Rheological behavior of 3D printed concrete: influential factors and printability prediction scheme
Journal of Building Engineering(2024)
摘要
The rheological properties of cementitious materials play a crucial role in determining the printability for extrusion-based 3D concrete printing. This study develops data-driven machine learning (ML) models to predict two key rheological parameters - plastic viscosity (PV) and yield stress (YS) of 3D printable cementitious composites based on the mixture composition and time after water addition. A systematic experimental study is conducted by varying the contents of cement, fly ash, silica fume, sulfoaluminate cement, superplasticizer, and water-to-binder ratio, and time after water addition. The measured rheological data is used to construct a database for training predictive models including linear regression, support vector regression, random forest, extreme gradient boosting, and multi-layer perceptron neural network. The extreme gradient boosting model achieves the highest prediction accuracy with low root mean square error and all coefficients of determination exceeding 0.9 for both plastic viscosity and yield stress. Importance analysis identifies the most influential parameters affecting the rheological properties. A printability classification scheme is proposed using the model predictions by defining a printable zone of PV and YS. The data-driven framework is validated to effectively predict printability of new mixtures without trial-and-error. This study demonstrates the potential of ML models to accelerate the design and optimization of 3D printable cementitious materials.
更多查看译文
关键词
3D printed concrete,Machine learning,Model predictions,Rheological properties,Printability
AI 理解论文
溯源树
样例
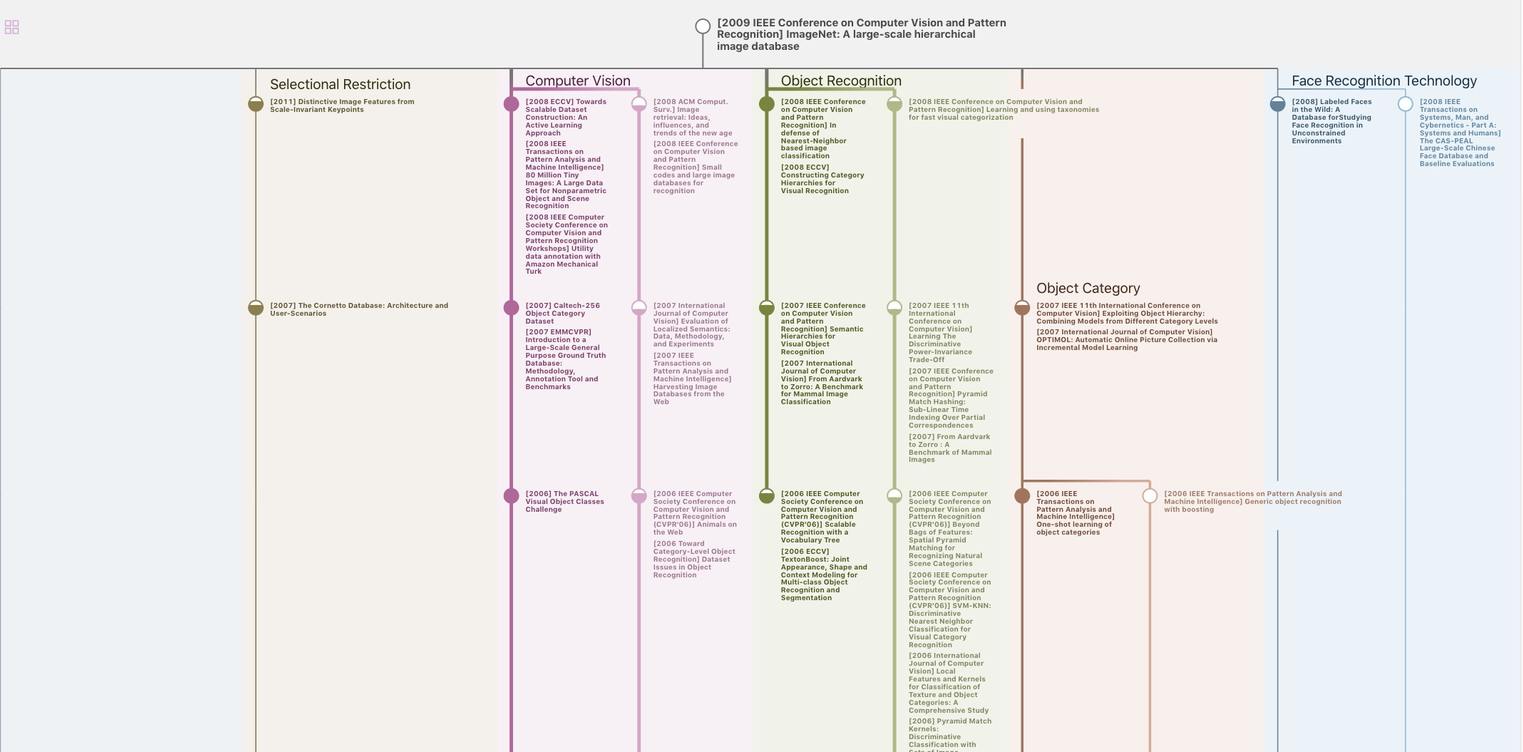
生成溯源树,研究论文发展脉络
Chat Paper
正在生成论文摘要