Timeline-based Sentence Decomposition with In-Context Learning for Temporal Fact Extraction
Annual Meeting of the Association for Computational Linguistics(2024)
Abstract
Facts extraction is pivotal for constructing knowledge graphs. Recently, theincreasing demand for temporal facts in downstream tasks has led to theemergence of the task of temporal fact extraction. In this paper, wespecifically address the extraction of temporal facts from natural languagetext. Previous studies fail to handle the challenge of establishingtime-to-fact correspondences in complex sentences. To overcome this hurdle, wepropose a timeline-based sentence decomposition strategy using large languagemodels (LLMs) with in-context learning, ensuring a fine-grained understandingof the timeline associated with various facts. In addition, we evaluate theperformance of LLMs for direct temporal fact extraction and get unsatisfactoryresults. To this end, we introduce TSDRE, a method that incorporates thedecomposition capabilities of LLMs into the traditional fine-tuning of smallerpre-trained language models (PLMs). To support the evaluation, we constructComplexTRED, a complex temporal fact extraction dataset. Our experiments showthat TSDRE achieves state-of-the-art results on both HyperRED-Temporal andComplexTRED datasets.
MoreTranslated text
PDF
View via Publisher
AI Read Science
Must-Reading Tree
Example
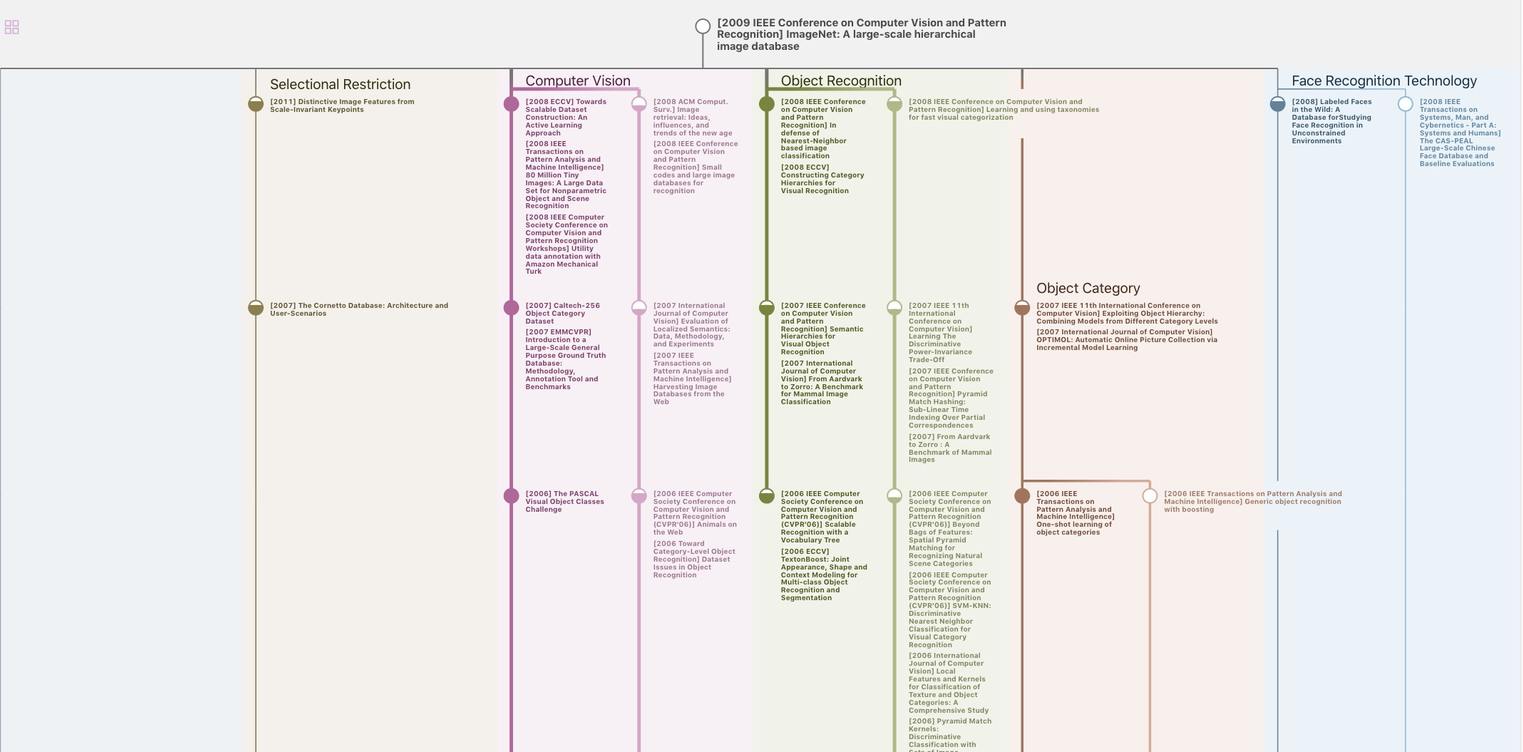
Generate MRT to find the research sequence of this paper
Data Disclaimer
The page data are from open Internet sources, cooperative publishers and automatic analysis results through AI technology. We do not make any commitments and guarantees for the validity, accuracy, correctness, reliability, completeness and timeliness of the page data. If you have any questions, please contact us by email: report@aminer.cn
Chat Paper
去 AI 文献库 对话