Handling Imbalanced Data for Detecting Scams in Ethereum Transactions Using Sampling Techniques.
International Symposium on Digital Forensics and Security(2024)
摘要
Blockchain technology and cryptocurrencies have captured global attention due to their numerous and versatile features, resulting in several industries and services adopting cryptocurrencies as payment methods. The advantages including user anonymity, open source, and tamper-proof transactions, have contributed to its popularity. However, these advantages have also attracted scammers who exploit the technology's features to engage in fraudulent activities, leading to a growth in crypto-frauds. To prevent and identify these frauds, various detection and prevention methodologies have been proposed, mainly using machine learning algorithms to identify scams as anomalies or outliers. The performance of such models depends on the datasets used and the features engineered. Often, these models face the challenge of having limited amounts of data that are scam-labeled. Under such circumstances, the model performs poorly due to an imbalanced dataset. Similarly, the features engineered and selected to train the model are also very important in detecting scams. With the help of sampling techniques, we propose to create a dataset that is researchready and addresses the data imbalance problem. Additionally, we list the resources that can be used to collect labeled data. Furthermore, we discuss the practical significance of features and various feature engineering strategies in detecting scams from transactions in Ethereum.
更多查看译文
关键词
Cryptocurrencies,Scams,Blockchain,Machine Learning,Fraud Detection
AI 理解论文
溯源树
样例
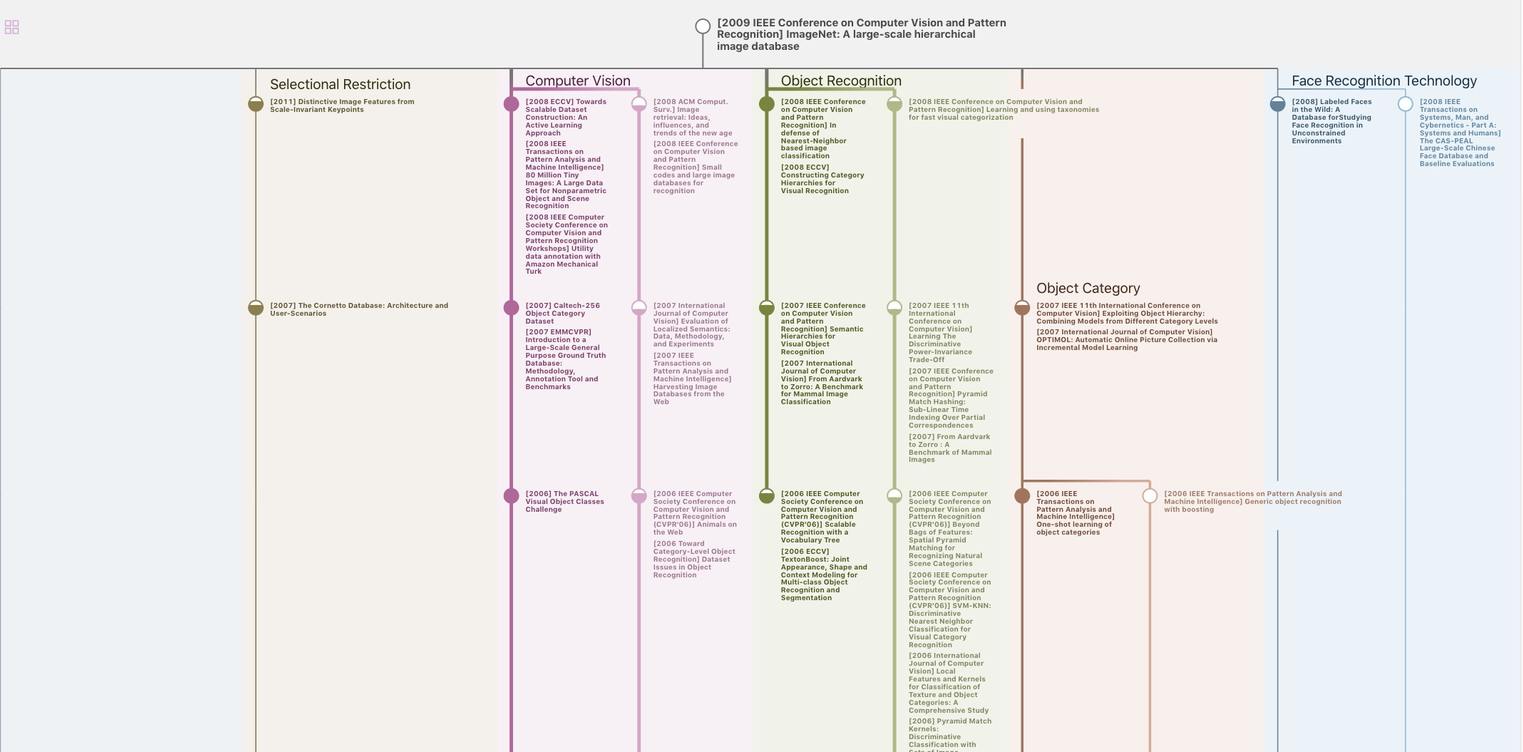
生成溯源树,研究论文发展脉络
Chat Paper
正在生成论文摘要