Prognostic value of deep learning based RCA PCAT and plaque volume beyond CT-FFR in patients with stent implantation
crossref(2024)
摘要
Abstract The study aims to investigate the prognostic value of deep learning based pericoronary adipose tissue attenuation computed tomography (PCAT) and plaque volume beyond coronary computed tomography angiography (CTA) -derived fractional flow reserve (CT-FFR) in patients with percutaneous coronary intervention (PCI). A total of 183 patients with PCI who underwent coronary CTA were included in this retrospectively study. Imaging assessment included PCAT, plaque volume and CT-FFR which were performed using an artificial intelligence (AI) assisted workstation. Kaplan-Meier and multivariate Cox regression were used to estimate major adverse cardiovascular events (MACE) including non-fatal myocardial infraction (MI), stroke and mortality. In total, 22 (12%) MACE occurred during the median follow-up of 38.0 months (interquartile range 34.6–54.6 months). Kaplan-Meier survival curves indicated that right coronary artery (RCA) PCAT (p = 0.007) and plaque volume (p = 0.008) were significantly associated with the increasing of MACE. Multivariable Cox regression analysis showed that RCA PCAT [hazard ratios (HR): 2.94, 95%CI: 1.15–7.50, p = 0.025] and plaque volume (HR: 3.91, 95%CI: 1.20-12.75, p = 0.024) were independent predictors of MACE after adjusting for clinical risk factors. However, CT-FFR was not independently associated with MACE in multivariable Cox regression (p = 0.271). Deep learning based RCA PCAT and plaque volume derived from coronary CTA was found to be more strongly associated with MACE than CT-FFR in patients with PCI.
更多查看译文
AI 理解论文
溯源树
样例
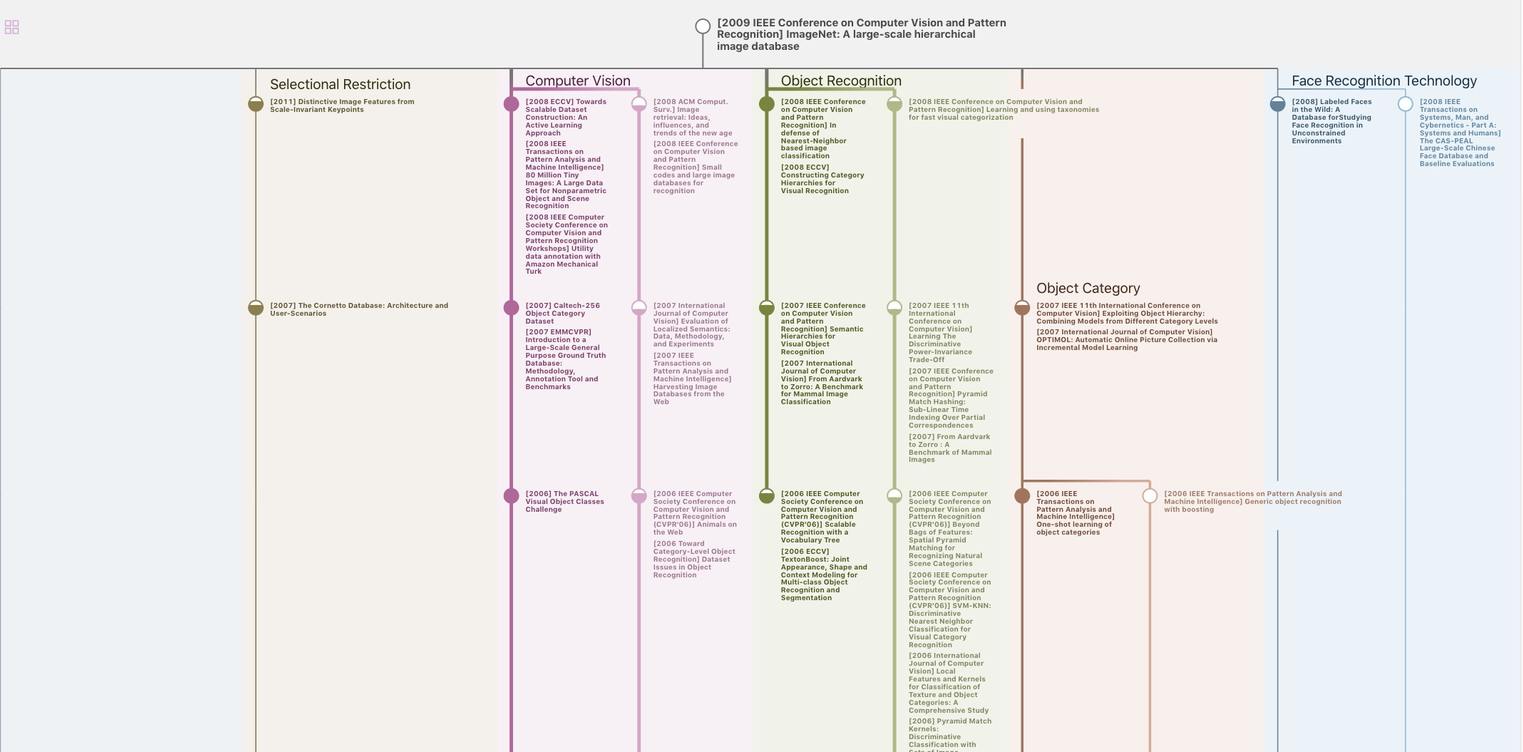
生成溯源树,研究论文发展脉络
Chat Paper
正在生成论文摘要