Enhancing Internet of Smart City Security: Utilizing Logistic Boosted Algorithms for Anomaly Detection and Cyberattack Prevention
SN Computer Science(2024)
摘要
With the increasing deployment of Internet of Things (IoT) devices in Smart Cities, ensuring the security and integrity of these systems is of paramount importance. This study focuses on the detection of anomalies and prevention of security attacks in Smart City environments, specifically in the context of traffic management. We compare the performance of three machine learning algorithms, namely logistic boosted algorithms, random forest, and support vector machines, using a dataset collected from various Smart City devices. Through comprehensive evaluation metrics such as accuracy, precision, recall, and F1 score, we analyze the efficacy of each algorithm. Our results indicate that the logistic boosted algorithm outperforms the other approaches in accurately identifying data outliers and achieving high performance across all evaluation metrics. Notably, it excels in handling imbalanced datasets and leveraging ensemble methods to enhance overall performance. While the random forest algorithm demonstrates exceptional performance in all metrics, the support vector machines approach exhibits superior recall but comparatively lower accuracy, precision, and F1 score. Moreover, the logistic boosted method showcases fewer misclassifications in the confusion matrices, making it highly effective for anomaly detection. The area under the ROC curve is highest for the logistic boosted algorithm (0.98), further emphasizing its effectiveness in filtering out anomalies. The random forest algorithm achieves the highest area under the curve (0.982), while the support vector machines method shows the lowest (0.968). Based on our findings, we conclude that the logistic boosted algorithm is the most effective for monitoring Smart City systems, followed by the random forest approach. However, the support vector machines method can be a viable alternative if recall is the primary performance metric of concern. Future research can focus on comparing the efficacy of different machine learning approaches for anomaly detection in Smart City environments and exploring the impact of unique dataset properties on algorithm performance. Additionally, exploring the potential of deep learning algorithms for traffic anomaly detection in Smart City systems could be a promising area of study. This study contributes to the advancement of state-of-the-art anomaly detection algorithms for ensuring the security and efficiency of Smart City applications.
更多查看译文
关键词
Traffic anomaly detection,Cyber security,Logistic boosted algorithms,Smart Cities,Internet of Things (IoT)
AI 理解论文
溯源树
样例
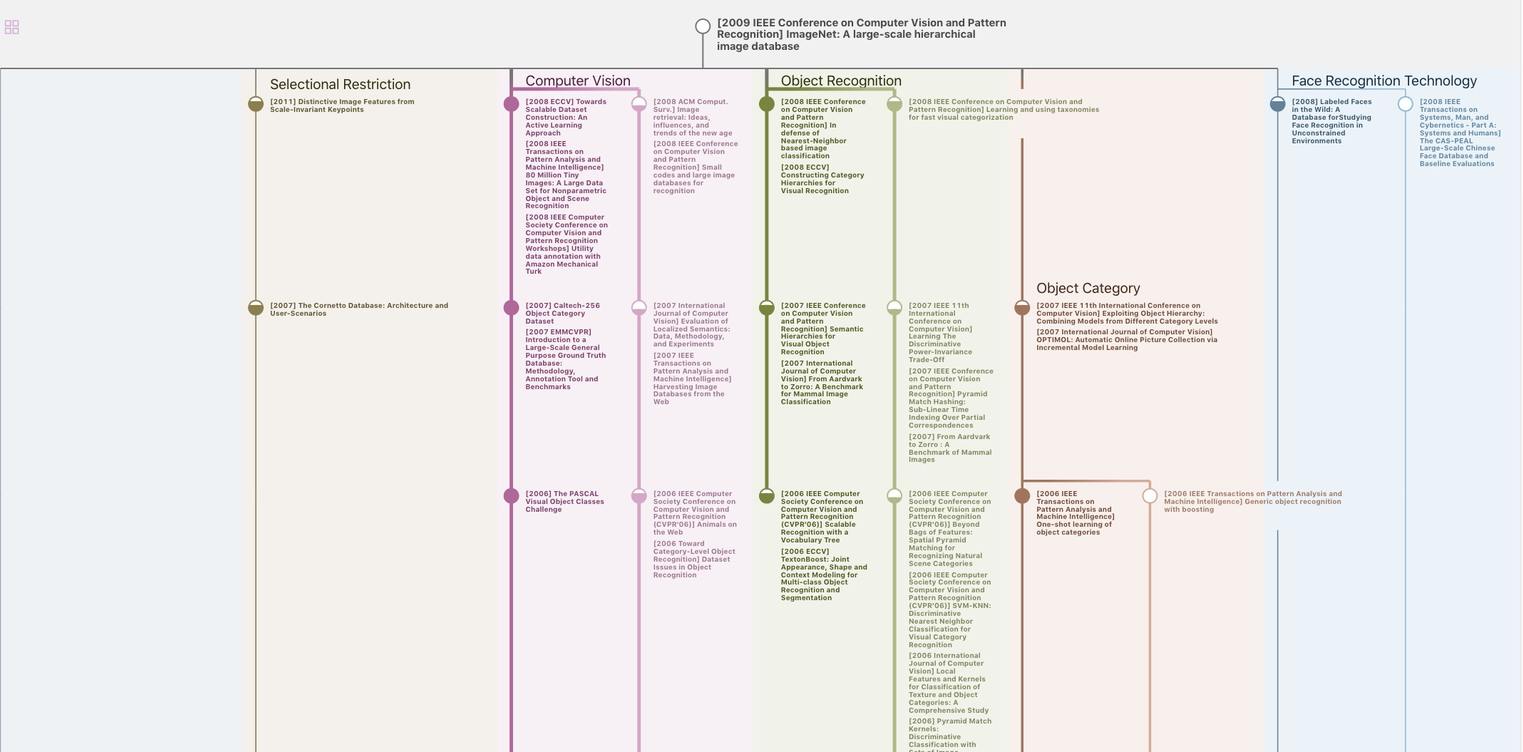
生成溯源树,研究论文发展脉络
Chat Paper
正在生成论文摘要