Beyond Static Calibration: the Impact of User Preference Dynamics on Calibrated Recommendation
ADJUNCT PROCEEDINGS OF THE 32ND ACM CONFERENCE ON USER MODELING, ADAPTATION AND PERSONALIZATION, UMAP 2024(2024)
摘要
Calibration in recommender systems is an important performance criterion thatensures consistency between the distribution of user preference categories andthat of recommendations generated by the system. Standard methods formitigating miscalibration typically assume that user preference profiles arestatic, and they measure calibration relative to the full history of user'sinteractions, including possibly outdated and stale preference categories. Weconjecture that this approach can lead to recommendations that, while appearingcalibrated, in fact, distort users' true preferences. In this paper, we conducta preliminary investigation of recommendation calibration at a more granularlevel, taking into account evolving user preferences. By analyzing differentlysized training time windows from the most recent interactions to the oldest, weidentify the most relevant segment of user's preferences that optimizes thecalibration metric. We perform an exploratory analysis with datasets fromdifferent domains with distinctive user-interaction characteristics. Wedemonstrate how the evolving nature of user preferences affects recommendationcalibration, and how this effect is manifested differently depending on thecharacteristics of the data in a given domain. Datasets, codes, and moredetailed experimental results are available at:https://github.com/nicolelin13/DynamicCalibrationUMAP.
更多查看译文
关键词
Recommender systems,calibration,preference dynamics
AI 理解论文
溯源树
样例
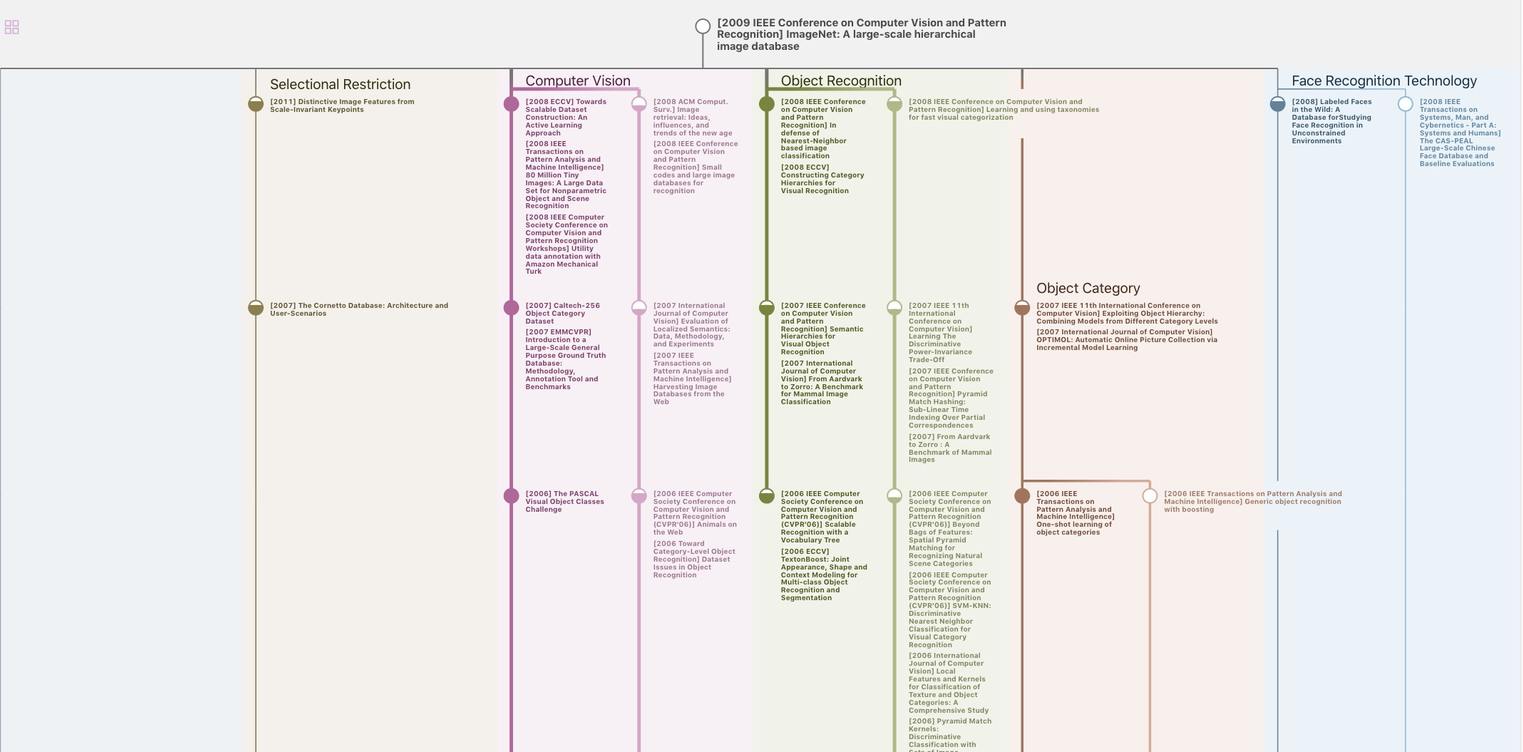
生成溯源树,研究论文发展脉络
Chat Paper
正在生成论文摘要