A novel approach for estimating fractional cover of crops by correcting angular effect using radiative transfer models and UAV multi-angular spectral data
Computers and Electronics in Agriculture(2024)
摘要
Fractional vegetation cover (FVC) plays an important role in spectral unmixing, crop growth monitoring, crop light interception calculation, and yield estimation. However, the spectral reflectance would change with view zenith angles (VZAs), and retrieved FVC is also affected by VZAs. Therefore, in this paper, the observed multi-angular (±45°, ±30°, 0°) spectral datasets with different crops (wheat and rice), along with simulated spectral dataset, were used to explore method of correcting the influence of angle effect in FVC retrieval. Firstly, a simulated dataset of multi-angular hyperspectral data and directional FVC were constructed using the PROSAIL model, and a single-angular FVC retrieval model (Sin-FVC) was established based on the Gaussian process regression (GPR) algorithm. Then, the single-angular FVC (i.e., FVCθ) was converted into vertical FVC (FVCθv) based on the Beer-Lambert law. Finally, a Multi-FVC model was developed, which summed outputs of the Sin-FVC model (FVC0°, FVCθv) according to their respective weights (i.e., FVCcorrect), to correct the angle effect. Using the pixel bisection model (FVCVI) as a comparison, different FVC retrieval models (Sin-FVC, Multi-FVC, and FVCVI) were compared and evaluated in terms of FVC retrieval accuracy, ability in weakening soil background, and LNC retrieval accuracy after spectral decomposition using different retrieved FVC (FVC0°, FVC-30°v, FVC-45°v, FVCcorrect). The results showed that FVCcorrect had the highest FVC retrieval accuracy (RRMSE = 8.5 %) compared with FVC retrieved from other models (FVCVI, FVC0°, FVC-30°v and FVC-45°v). Meanwhile, when using the reflectance at NIR band in the black soil background as the baseline, after decoupling mixing spectra based on FVCcorrect, the relative offset (i.e., ROcorrect) of each vegetation index (NDVI, EVI, SAVI, OSAVI) was minimum (least-ROcorrect = 0.42 %). That is, the soil background was effectively suppressed by FVCcorrect spectral decomposition, along with the highest LNC retrieval accuracy, with an RRMSE of 14.2 %.
更多查看译文
关键词
Multi-FVC model,Multi-angle remote sensing,Soil background, PROSAIL,Gaussian process regression
AI 理解论文
溯源树
样例
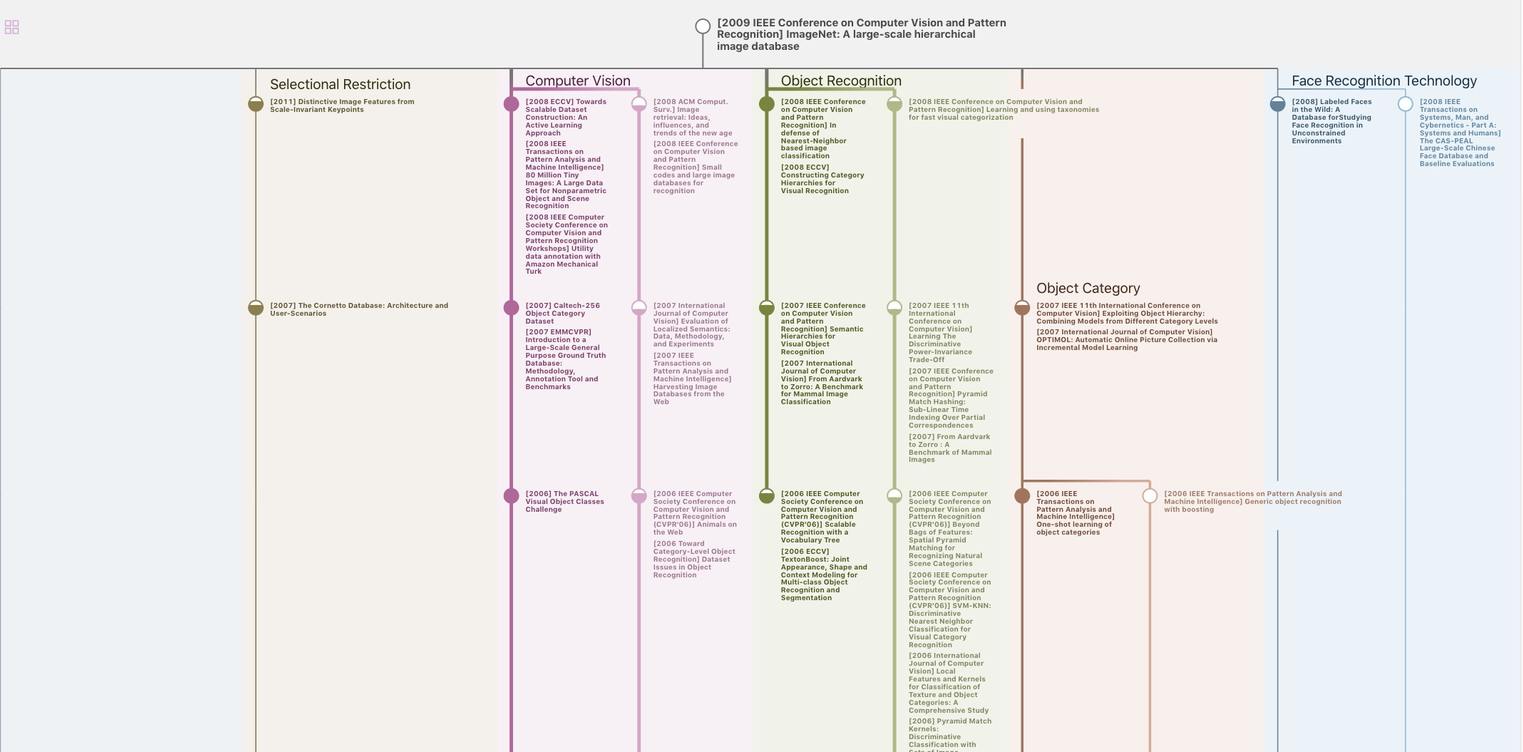
生成溯源树,研究论文发展脉络
Chat Paper
正在生成论文摘要