A domain knowledge-based interpretable deep learning system for improving clinical breast ultrasound diagnosis
COMMUNICATIONS MEDICINE(2024)
摘要
Background Though deep learning has consistently demonstrated advantages in the automatic interpretation of breast ultrasound images, its black-box nature hinders potential interactions with radiologists, posing obstacles for clinical deployment.Methods We proposed a domain knowledge-based interpretable deep learning system for improving breast cancer risk prediction via paired multimodal ultrasound images. The deep learning system was developed on 4320 multimodal breast ultrasound images of 1440 biopsy-confirmed lesions from 1348 prospectively enrolled patients across two hospitals between August 2019 and December 2022. The lesions were allocated to 70% training cohort, 10% validation cohort, and 20% test cohort based on case recruitment date.Results Here, we show that the interpretable deep learning system can predict breast cancer risk as accurately as experienced radiologists, with an area under the receiver operating characteristic curve of 0.902 (95% confidence interval = 0.882 - 0.921), sensitivity of 75.2%, and specificity of 91.8% on the test cohort. With the aid of the deep learning system, particularly its inherent explainable features, junior radiologists tend to achieve better clinical outcomes, while senior radiologists experience increased confidence levels. Multimodal ultrasound images augmented with domain knowledge-based reasoning cues enable an effective human-machine collaboration at a high level of prediction performance.Conclusions Such a clinically applicable deep learning system may be incorporated into future breast cancer screening and support assisted or second-read workflows. Breast cancer is one of the most common cancers, and finding it early can greatly improve patients' chances of survival and recovery. We create a tool based on artificial intelligence (AI)-whereby computer software learns to perform tasks that normally require human thinking-called MUP-Net. MUP-Net can analyze medical images to predict a patient's risk of having breast cancer. To make this AI tool usable in clinical practice, we enabled doctors to see the reasoning behind the AI's predictions by visualizing the key image features it analyzed. We showed that our AI tool not only makes doctors more confident in their diagnosis but also helps them make better decisions, especially for less experienced doctors. With further testing, our AI tool may help clinicians to diagnose breast cancer more accurately and quickly, potentially improving patient outcomes. Yan, Liang, Zhang et al. propose a domain knowledge-based interpretable deep learning system to improve breast cancer risk prediction from multimodal ultrasound images. Its inherent interpretability enables effective human-machine collaboration and thus may aid clinical decision-making.
更多查看译文
AI 理解论文
溯源树
样例
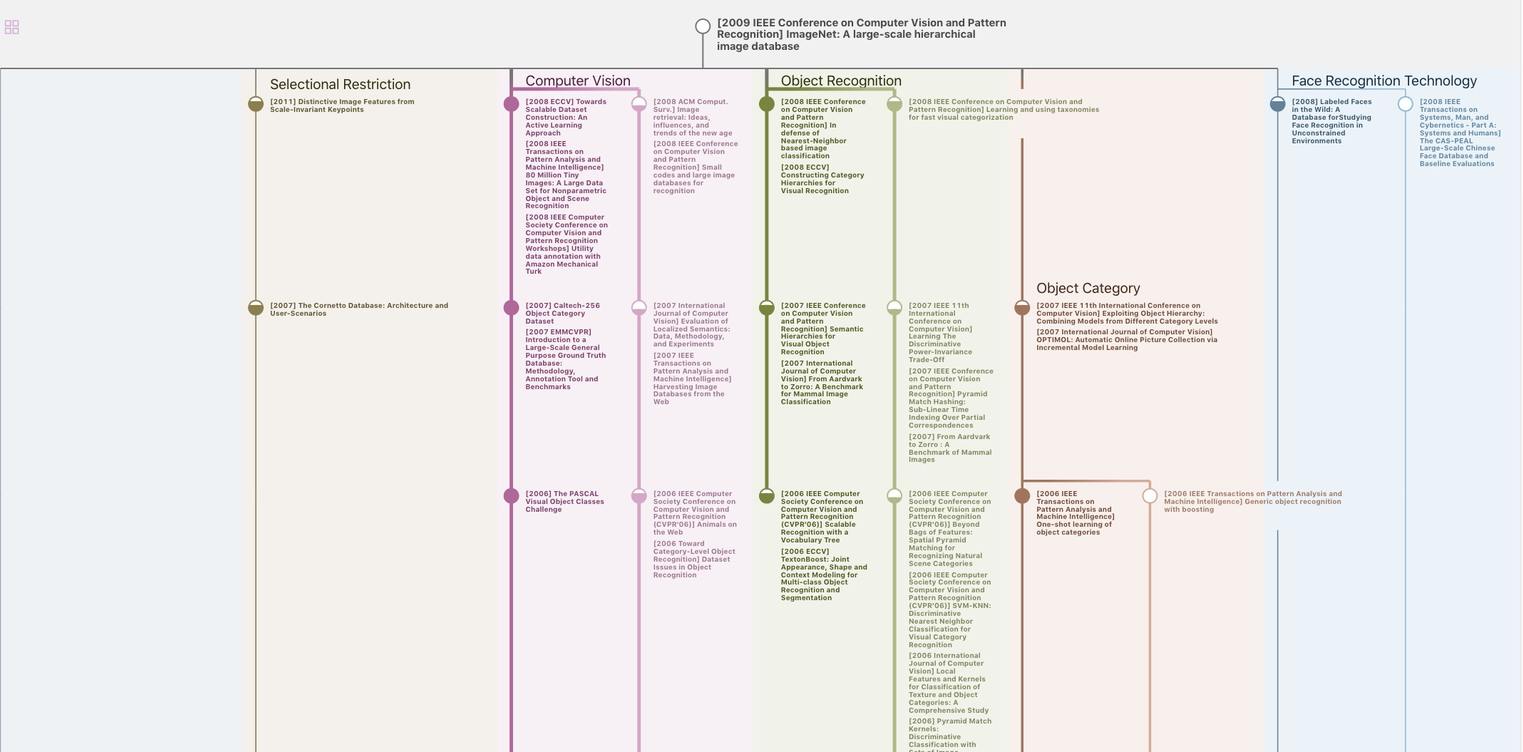
生成溯源树,研究论文发展脉络
Chat Paper
正在生成论文摘要