Cross-domain information fusion for enhanced cell population delineation in single-cell spatial-omics data.
bioRxiv : the preprint server for biology(2024)
摘要
Cell population delineation and identification is an essential step in single-cell and spatial-omics studies. Spatial-omics technologies can simultaneously measure information from three complementary domains related to this task: expression levels of a panel of molecular biomarkers at single-cell resolution, relative positions of cells, and images of tissue sections, but existing computational methods for performing this task on single-cell spatial-omics datasets often relinquish information from one or more domains. The additional reliance on the availability of "atlas" training or reference datasets limits cell type discovery to well-defined but limited cell population labels, thus posing major challenges for using these methods in practice. Successful integration of all three domains presents an opportunity for uncovering cell populations that are functionally stratified by their spatial contexts at cellular and tissue levels: the key motivation for employing spatial-omics technologies in the first place. In this work, we introduce Cell S patio- and N eighborhood-informed A nnotation and P atterning (CellSNAP), a self-supervised computational method that learns a representation vector for each cell in tissue samples measured by spatial-omics technologies at the single-cell or finer resolution. The learned representation vector fuses information about the corresponding cell across all three aforementioned domains. By applying CellSNAP to datasets spanning both spatial proteomic and spatial transcriptomic modalities, and across different tissue types and disease settings, we show that CellSNAP markedly enhances de novo discovery of biologically relevant cell populations at fine granularity, beyond current approaches, by fully integrating cells' molecular profiles with cellular neighborhood and tissue image information.
更多查看译文
AI 理解论文
溯源树
样例
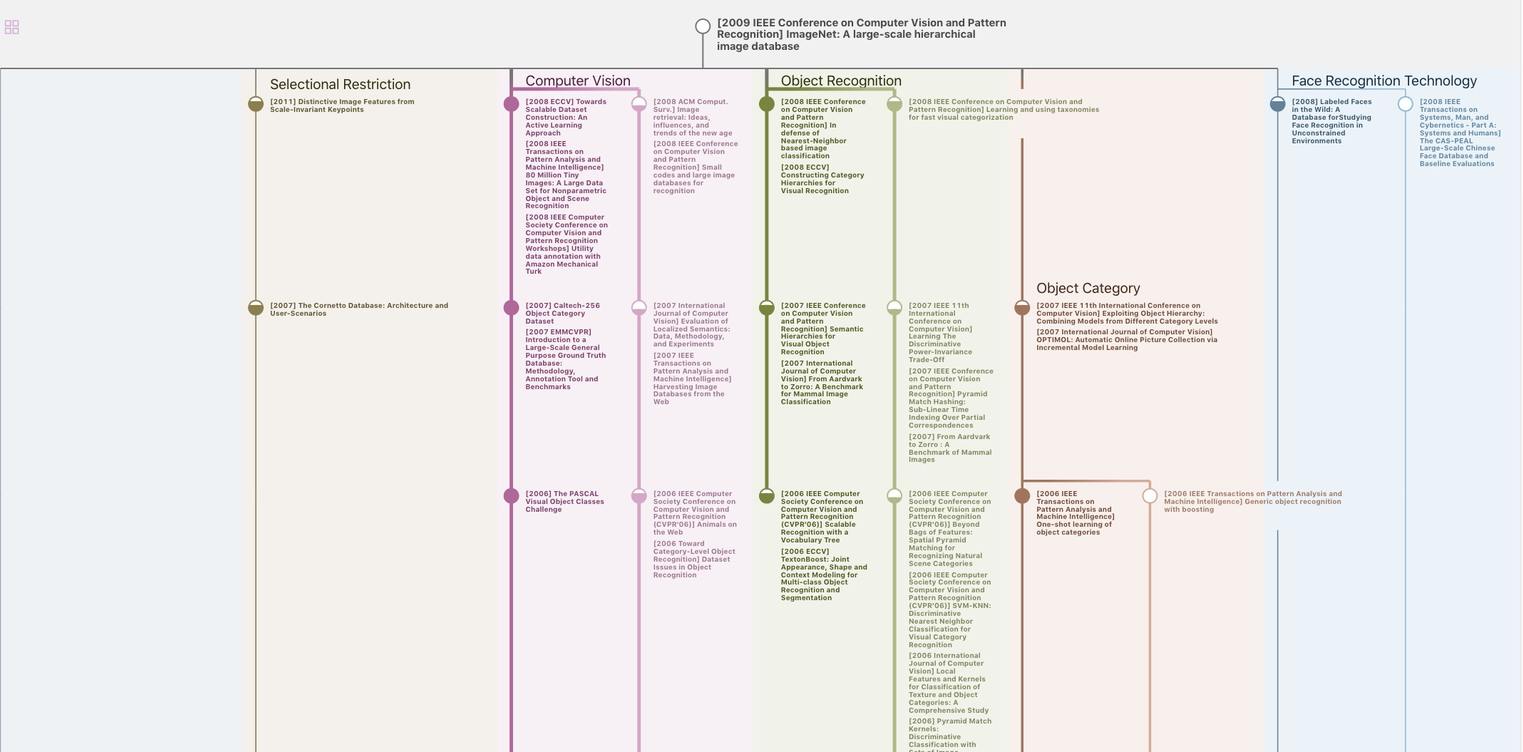
生成溯源树,研究论文发展脉络
Chat Paper
正在生成论文摘要