SGCLDGA: Unveiling Drug-Gene Associations Through Simple Graph Contrastive Learning
Briefings in bioinformatics(2024)
摘要
Drug repurposing offers a viable strategy for discovering new drugs and therapeutic targets through the analysis of drug-gene interactions. However, traditional experimental methods are plagued by their costliness and inefficiency. Despite graph convolutional network (GCN)-based models' state-of-the-art performance in prediction, their reliance on supervised learning makes them vulnerable to data sparsity, a common challenge in drug discovery, further complicating model development. In this study, we propose SGCLDGA, a novel computational model leveraging graph neural networks and contrastive learning to predict unknown drug-gene associations. SGCLDGA employs GCNs to extract vector representations of drugs and genes from the original bipartite graph. Subsequently, singular value decomposition (SVD) is employed to enhance the graph and generate multiple views. The model performs contrastive learning across these views, optimizing vector representations through a contrastive loss function to better distinguish positive and negative samples. The final step involves utilizing inner product calculations to determine association scores between drugs and genes. Experimental results on the DGIdb4.0 dataset demonstrate SGCLDGA's superior performance compared with six state-of-the-art methods. Ablation studies and case analyses validate the significance of contrastive learning and SVD, highlighting SGCLDGA's potential in discovering new drug-gene associations. The code and dataset for SGCLDGA are freely available at https://github.com/one-melon/SGCLDGA.
更多查看译文
关键词
drug-gene association,singular value decomposition,contrastive learning,graph neural network
AI 理解论文
溯源树
样例
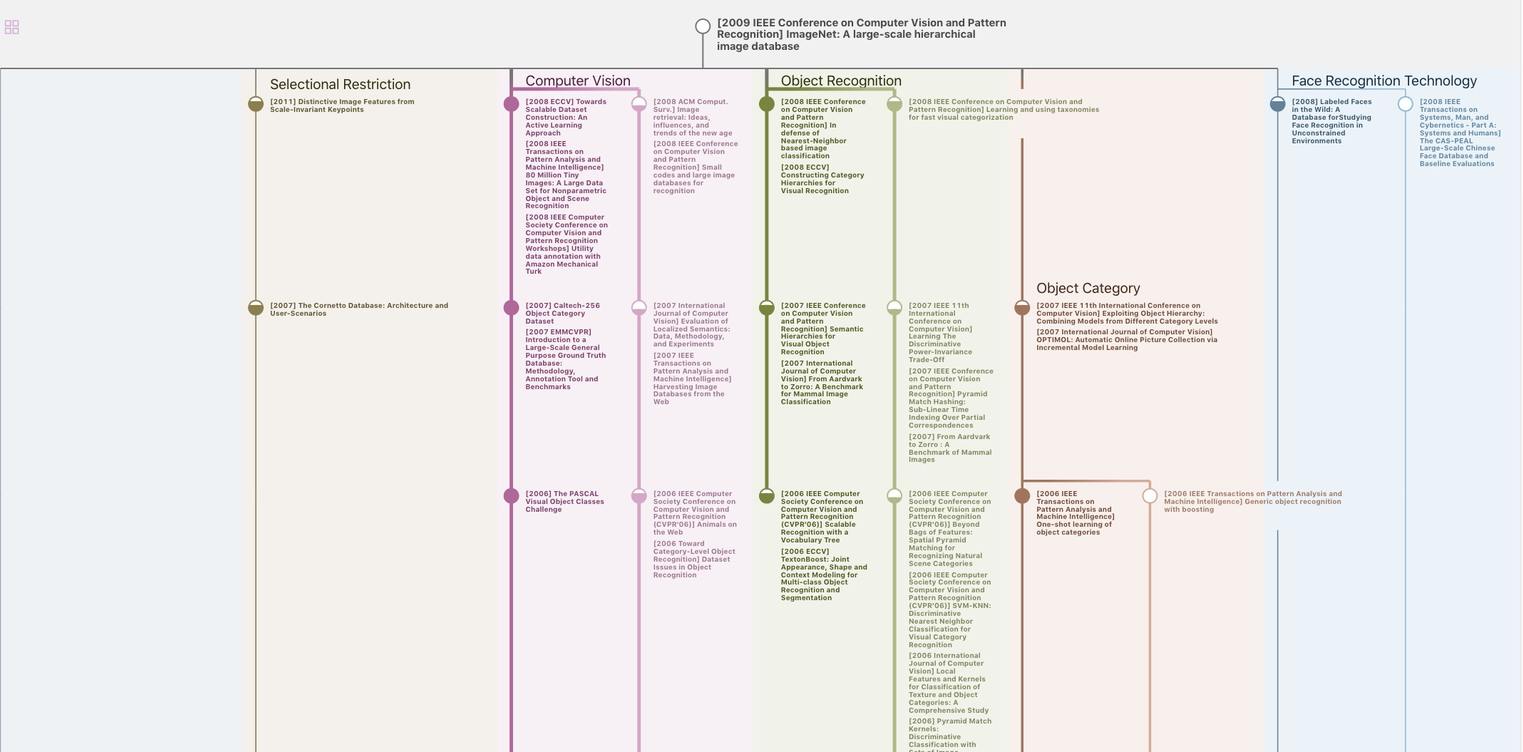
生成溯源树,研究论文发展脉络
Chat Paper
正在生成论文摘要