Multi Analyte Concentration Analysis of Marine Samples Through Regression Based Machine Learning
crossref(2024)
摘要
Marine systems are incredibly chemically complex. An understanding of the chemical compounds that make up the chemical diversity in these samples is critical to understanding ecological and ocean health metrics. Using Raman spectroscopy in tandem with machine learning combines a low-cost highly transportable analytical technique with a powerful and rapid computational approach that can aid in marine analysis. Here we use Raman spectroscopy and machine learning to identify mM concentrations of three chemically relevant compounds in three distinct classes in a complex aqueous matrix. Saccharides are represented by glucose, fatty acids by butyric acid, and proteins are represented by amino acid proxy through glycine. Eight classical machine learning models (gradient boosted regressors, random forests, histogram gradient boosted regressors, decision trees, k nearest neighbors, support vector regression, multilayer perceptrons, and multivariate linear regression) were tested for their accuracy in identifying the concentrations of glycine, glucose, and butyric acid in marine samples, which were benchmarked through a mass spectrometric method. Support vector regression was able to best identify all three concentrations of glycine, butyric acid, and glucose. Butyric acid was similarly well described through gradient boosted regression and histogram gradient boosted regression. The described spectroscopy and machine learning methodology has the potential to significantly advance rapid field analysis of marine samples.
更多查看译文
AI 理解论文
溯源树
样例
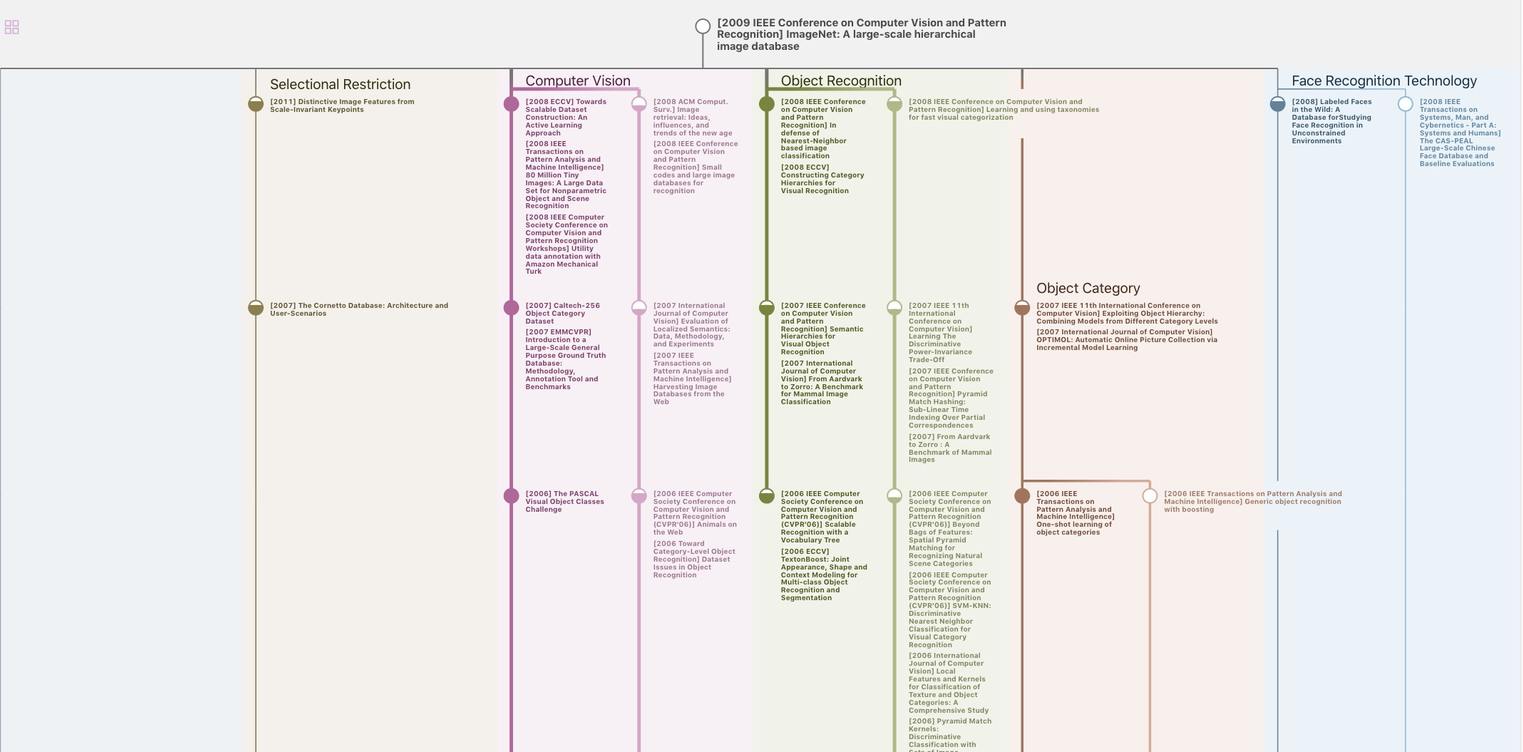
生成溯源树,研究论文发展脉络
Chat Paper
正在生成论文摘要