A multi-source information fusion method for tool life prediction based on CNN-SVM
Mechanical Engineering Science(2022)
摘要
For milling tool life prediction and health management, accurate extraction and dimensionality reduction of its tool wear features are the key to reduce prediction errors. In this paper, we adopt multi-source information fusion technology to extract and fuse the features of cutting vibration signal, cutting force signal and acoustic emission signal in time domain, frequency domain and time-frequency domain, and downscale the sample features by Pearson correlation coefficient to construct a sample data set; then we propose a tool life prediction model based on CNN-SVM optimized by genetic algorithm (GA), which uses CNN convolutional neural network as the feature learner and SVM support vector machine as the trainer for regression prediction. The results show that the improved model in this paper can effectively predict the tool life with better generalization ability, faster network fitting, and 99.85% prediction accuracy. And compared with the BP model, CNN model, SVM model and CNN-SVM model, the performance of the coefficient of determination R2 metric improved by 4.88%, 2.96%, 2.53% and 1.34%, respectively.
更多查看译文
AI 理解论文
溯源树
样例
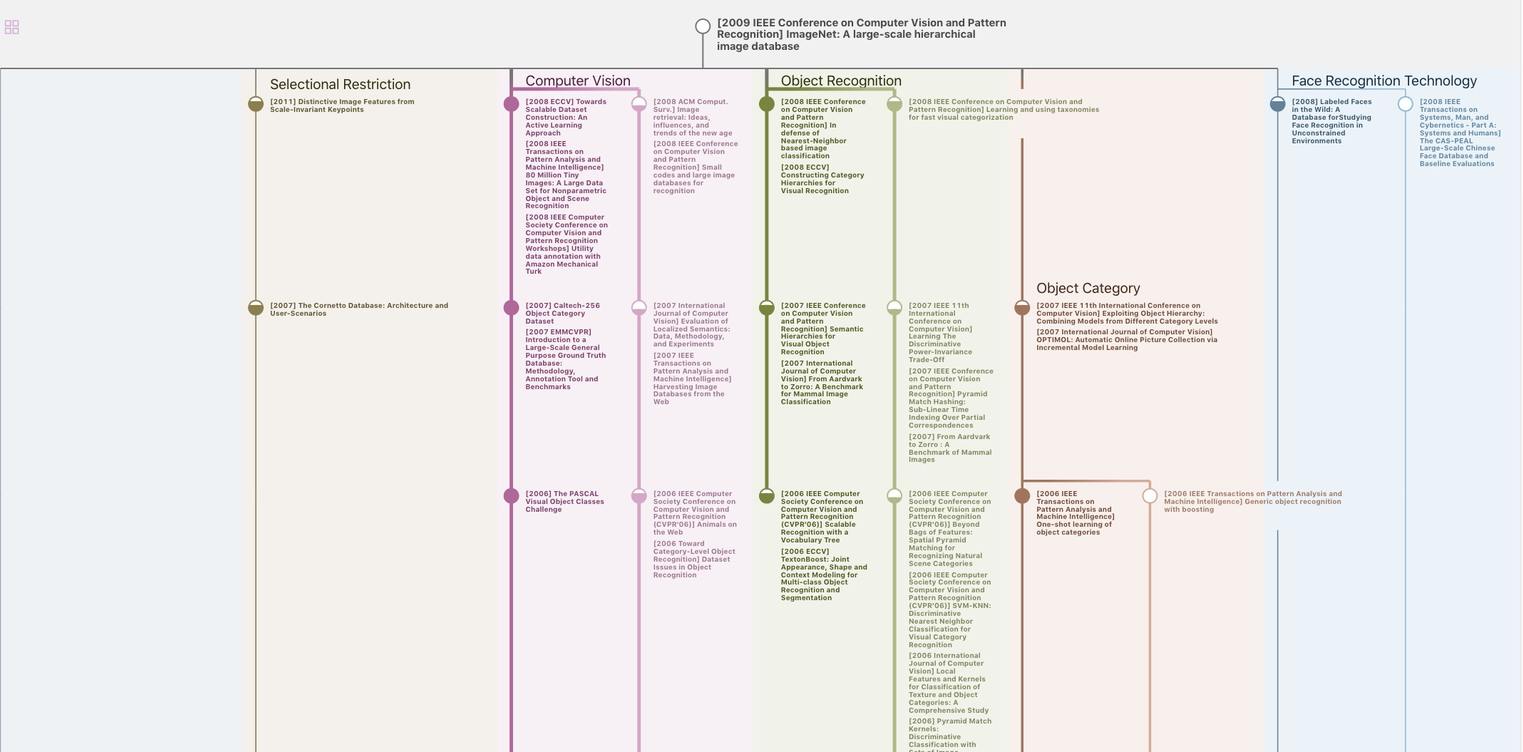
生成溯源树,研究论文发展脉络
Chat Paper
正在生成论文摘要