MFT-GAN: A Multiscale Feature-guided Transformer Network for Unsupervised Hyperspectral Pansharpening
IEEE Transactions on Geoscience and Remote Sensing(2024)
摘要
Unsupervised learning, learning data distributions without needing labeled samples, is a particularly promising approach for solving the challenging task of hyperspectral pansharpening. Inspired by the above, we introduce an innovative generative adversarial network framework (named MFT-GAN) that incorporates a transformer network and multiscale interaction technology. Specifically, MFT-GAN is constructed of one generator and two discriminators. The generator is composed of a multiscale feature guidance branch (MFGB) and a feature interaction fusion branch (FIFB). The former aims at extracting spectral and spatial information branches from low-resolution hyperspectral (LRHS) and panchromatic (PAN) images by convolutional sampling operations, respectively. The latter accomplishes the interaction of spectral and spatial information at different scales through multiscale interaction technology. Then, the multiscale interaction results are fused by level-by-level convolutional sampling and pixel summation operations to generate high-resolution hyperspectral (HRHS) images. The discriminators are composed of a spectral transformer discriminator and a spatial transformer discriminator, designed to maintain structural and parametric balance and learn long-range spectral and spatial correlation relationships. In addition, hybrid loss functions are employed to complete the adversarial training of the MFT-GAN to improve its performance. The experiments on simulated and real datasets further demonstrated the effectiveness of the proposed MFT-GAN method.
更多查看译文
关键词
Unsupervised hyperspectral pansharpening,generative adversarial network,multiscale feature guidance,feature interaction fusion,spectral and spatial transformer
AI 理解论文
溯源树
样例
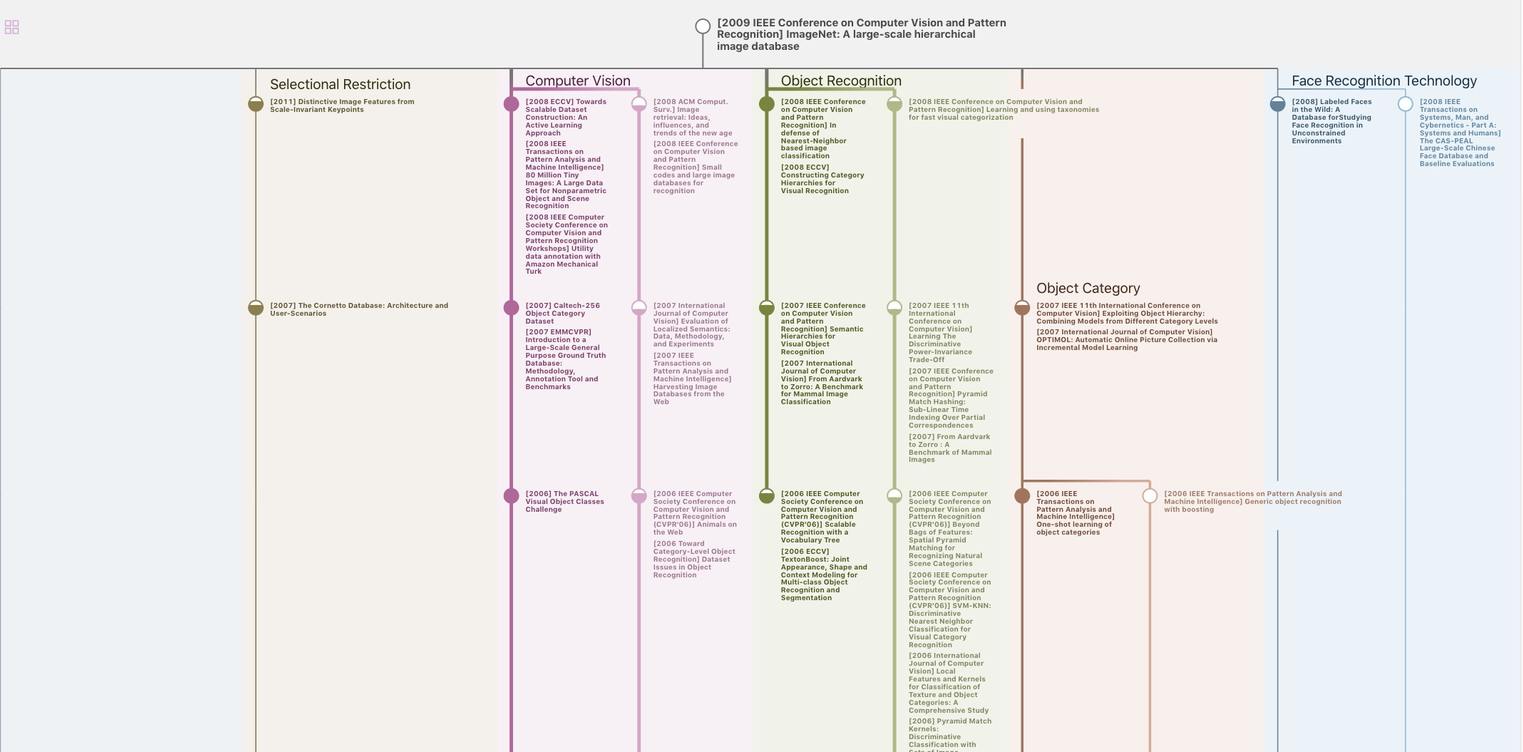
生成溯源树,研究论文发展脉络
Chat Paper
正在生成论文摘要