Using natural language processing in emergency medicine health service research: A systematic review and meta-analysis.
Academic emergency medicine : official journal of the Society for Academic Emergency Medicine(2024)
摘要
OBJECTIVES:Natural language processing (NLP) represents one of the adjunct technologies within artificial intelligence and machine learning, creating structure out of unstructured data. This study aims to assess the performance of employing NLP to identify and categorize unstructured data within the emergency medicine (EM) setting.
METHODS:We systematically searched publications related to EM research and NLP across databases including MEDLINE, Embase, Scopus, CENTRAL, and ProQuest Dissertations & Theses Global. Independent reviewers screened, reviewed, and evaluated article quality and bias. NLP usage was categorized into syndromic surveillance, radiologic interpretation, and identification of specific diseases/events/syndromes, with respective sensitivity analysis reported. Performance metrics for NLP usage were calculated and the overall area under the summary of receiver operating characteristic curve (SROC) was determined.
RESULTS:A total of 27 studies underwent meta-analysis. Findings indicated an overall mean sensitivity (recall) of 82%-87%, specificity of 95%, with the area under the SROC at 0.96 (95% CI 0.94-0.98). Optimal performance using NLP was observed in radiologic interpretation, demonstrating an overall mean sensitivity of 93% and specificity of 96%.
CONCLUSIONS:Our analysis revealed a generally favorable performance accuracy in using NLP within EM research, particularly in the realm of radiologic interpretation. Consequently, we advocate for the adoption of NLP-based research to augment EM health care management.
更多查看译文
AI 理解论文
溯源树
样例
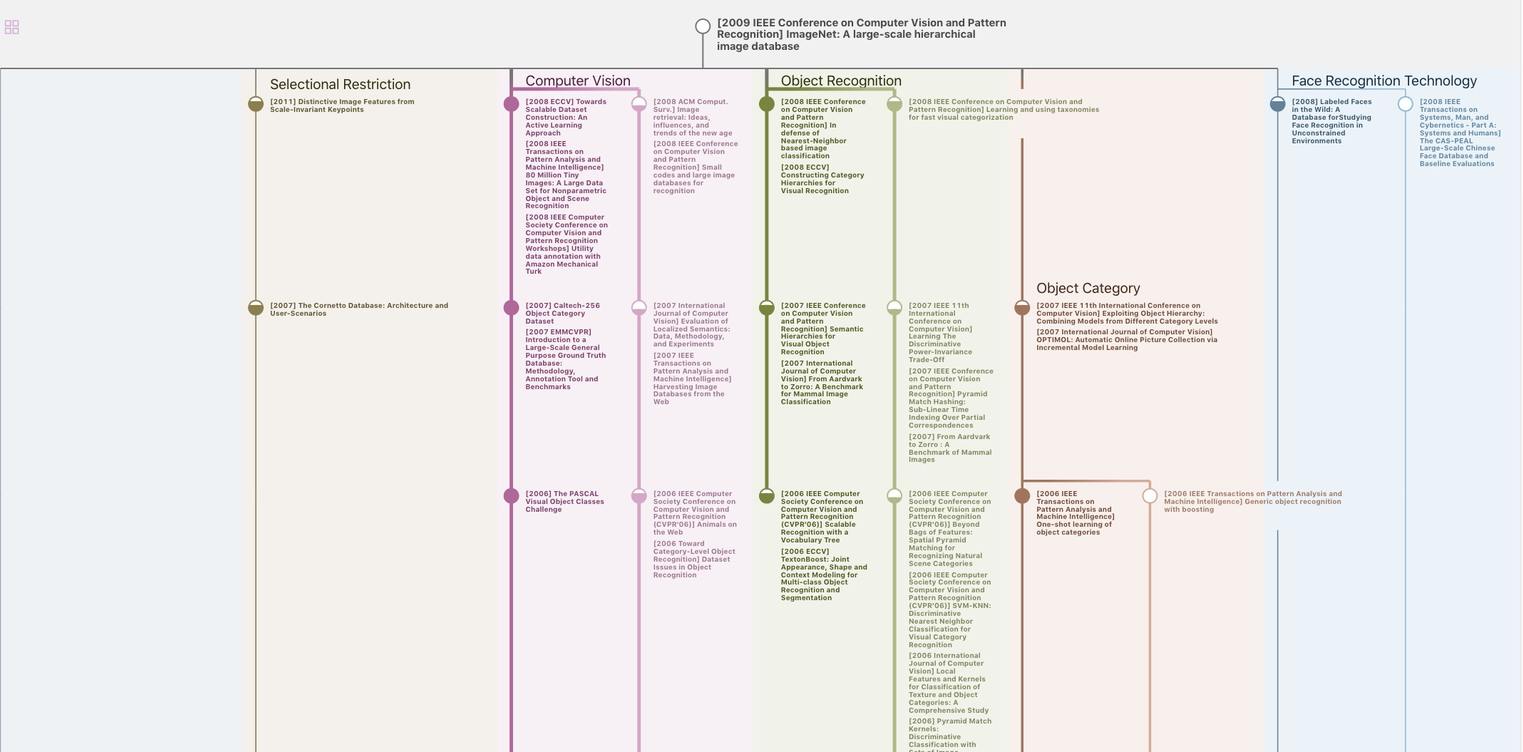
生成溯源树,研究论文发展脉络
Chat Paper
正在生成论文摘要