Leveraging Limited Experimental Data with Machine Learning: Differentiating a Methyl from an Ethyl Group in the Corey-Bakshi-Shibata Reduction.
Journal of the American Chemical Society(2024)
Abstract
We present a case study on how to improve an existing metal-free catalyst for a particularly difficult reaction, namely, the Corey-Bakshi-Shibata (CBS) reduction of butanone, which constitutes the classic and prototypical challenge of being able to differentiate a methyl from an ethyl group. As there are no known strategies on how to address this challenge, we leveraged the power of machine learning by constructing a realistic (for a typical laboratory) small, albeit high-quality, data set of about 100 reactions (run in triplicate) that we used to train a model in combination with a key-intermediate graph (of substrate and catalyst) to predict the differences in Gibbs activation energies Delta Delta G double dagger of the enantiomeric reaction paths. With the help of this model, we were able to select and subsequently screen a small selection of catalysts and increase the selectivity for the CBS reduction of butanone to 80% enantiomeric excess (ee), the highest possible value achieved to date for this substrate with a metal-free catalyst, thereby also exceeding the best available enzymatic systems (64% ee) and the selectivity with Corey's original catalyst (60% ee). This translates into a >50% improvement in relative Delta G double dagger from 0.9 to 1.4 kcal mol(-1). We underscore the transformative potential of machine learning in accelerating catalyst design because we rely on a manageable small data set and a key-intermediate graph representing a combination of catalyst and substrate graphs in lieu of a transition-state model. Our results highlight the synergy of synthetic chemistry and data-centric approaches and provide a blueprint for future catalyst optimization.
MoreTranslated text
AI Read Science
Must-Reading Tree
Example
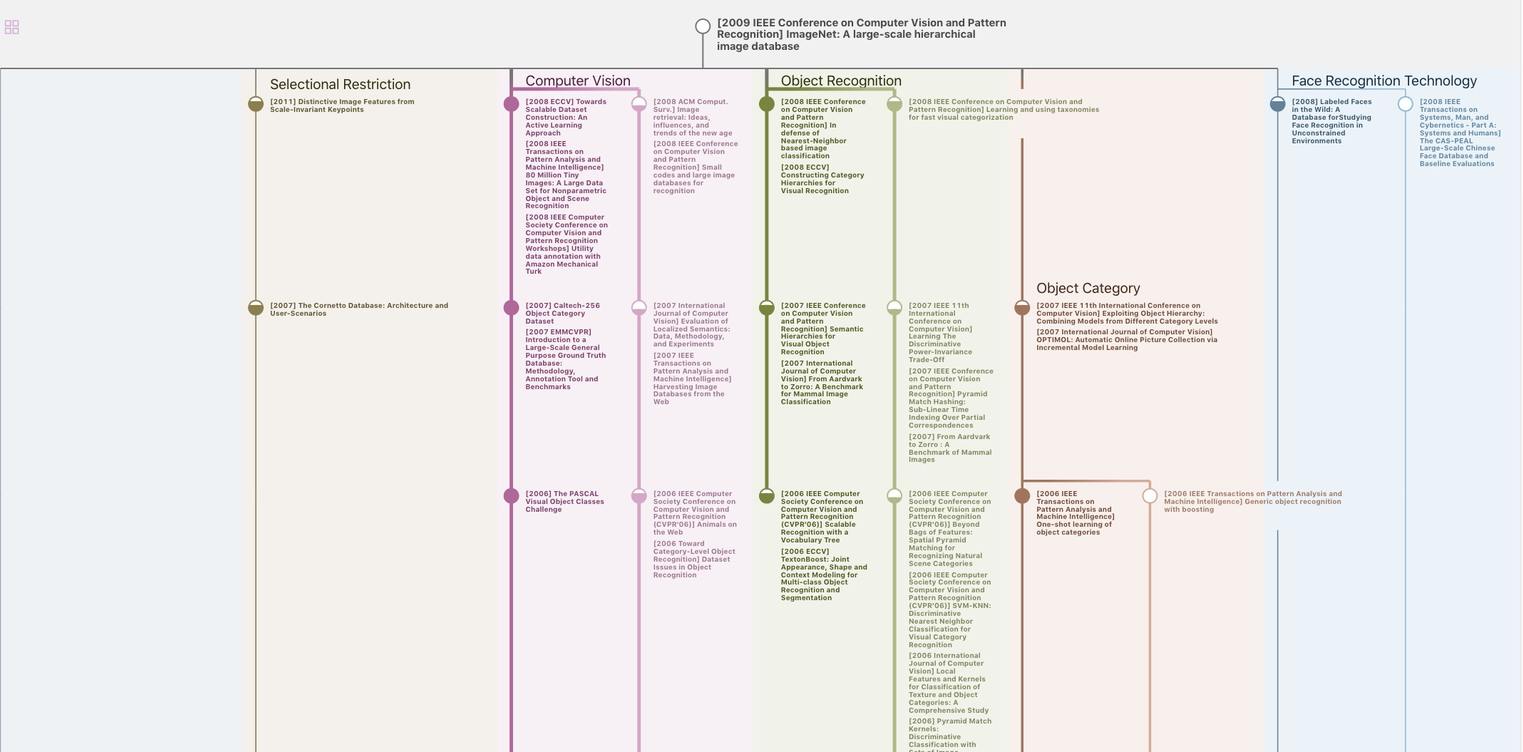
Generate MRT to find the research sequence of this paper
Chat Paper
Summary is being generated by the instructions you defined