源树
样例
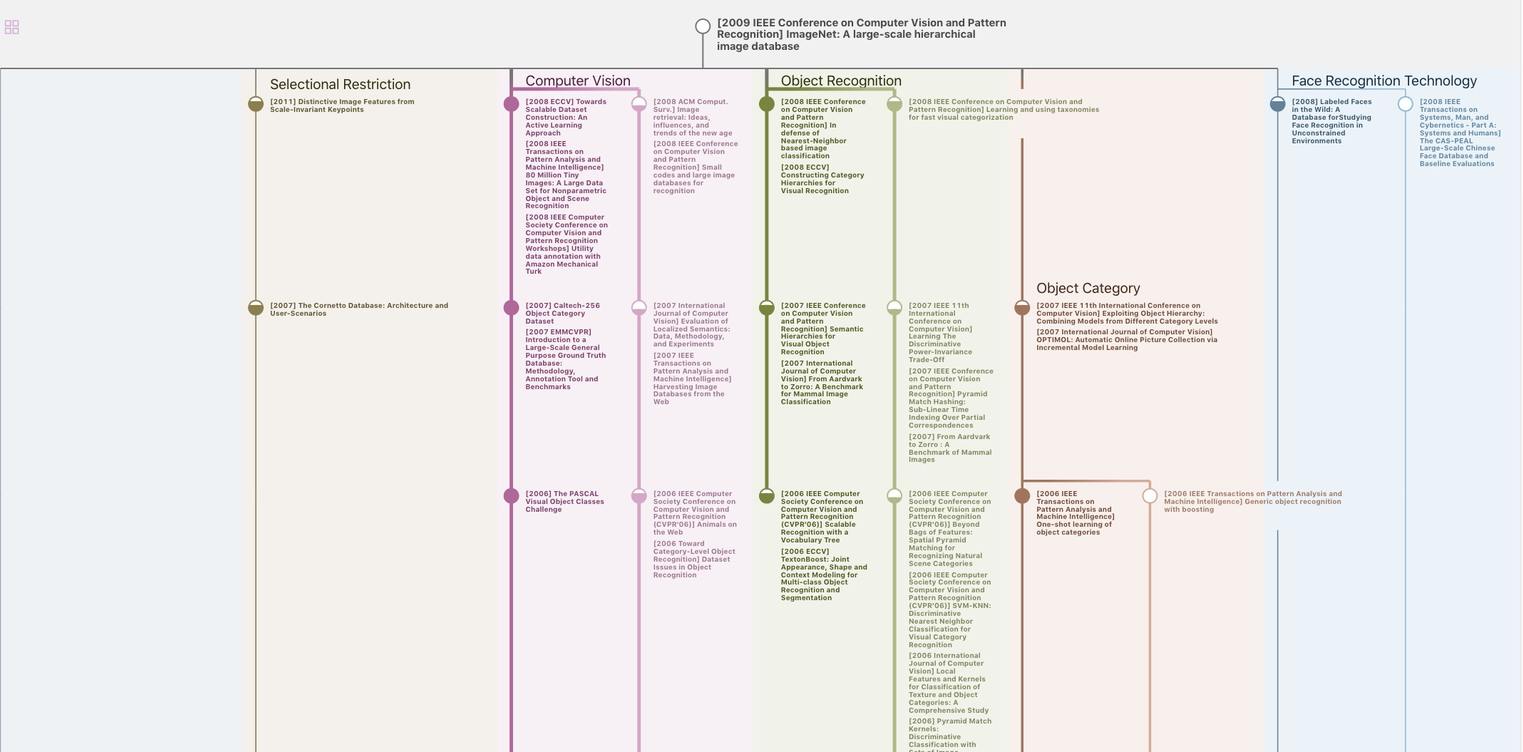
生成溯源树,研究论文发展脉络
Chat Paper
正在生成论文摘要