A Novel Strategy for Differentiating Motor Imagination Brain-Computer Interface Tasks by Fusing EEG and Functional Near-Infrared Spectroscopy Signals
Biomedical Signal Processing and Control(2024)SCI 2区SCI 3区
State Key Laboratory of Reliability and Intelligence of Electrical Equipment | Chinese Acad Med Sci & Peking Union Med Coll
Abstract
The multimodal brain-computer interface (BCI) is an innovative paradigm for human-computer interaction that utilizes both electroencephalography (EEG) and functional near-infrared spectroscopy (fNIRS) signals; therefore, it has received considerable interest. In this study, we concurrently collected EEG and fNIRS data from eighteen healthy participants while they engaged in the mental simulation of performing grasping movements with their left and right hands. During the feature screening session, we assessed the effectiveness of combining the Relief and minimum redundancy maximum relevance (mRMR) algorithms. This algorithm was applied individually to analyze the common spatial pattern (CSP) characteristics of EEG signals across distinct frequency bands as well as the modified CSP (MCSP) attributes of fNIRS signals. Moreover, the enhancement of classification accuracy via feature-level fusion of the two signal types was investigated. The support vector machine (SVM) algorithm was used as the classifier for both training and validation. The results show a significant decrease in the feature count and a substantial enhancement in classification accuracy. Additionally, the highest classification accuracy (88.33 % +/- 5.80 % for EEG + HbO + HbR, P < 0.05) was achieved when utilizing multimodal features, which exceeds that when utilizing EEG alone (84.28 % +/- 7.56 %). Furthermore, the group of participants yielding an enhanced classification accuracy under the multi-modal characteristics constituted the highest percentage among all participants in the case of combined EEG and HbR (88.89 %). The proposed multi-modal information fusion strategy can serve as an effective reference for task recognition in BCI.
MoreTranslated text
Key words
Multimodal brain-computer interface,Motor imagery,Electroencephalography,Functional near-infrared spectroscopy,Feature-level fusion
求助PDF
上传PDF
View via Publisher
AI Read Science
AI Summary
AI Summary is the key point extracted automatically understanding the full text of the paper, including the background, methods, results, conclusions, icons and other key content, so that you can get the outline of the paper at a glance.
Example
Background
Key content
Introduction
Methods
Results
Related work
Fund
Key content
- Pretraining has recently greatly promoted the development of natural language processing (NLP)
- We show that M6 outperforms the baselines in multimodal downstream tasks, and the large M6 with 10 parameters can reach a better performance
- We propose a method called M6 that is able to process information of multiple modalities and perform both single-modal and cross-modal understanding and generation
- The model is scaled to large model with 10 billion parameters with sophisticated deployment, and the 10 -parameter M6-large is the largest pretrained model in Chinese
- Experimental results show that our proposed M6 outperforms the baseline in a number of downstream tasks concerning both single modality and multiple modalities We will continue the pretraining of extremely large models by increasing data to explore the limit of its performance
Upload PDF to Generate Summary
Must-Reading Tree
Example
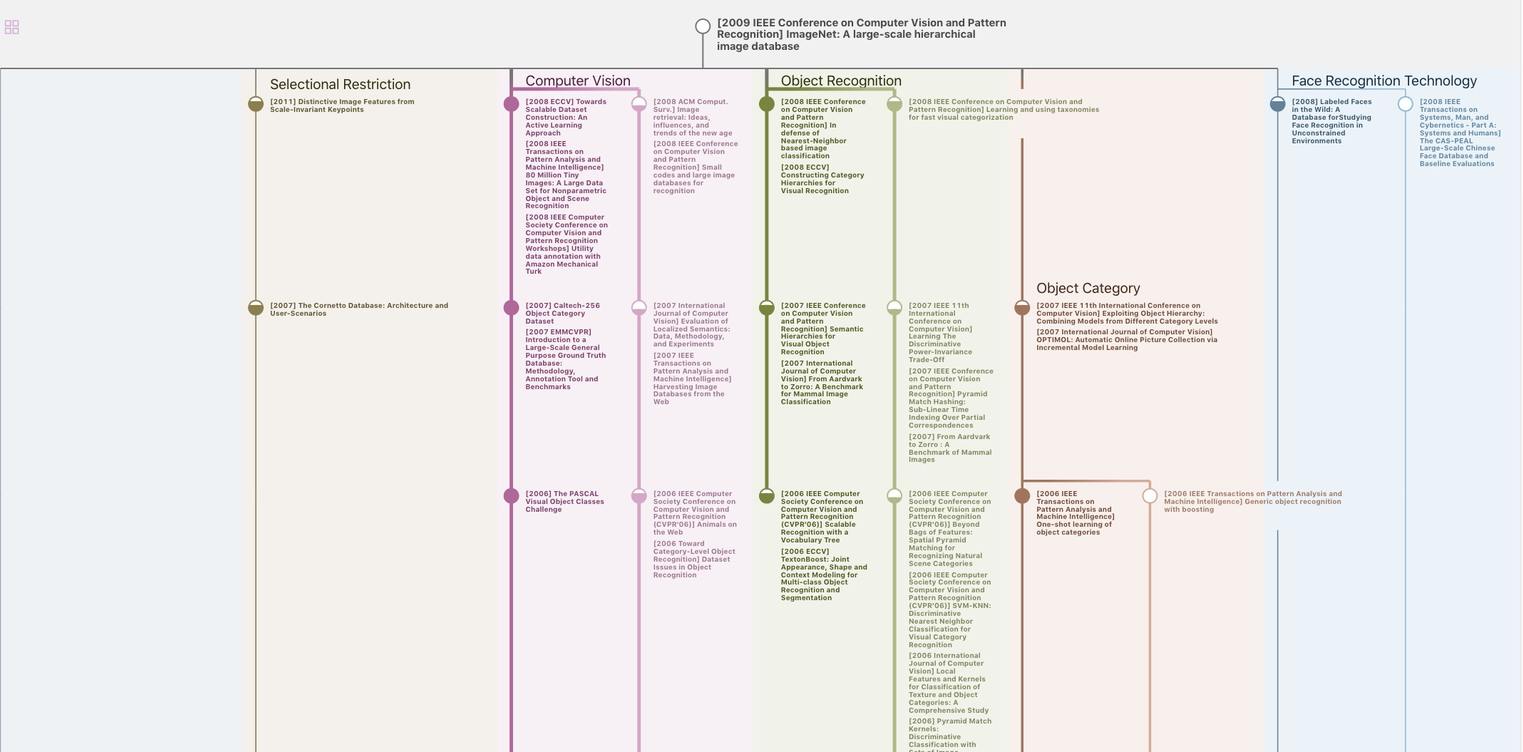
Generate MRT to find the research sequence of this paper
Related Papers
Data Disclaimer
The page data are from open Internet sources, cooperative publishers and automatic analysis results through AI technology. We do not make any commitments and guarantees for the validity, accuracy, correctness, reliability, completeness and timeliness of the page data. If you have any questions, please contact us by email: report@aminer.cn
Chat Paper