Rapid parameter estimation for pulsar-timing-array datasets with variational inference and normalizing flows
arxiv(2024)
摘要
In the gravitational-wave analysis of pulsar-timing-array datasets, parameter
estimation is usually performed using Markov Chain Monte Carlo methods to
explore posterior probability densities. We introduce an alternative procedure
that relies instead on stochastic gradient-descent Bayesian variational
inference, whereby we obtain the weights of a neural-network approximation of
the posterior by minimizing the Kullback-Leibler divergence of the
approximation from the exact posterior. This technique is distinct from
simulation-based inference with normalizing flows, since we train the network
for a single dataset, rather than the population of all possible datasets, and
we require the computation of the data likelihood and its gradient. Unlike
Markov Chain methods, our technique can transparently exploit highly parallel
computing platforms. This makes it extremely fast on modern graphical
processing units, where it can analyze the NANOGrav 15-yr dataset in few tens
of minutes, depending on the probabilistic model, as opposed to hours or days
with the analysis codes used until now. We expect that this speed will unlock
new kinds of astrophysical and cosmological studies of pulsar-timing-array
datasets. Furthermore, variational inference would be viable in other contexts
of gravitational-wave data analysis as long as differentiable and
parallelizable likelihoods are available.
更多查看译文
AI 理解论文
溯源树
样例
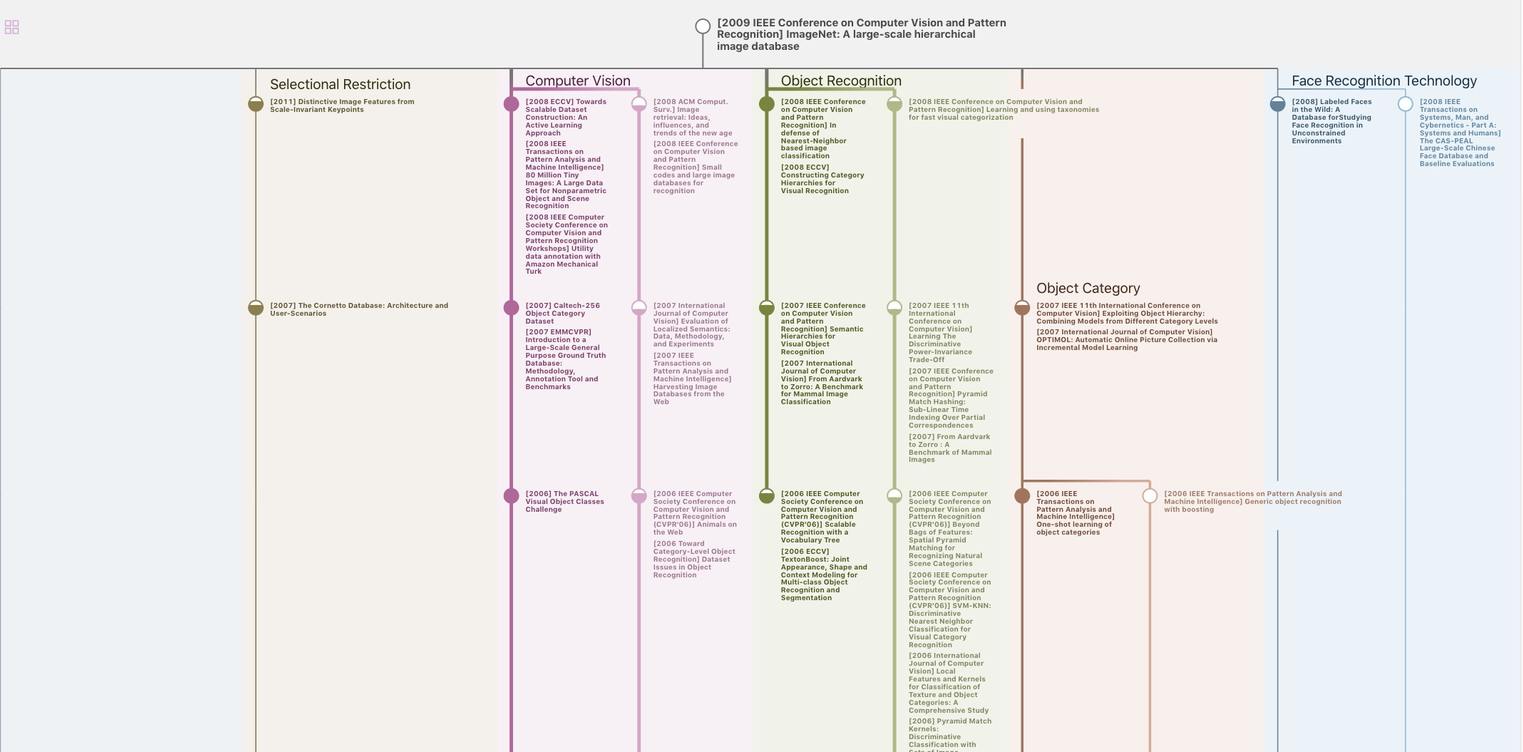
生成溯源树,研究论文发展脉络
Chat Paper
正在生成论文摘要