CNN-LSTM based on attention mechanism for brake pad remaining life prediction
Mechanical Engineering Science(2022)
摘要
In order to predict the remaining service life of brake pads accurately and efficiently, and to achieve intelligent warning, this paper proposes a CNN-LSTM brake pad remaining life prediction model based on an attention mechanism. The model constructs a non-linear relationship between brake pad features such as brake temperature, brake oil pressure and brake speed and brake pad wear data through convolutional neural network (CNN) and long and short term memory network (LSTM), as well as capturing the time dependence that exists in the brake pad wear sequence. The attention mechanism is also introduced to assign different weight values to the features output from multiple historical moments, highlighting the features with high saliency and avoiding the influence of invalid features, so as to improve the prediction effect of the remaining brake pad life. The results show that the proposed CNN-LSTM-Attention model can effectively predict the remaining life of brake pads, with the mean absolute error MAE value of 0.0048, root mean square error RMSE value of 0.0059 and coefficient of determination R2 value of 0.9636; and compared with the BP model, CNN model, LSTM model and CNN-LSTM model, the coefficient of determination R2 values are closest to 1, with an improvement of 8.26%, 5.25%, 3.99% and 1.85% respectively, enabling more effective monitoring and intelligent warning of the remaining brake pad life.
更多查看译文
AI 理解论文
溯源树
样例
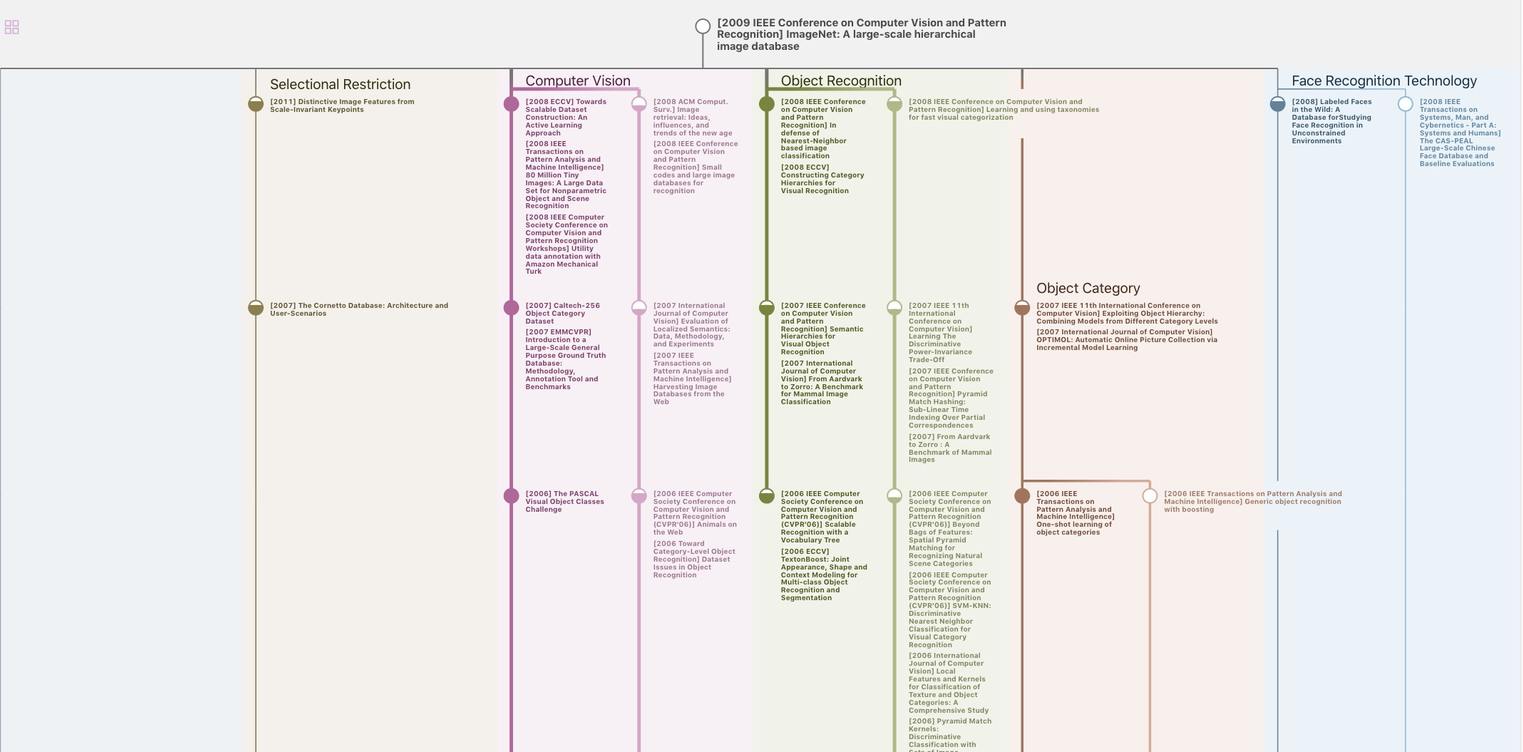
生成溯源树,研究论文发展脉络
Chat Paper
正在生成论文摘要