Scaling convolutional neural networks achieves expert-level seizure detection in neonatal EEG
CoRR(2024)
摘要
Background: Neonatal seizures are a neurological emergency that require
urgent treatment. They are hard to diagnose clinically and can go undetected if
EEG monitoring is unavailable. EEG interpretation requires specialised
expertise which is not widely available. Algorithms to detect EEG seizures can
address this limitation but have yet to reach widespread clinical adoption.
Methods: Retrospective EEG data from 332 neonates was used to develop and
validate a seizure-detection model. The model was trained and tested with a
development dataset (n=202) that was annotated with over 12k seizure events
on a per-channel basis. This dataset was used to develop a convolutional neural
network (CNN) using a modern architecture and training methods. The final model
was then validated on two independent multi-reviewer datasets (n=51 and
n=79).
Results: Increasing dataset and model size improved model performance:
Matthews correlation coefficient (MCC) and Pearson's correlation (r)
increased by up to 50
50k hours of annotated single-channel EEG was used for training a model with 21
million parameters. State-of-the-art was achieved on an open-access dataset
(MCC=0.764, r=0.824, and AUC=0.982). The CNN attains expert-level performance
on both held-out validation sets, with no significant difference in inter-rater
agreement among the experts and among experts and algorithm (Δκ <
-0.095, p>0.05).
Conclusion: With orders of magnitude increases in data and model scale we
have produced a new state-of-the-art model for neonatal seizure detection.
Expert-level equivalence on completely unseen data, a first in this field,
provides a strong indication that the model is ready for further clinical
validation.
更多查看译文
AI 理解论文
溯源树
样例
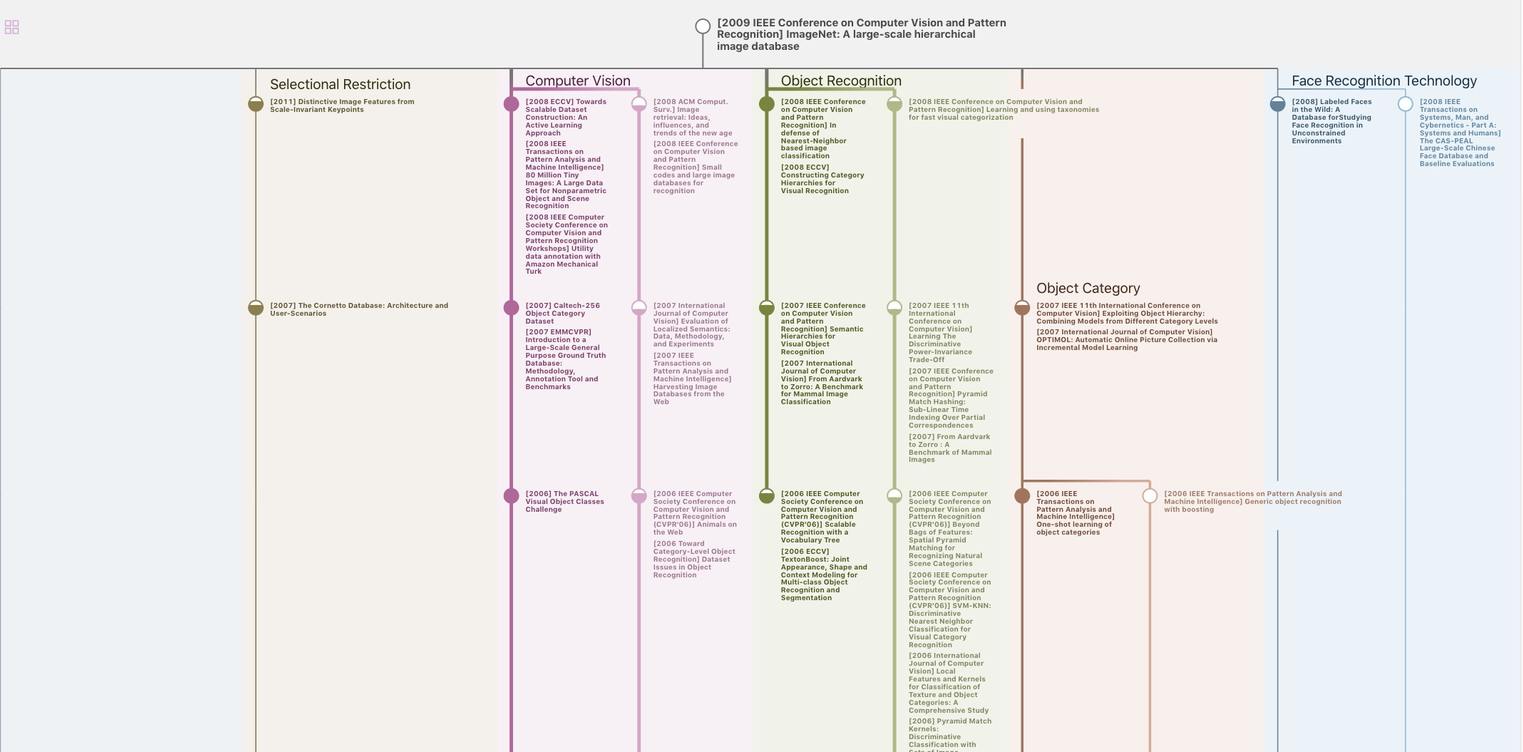
生成溯源树,研究论文发展脉络
Chat Paper
正在生成论文摘要