Abnormal Logical Representation Learning for Intrusion Detection in Industrial Control Systems
IEEE Transactions on Industrial Informatics(2024)
摘要
As security threats to industrial control systems become more prevalent, it is imperative to deploy effective intrusion-detection systems. However, the existing methods are insufficient for addressing contemporary attacks. Rule-based methods are heavily dependent on manual settings, and the covertness of attacks poses challenges to rule effectiveness. Machine and deep learning methods exhibit low interpretability owing to their complex designs, and the semantic gap between the model and the actual operational interpretation limits their applicability. To mitigate these shortcomings, we propose an abnormal logical representation learning (ALRL) intrusion detection method for industrial control systems. ALRL contains a specific lightweight neural network and employs knowledge distillation to achieve high classification ability. More importantly, it can generate effective and concise intrusion detection rules directly from the learned knowledge of the model. The hierarchical model structure and residual connections ensure high interpretability of the rules. Experiments conducted on two publicly available industrial control datasets demonstrate that ALRL can classify attacks with an excellent performance. In addition, the logical rules generated by ALRL can effectively detect all types of attacks and exhibit good interpretability.
更多查看译文
关键词
Control logic,industrial control system (ICS),intrusion detection,model interpretability,rule generation
AI 理解论文
溯源树
样例
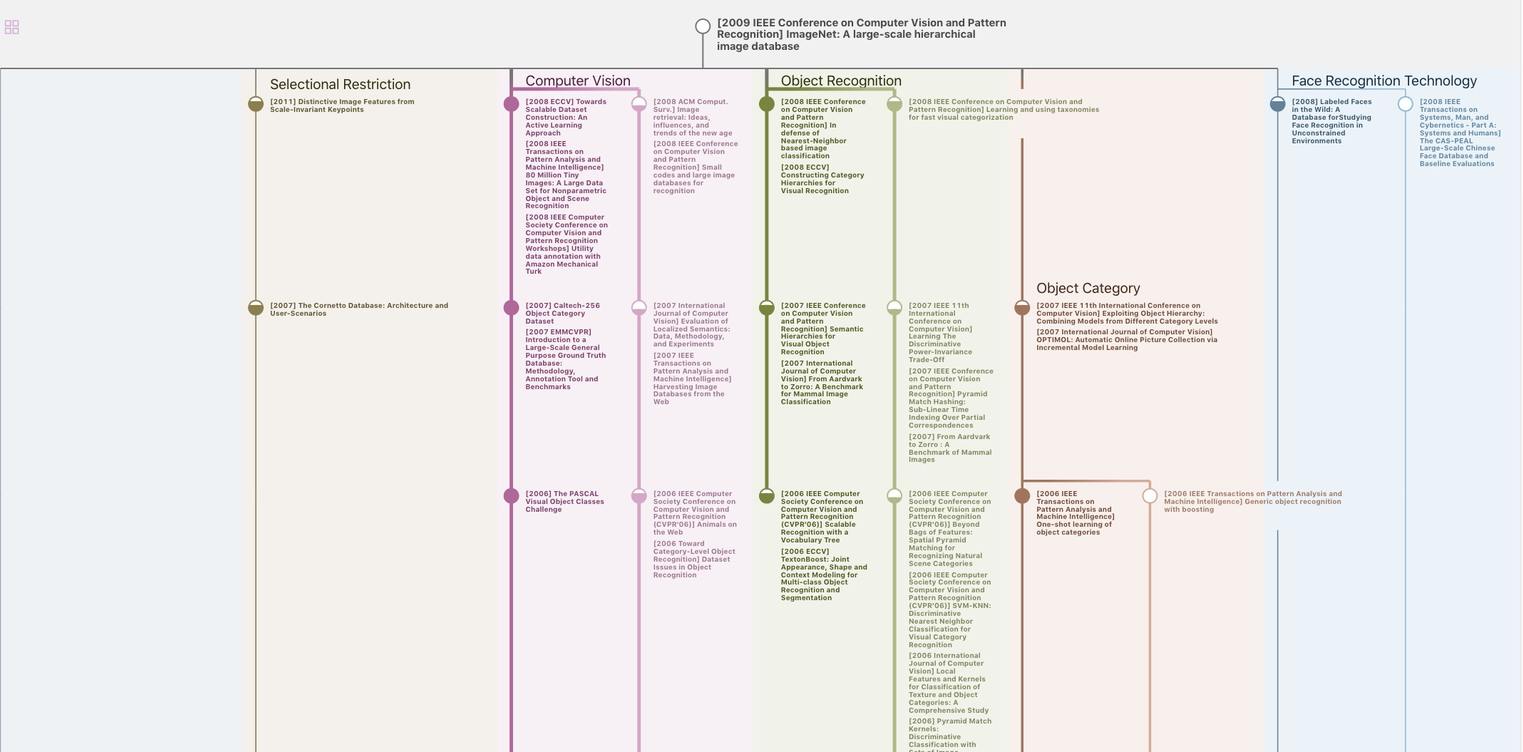
生成溯源树,研究论文发展脉络
Chat Paper
正在生成论文摘要