A 28nm 8928Kb/mm 2 -Weight-Density Hybrid SRAM/ROM Compute-in-Memory Architecture Reducing >95% Weight Loading from DRAM.
IEEE Custom Integrated Circuits Conference(2024)
摘要
Large transformer networks have demonstrated remarkable advancements in various AI tasks, However, the explosive growth of parameter causes severe challenges for AI accelerators because of the huge amount of data movement. Compute-in-memory (CiM) has thus been proposed as a competitive approach to the reduction of data movement [2–6]. However, three main challenges limit the energy efficiency of CiM. Firstly, the limited on-chip memory capacity severely affects the task-level efficiency due to frequent weight reload from DRAM. This challenge can be addressed from the insight that large pre-trained models can be adapted to various downstream tasks with most of the weights unchanged. Therefore, a hybrid “ultra-dense-ROM + flexible-SRAM” CiM structure can lead to a significant reduction in off-chip DRAM access. Secondly, ADCs with lower resolution can significantly reduce the overhead but will cause pernicious impact to the accuracy. The proposed adaptive-resolution ADC, which accumulates 2b updates onto a 5b partial sum, can reduce the conversion overhead while ensuring high accuracy. Thirdly, the energy efficiency of charge-domain computing is limited due to large computing capacitors that are difficult to scale down under variations. This work reduces the computing capacitors substantially with post-fabrication 1-of-N capacitor selection (PFCS).
更多查看译文
关键词
Energy Efficiency,Density Data,Additional Weight,High Energy Efficiency,Reduction In Availability,Amount Of Movement,Transformer Layers,Reduction In Movement,Ultrahigh Density,Capacitor Size,Limited Memory Capacity,Sign Bit
AI 理解论文
溯源树
样例
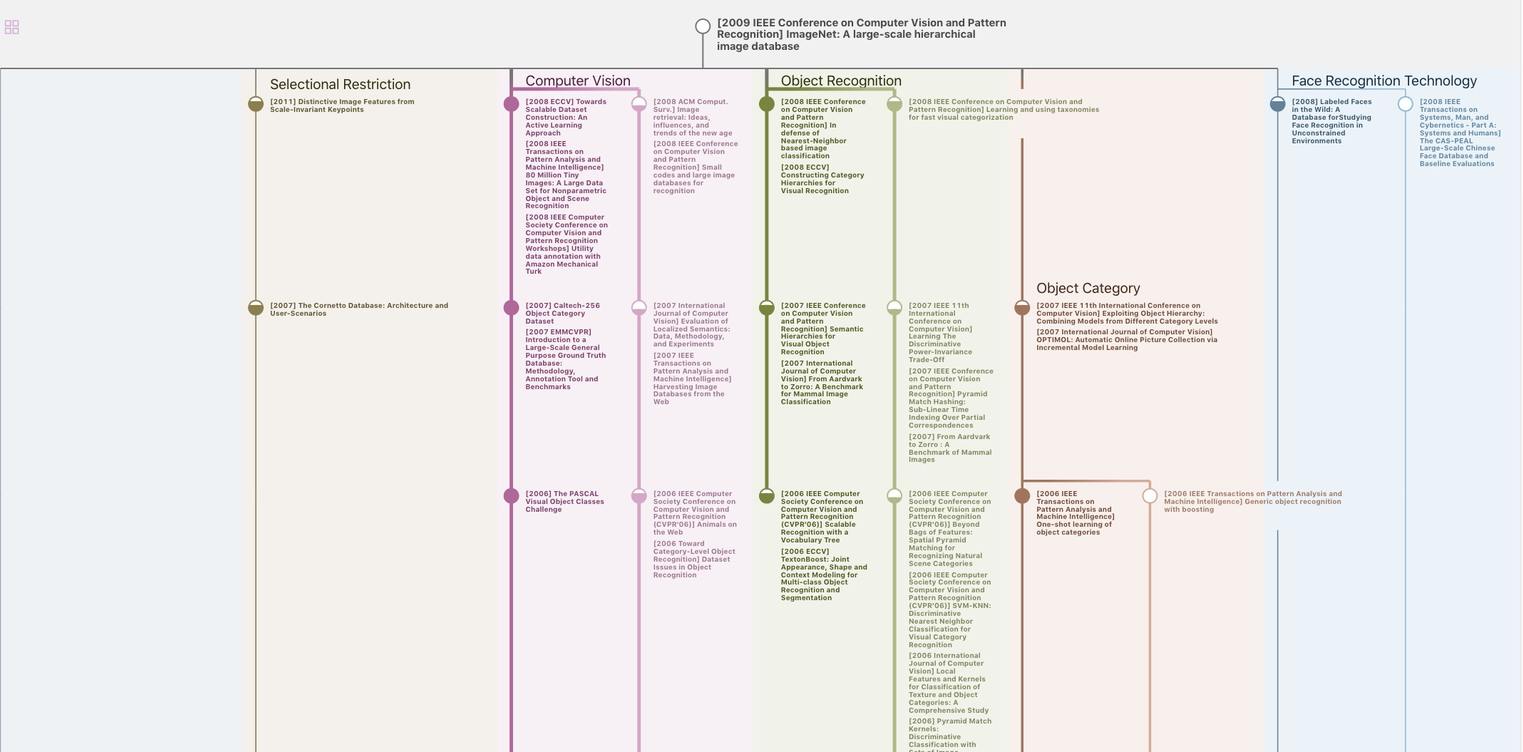
生成溯源树,研究论文发展脉络
Chat Paper
正在生成论文摘要