Abstract IA020: AI-powered Tool for Rapid & Reliable Bladder Cancer Screening and Surveillance: Multicenter Validation Efforts
Clinical Cancer Research(2024)
摘要
Abstract Bladder cancer is the most recurrent form of cancer, requiring frequent screening and surveillance, which contributes to its status as the costliest per capita. While urine cytology serves as a crucial adjunct to invasive cystoscopy, it is high volume, labor-intensive, and relatively inconsistent. Despite the implementation of The Paris System, which introduced updated quantitative guidelines, implementation of digital tools for urine cytology still trails the technological advancements seen in semi-automated Pap smear screenings. This lag can be attributed to the diverse cytomorphology of urine specimens and variation in specimen preparation and screening. Our team has developed AutoParis-X (APX), a state-of-the-art technology leveraging deep learning algorithms to extract objective (e.g., nucleus-to-cytoplasm ratio) and subjective (e.g., hyperchromasia) features from whole slide images (WSI). APX transforms these features into the Atypia Burden Score (ABS), offering a quantitative assessment of malignancy and recurrence risks on a scale from 0 to 1. Initially validated with 1300 samples (ThinPrep; Leica Aperio GT450) at Dartmouth Hitchcock, achieving an AUROC of 0.9, APX has evolved into APX-WEB, a user-friendly, web-based tool for cytopathologists. Notable challenges persist, including: 1) validating across nationally representative cohorts with varied specimen preparations and imaging, 2) addressing the intricacies of z-stacking for imaging non-monolayer preparations, and 3) integration of genomics to heighten the sensitivity of imaging assays. This study aimed to establish a performance baseline for APX's adaptability to these challenges without algorithmic adjustments. APX was applied across three distinct cohorts: 1) a preliminary national validation on 500 ThinPrep WSI in collaboration with Hologic; 2) assessment on non-monolayer specimen preparations (e.g., SurePath), using z-stacking, conducted with Johns Hopkins (n=100 WSI); and 3) a pilot study at Dartmouth (n=19) for integrating APX's imaging features with whole exomic sequencing (WES). Preliminary findings indicate: 1) Promising performance on a held-out Hologic national cohort (sensitivity=0.87, specificity=0.73; original APX study: sensitivity=0.88, specificity=0.83). 2) In SurePath z-stack WSI, the number of detected urothelial cells per cluster decreased with distance from the focus plane (rho =-0.13, p<0.001). 3) Of eight cases deemed negative or atypical by cytology, three exhibited TERT mutations, suggesting subclinical recurrence, in contrast to the consistent findings in all nine suspicious or positive cases. This highlights the potential of integrating genomics with image-based classifiers to enhance diagnostic precision. The findings underscore the need for future multicenter work aimed at refining machine learning models to achieve enhanced predictive performance across various operational parameters and settings, facilitating their implementation. Citation Format: Joshua Levy, Keluo Yao, Jonathan Marotti, Darcy Kerr, Edward Gutmann, Sam Harvey, Yoseph Sayegh, Chris VandenBussche, Michael Quick, Peter Costa, Lauren Wainman, Donald Green, Parth Shah, Xiaoying Liu, Louis Vaickus. AI-powered tool for rapid & reliable bladder cancer screening and surveillance: multicenter validation efforts [abstract]. In: Proceedings of the AACR Special Conference on Bladder Cancer: Transforming the Field; 2024 May 17-20; Charlotte, NC. Philadelphia (PA): AACR; Clin Cancer Res 2024;30(10_Suppl):Abstract nr IA020.
更多查看译文
AI 理解论文
溯源树
样例
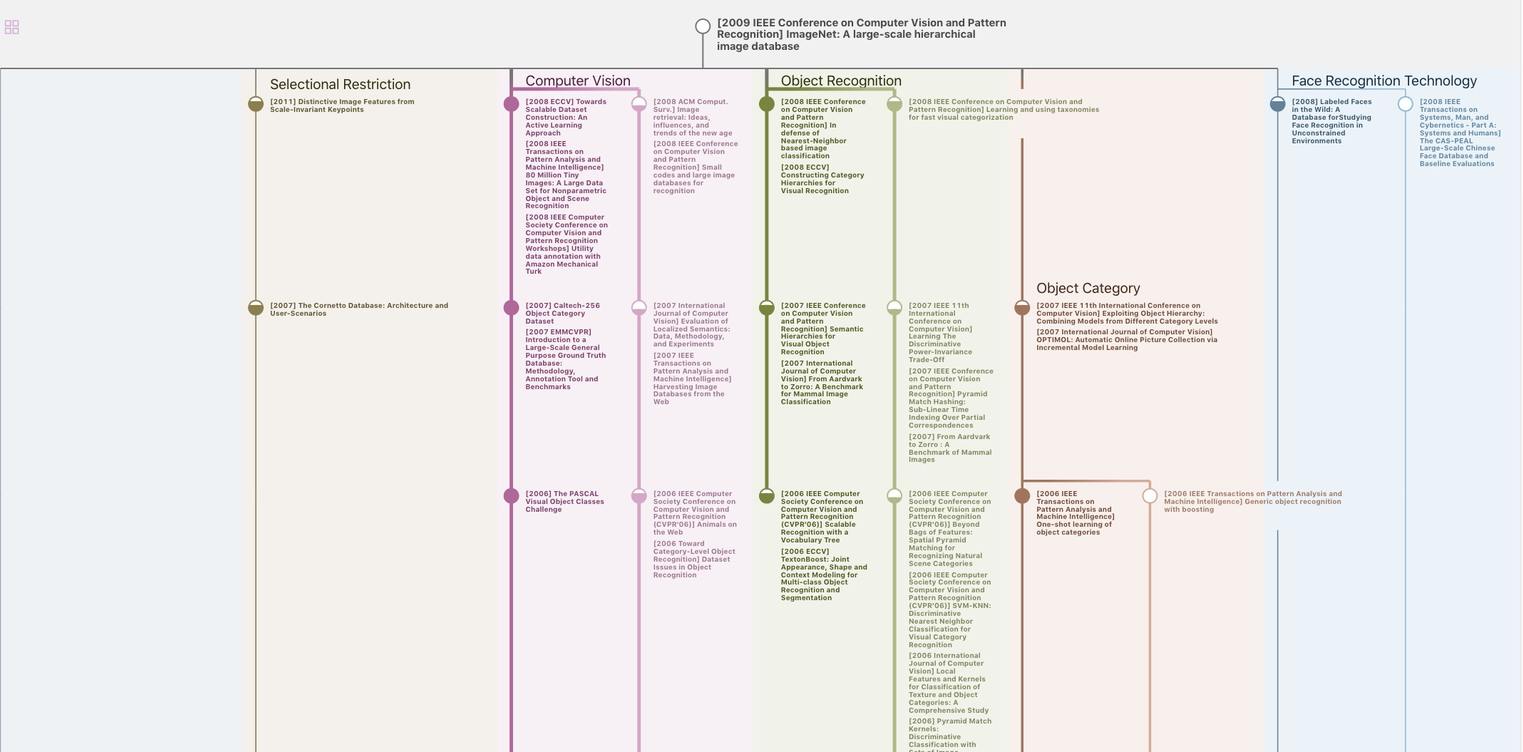
生成溯源树,研究论文发展脉络
Chat Paper
正在生成论文摘要