Evaluating Text-to-Speech Synthesis from a Large Discrete Token-based Speech Language Model
International Conference on Computational Linguistics(2024)
Abstract
Recent advances in generative language modeling applied to discrete speech
tokens presented a new avenue for text-to-speech (TTS) synthesis. These speech
language models (SLMs), similarly to their textual counterparts, are scalable,
probabilistic, and context-aware. While they can produce diverse and natural
outputs, they sometimes face issues such as unintelligibility and the inclusion
of non-speech noises or hallucination. As the adoption of this innovative
paradigm in speech synthesis increases, there is a clear need for an in-depth
evaluation of its capabilities and limitations. In this paper, we evaluate TTS
from a discrete token-based SLM, through both automatic metrics and listening
tests. We examine five key dimensions: speaking style, intelligibility, speaker
consistency, prosodic variation, spontaneous behaviour. Our results highlight
the model's strength in generating varied prosody and spontaneous outputs. It
is also rated higher in naturalness and context appropriateness in listening
tests compared to a conventional TTS. However, the model's performance in
intelligibility and speaker consistency lags behind traditional TTS.
Additionally, we show that increasing the scale of SLMs offers a modest boost
in robustness. Our findings aim to serve as a benchmark for future advancements
in generative SLMs for speech synthesis.
MoreTranslated text
PDF
View via Publisher
AI Read Science
AI Summary
AI Summary is the key point extracted automatically understanding the full text of the paper, including the background, methods, results, conclusions, icons and other key content, so that you can get the outline of the paper at a glance.
Example
Background
Key content
Introduction
Methods
Results
Related work
Fund
Key content
- Pretraining has recently greatly promoted the development of natural language processing (NLP)
- We show that M6 outperforms the baselines in multimodal downstream tasks, and the large M6 with 10 parameters can reach a better performance
- We propose a method called M6 that is able to process information of multiple modalities and perform both single-modal and cross-modal understanding and generation
- The model is scaled to large model with 10 billion parameters with sophisticated deployment, and the 10 -parameter M6-large is the largest pretrained model in Chinese
- Experimental results show that our proposed M6 outperforms the baseline in a number of downstream tasks concerning both single modality and multiple modalities We will continue the pretraining of extremely large models by increasing data to explore the limit of its performance
Try using models to generate summary,it takes about 60s
Must-Reading Tree
Example
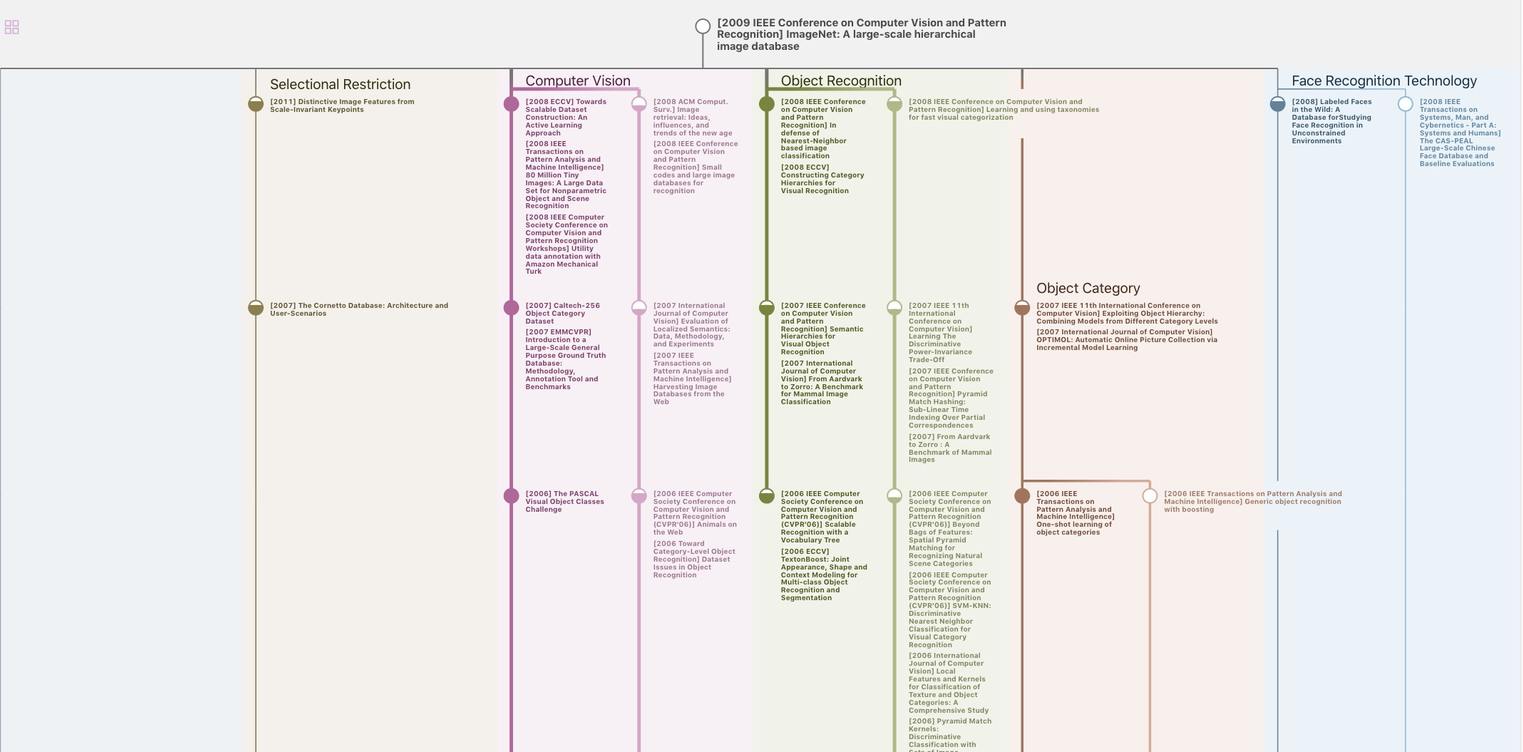
Generate MRT to find the research sequence of this paper
Related Papers
Data Disclaimer
The page data are from open Internet sources, cooperative publishers and automatic analysis results through AI technology. We do not make any commitments and guarantees for the validity, accuracy, correctness, reliability, completeness and timeliness of the page data. If you have any questions, please contact us by email: report@aminer.cn
Chat Paper
去 AI 文献库 对话