Automated Data Augmentation for Audio Classification
IEEE-ACM TRANSACTIONS ON AUDIO SPEECH AND LANGUAGE PROCESSING(2024)
摘要
Audio classification is a challenging task that requires categorizing audio data based on its content or characteristics. Existing approaches for audio classification rely either on supervised learning or fine-tuning based on self-supervised learning, both of which require manually labeled data. However, manually labeling audio datasets is a time-consuming and expensive process that limits the dataset's size. Moreover, the diversity of sound categories and class imbalances can further impede classification performance. To overcome these challenges, researchers have proposed various audio data augmentation methods. However, most of these methods focus less on augmentations combination and design and rely solely on waveform-based or spectrogrambased approaches. This paper presents an Automated Audio Augmentation (AAA) method for audio classification, which generates learnable and composable augmentation policies suitable for the audio classification task and can be employed in a plug-andplay manner. This method leverages both waveform-level and spectrogram-level augmentation, and a Bayesian optimization algorithm is proposed to search for composed augmentation policies. To the best of our knowledge, this is the first attempt to propose an automatic data augmentation method for audio classification tasks. Through large-scale empirical studies, we demonstrate that the proposed method outperforms previous competitive methods by a significant margin. We improve the average performance of multiple datasets by 6.421% and by 7.33% on few-shot scenarios, respectively.
更多查看译文
关键词
Audio Classification,Automated Augmentation,Audio Data Augmentation
AI 理解论文
溯源树
样例
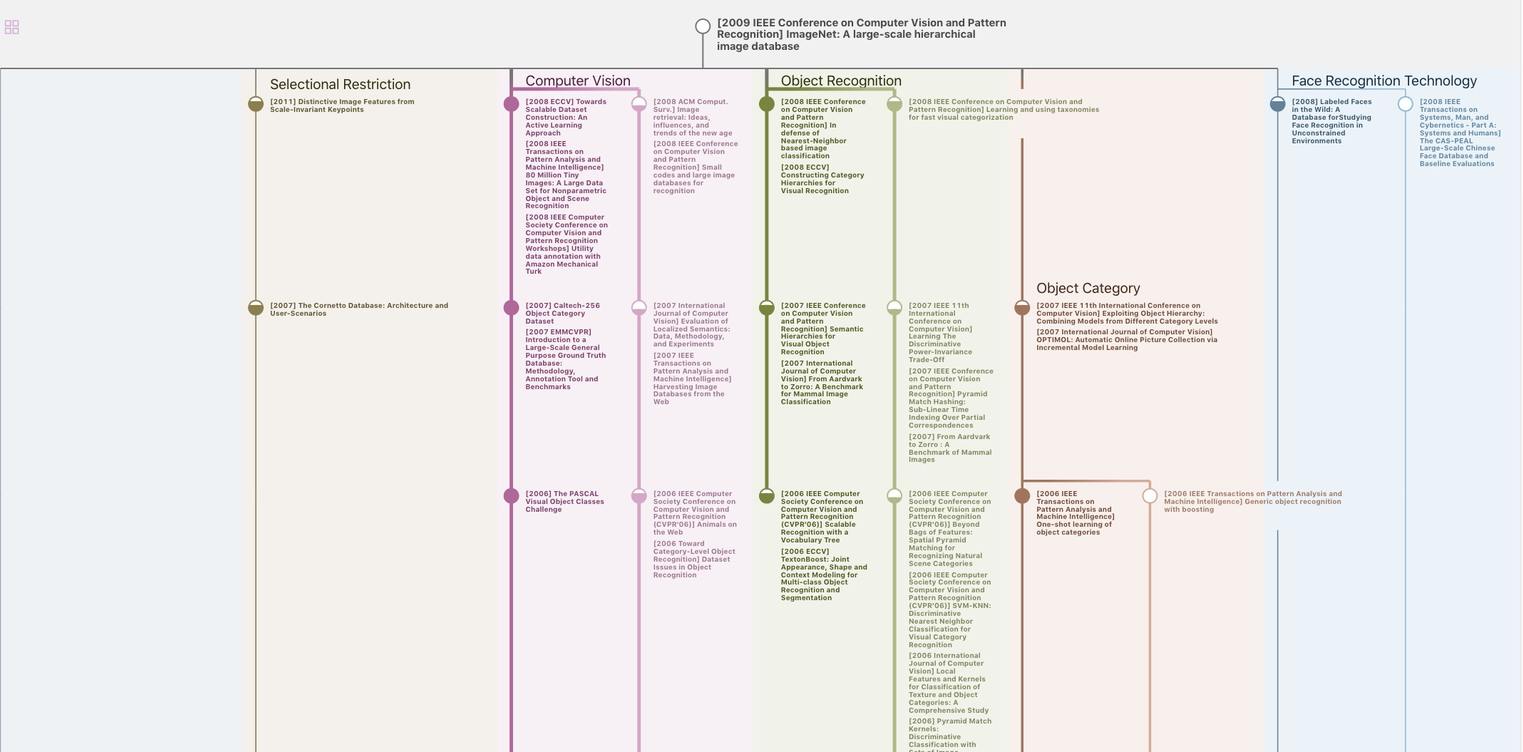
生成溯源树,研究论文发展脉络
Chat Paper
正在生成论文摘要