Convolutional neural network accelerated normal vector method for free-from meta-optics inverse design based on Fourier modal methods
crossref(2024)
摘要
Conventional Fourier modal methods (FMMs) remain computationally intensive for simulating and inversely designing free-form meta-atoms. This work introduces a convolutional neural network (CNN)-based method for generating normal vector (NV) fields to improve FMMs’ convergence. Our proposed models predict NV fields from free-form shapes with high accuracy and reduced computational demand. Theoretical analysis and experimental validation using the rigorous diffraction interface theory (R-DIT) demonstrate equivalent accuracy with significant speed improvement, particularly up to 3x speedup on GPU platforms, utilizing only minimal convolutional layers. This integration of machine learning with traditional simulation techniques illustrates the potential for pioneering advanced tools in photonics de
更多查看译文
AI 理解论文
溯源树
样例
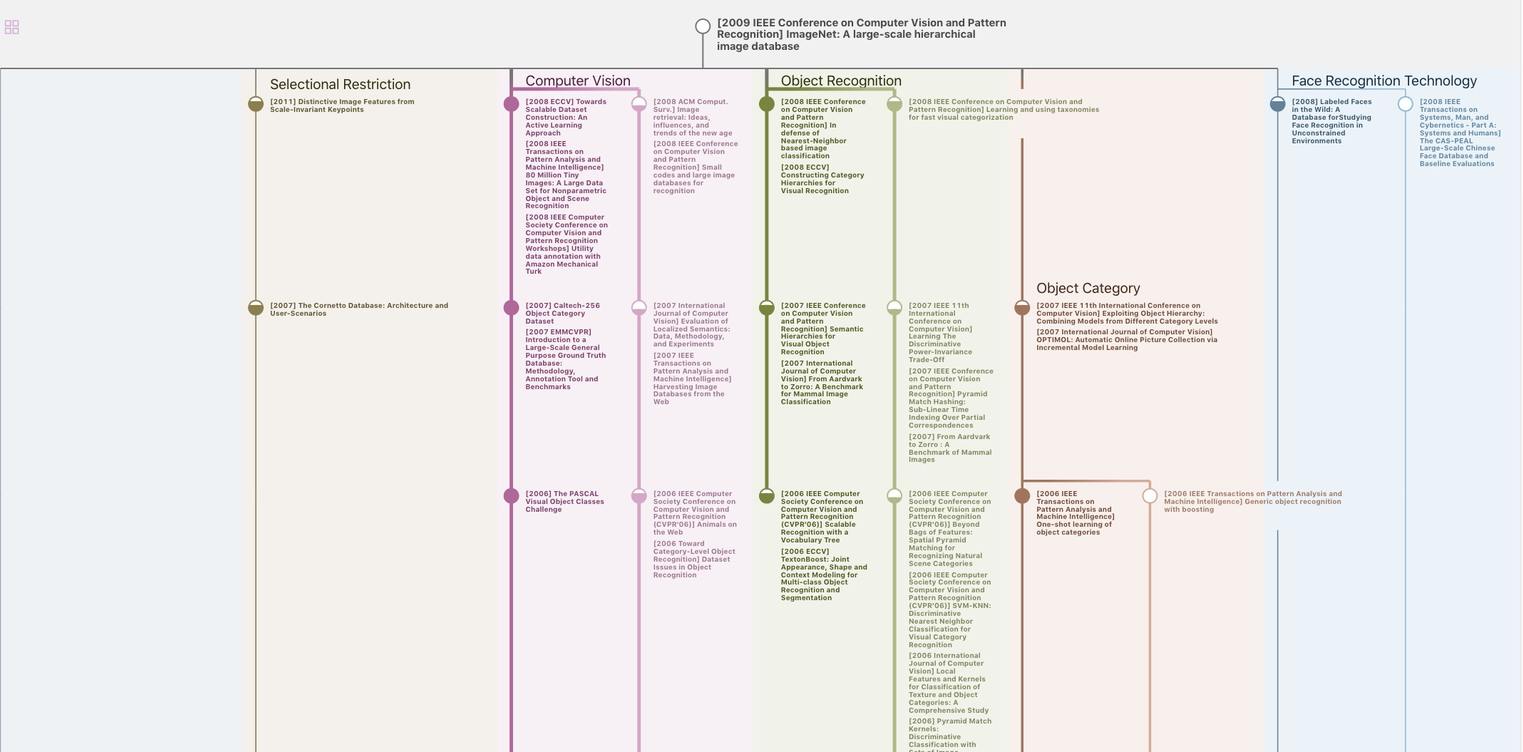
生成溯源树,研究论文发展脉络
Chat Paper
正在生成论文摘要